WIT by Google AI
WIT by Google AI is a vast multilingual dataset of 37.6M image-text pairs from Wikipedia, enhancing multimodal machine learning research.
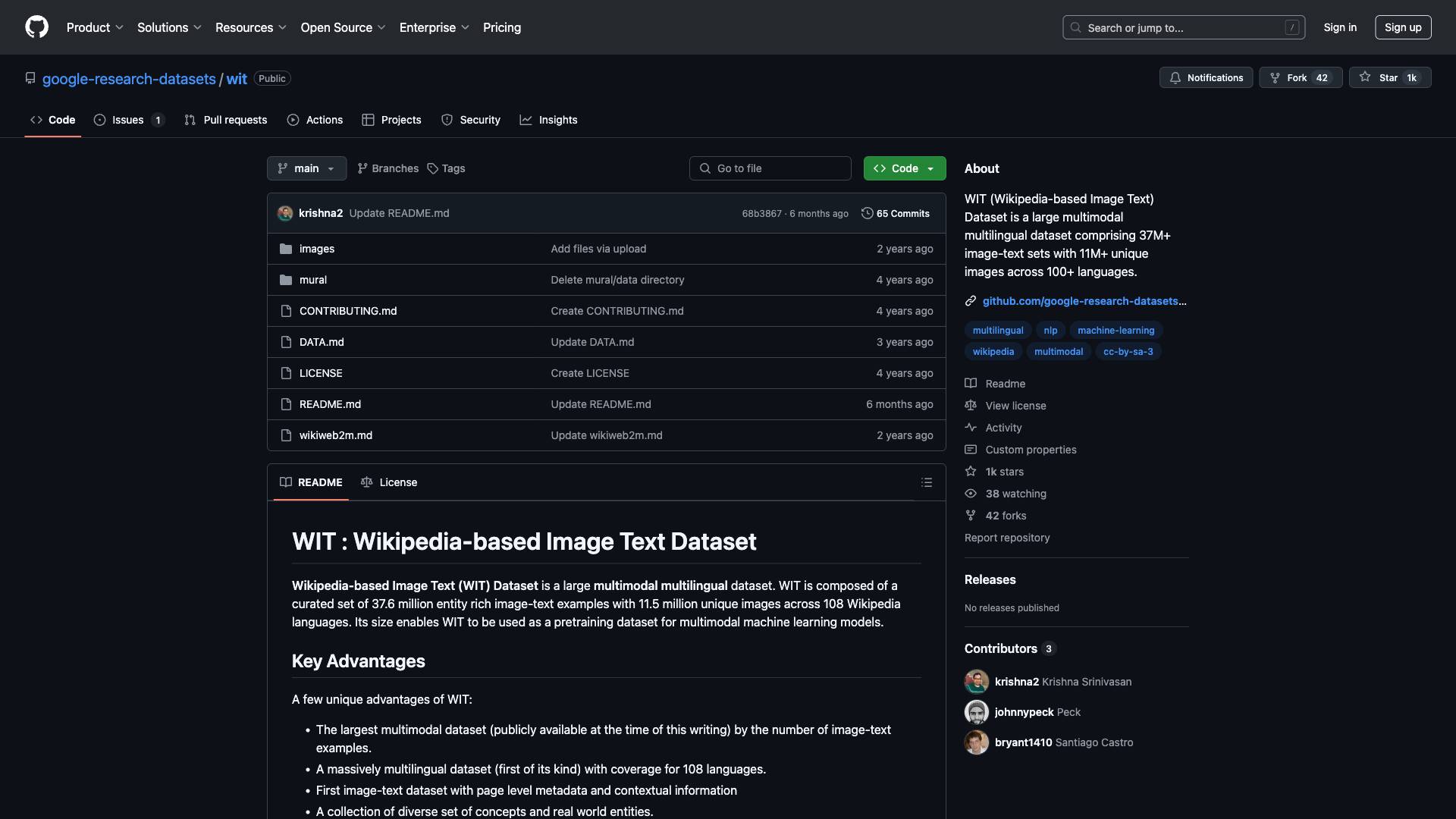
Tags
Useful for
- 1.What is WIT by Google AI?
- 1.1.Features
- 1.2.Use Cases
- 1.3.Pricing
- 1.4.Comparison with Other Tools
- 1.5.FAQ
- 1.5.1.What types of machine learning tasks can be performed using WIT?
- 1.5.2.How can I access the WIT dataset?
- 1.5.3.Is WIT suitable for academic research?
- 1.5.4.Can I use WIT for commercial purposes?
- 1.5.5.How does WIT compare to other multimodal datasets?
- 1.5.6.How can I contribute to the WIT project?
- 1.5.7.Who can I contact for more information about WIT?
What is WIT by Google AI?
WIT, or the Wikipedia-based Image Text Dataset, is an innovative tool developed by Google AI that serves as a large multimodal multilingual dataset. It comprises a curated collection of over 37.6 million image-text examples, featuring 11.5 million unique images across 108 languages sourced from Wikipedia. This dataset is designed to enhance the capabilities of multimodal machine learning models by providing a rich resource for training and evaluation.
The primary motivation behind the creation of WIT is to address the challenges faced by existing datasets, particularly in the realm of multilingual and multimodal learning. By leveraging images as a language-agnostic medium, WIT aims to improve the understanding of textual information across various languages, thus facilitating better performance in machine learning tasks.
