Synthetic Data Hub
Synthetic Data Hub offers curated, anonymized datasets for AI and machine learning, enhancing data privacy and model training through robust APIs.