Features
WIT boasts several standout features that make it a valuable resource for researchers and developers in the fields of machine learning and natural language processing:
-
Large Scale: WIT is the largest publicly available multimodal dataset, with 37.6 million image-text examples. This extensive size allows for comprehensive training and evaluation of machine learning models.
-
Multilingual Coverage: The dataset supports 108 languages, making it the first of its kind to offer such extensive multilingual coverage. This feature is crucial for advancing research in multilingual multimodal learning.
-
Diverse Content: WIT encompasses a wide range of concepts and real-world entities, providing a rich and diverse set of image-text pairs. This diversity is vital for training models that can generalize across different contexts.
-
Page Level Metadata: Unlike other datasets, WIT includes page-level metadata and contextual information, which adds depth to the image-text examples and aids in better understanding and modeling.
-
Rigorous Filtering: The dataset is created through a meticulous process of extracting and filtering high-quality image-text pairs from Wikipedia articles, ensuring that the data is clean and reliable.
-
Challenging Test Sets: WIT provides challenging real-world test sets that can be used to evaluate the performance of multimodal models, pushing the boundaries of current research.
-
Community Engagement: WIT encourages collaboration and engagement within the research community, allowing users to share their findings and projects that utilize the dataset.
Use Cases
WIT can be applied in various domains and use cases, enhancing the capabilities of machine learning models and facilitating research in multimodal learning:
-
Multimodal Machine Learning: Researchers can utilize WIT to train models that learn the relationships between images and text, improving performance in tasks such as image captioning, visual question answering, and image-text retrieval.
-
Multilingual Natural Language Processing: The dataset's extensive language coverage enables the development of multilingual NLP models, allowing for better understanding and processing of text in various languages.
-
Image Retrieval Systems: WIT can be employed to build robust image retrieval systems that leverage both visual and textual information, enhancing the accuracy and relevance of search results.
-
Content Creation and Enhancement: Developers can use WIT to create tools that assist in content generation, such as automatically generating descriptions for images or suggesting relevant images for given text.
-
Academic Research: Scholars and researchers can leverage WIT for academic purposes, conducting studies on multimodal learning, language representation, and the intersection of vision and language.
-
Competitions and Challenges: WIT has been featured in various competitions, such as the WIT Image-Text Competition on Kaggle, providing a platform for researchers to showcase their models and innovations.
Pricing
WIT is made available for free under the Creative Commons Attribution-ShareAlike 3.0 Unported license, making it accessible to researchers, developers, and enthusiasts. This open-access model encourages widespread use and collaboration within the community, enabling users to benefit from the dataset without financial barriers.
Comparison with Other Tools
When comparing WIT to other multimodal datasets, several unique aspects set it apart:
-
Size and Scale: WIT is the largest multimodal dataset available, surpassing many other datasets in terms of the number of image-text pairs. This extensive size allows for more comprehensive training and evaluation of machine learning models.
-
Multilingual Focus: While many datasets primarily focus on English, WIT's coverage of 108 languages addresses a significant gap in the research community, enabling the development of multilingual models that can process text in various languages.
-
Quality Control: WIT's rigorous filtering process ensures that only high-quality image-text pairs are included, which is not always the case with other datasets. This focus on quality enhances the reliability of the dataset for research and development.
-
Rich Metadata: The inclusion of page-level metadata and contextual information is a distinguishing feature of WIT, providing additional insights that can aid in modeling and understanding the relationships between images and text.
-
Community Engagement: WIT actively encourages collaboration and engagement within the research community, fostering a culture of sharing and innovation that may not be as prevalent in other datasets.
FAQ
What types of machine learning tasks can be performed using WIT?
WIT can be utilized for a variety of machine learning tasks, including image captioning, visual question answering, image-text retrieval, and more. Its diverse and extensive dataset allows for the training of models that can effectively learn the relationships between images and text.
How can I access the WIT dataset?
The WIT dataset is available for free under the Creative Commons Attribution-ShareAlike 3.0 Unported license. Interested users can download the dataset from the official repository.
Is WIT suitable for academic research?
Yes, WIT is an excellent resource for academic research. Its large scale, multilingual coverage, and high-quality data make it a valuable tool for studies in multimodal learning, natural language processing, and the intersection of vision and language.
Can I use WIT for commercial purposes?
While WIT is available under an open-access license, users should review the specific terms of the Creative Commons Attribution-ShareAlike 3.0 Unported license to understand the conditions for commercial use.
How does WIT compare to other multimodal datasets?
WIT stands out due to its size, multilingual focus, quality control measures, and inclusion of rich metadata. These features make it a unique and powerful resource for researchers and developers working in the fields of multimodal learning and natural language processing.
How can I contribute to the WIT project?
WIT encourages community engagement and collaboration. Users are invited to share their findings, research projects, or blog posts related to WIT, fostering a culture of innovation and knowledge sharing.
Who can I contact for more information about WIT?
For any questions or inquiries regarding the WIT dataset, users can reach out to the designated contact email provided in the dataset's documentation. Additionally, users can contact the first author, Krishna, through their personal page for more specific queries.
In conclusion, WIT by Google AI represents a significant advancement in the field of multimodal multilingual datasets. Its extensive size, multilingual coverage, and commitment to quality make it an invaluable resource for researchers and developers alike. By facilitating better understanding and modeling of the relationships between images and text, WIT has the potential to drive innovation and progress in machine learning and natural language processing.
Ready to try it out?
Go to WIT by Google AI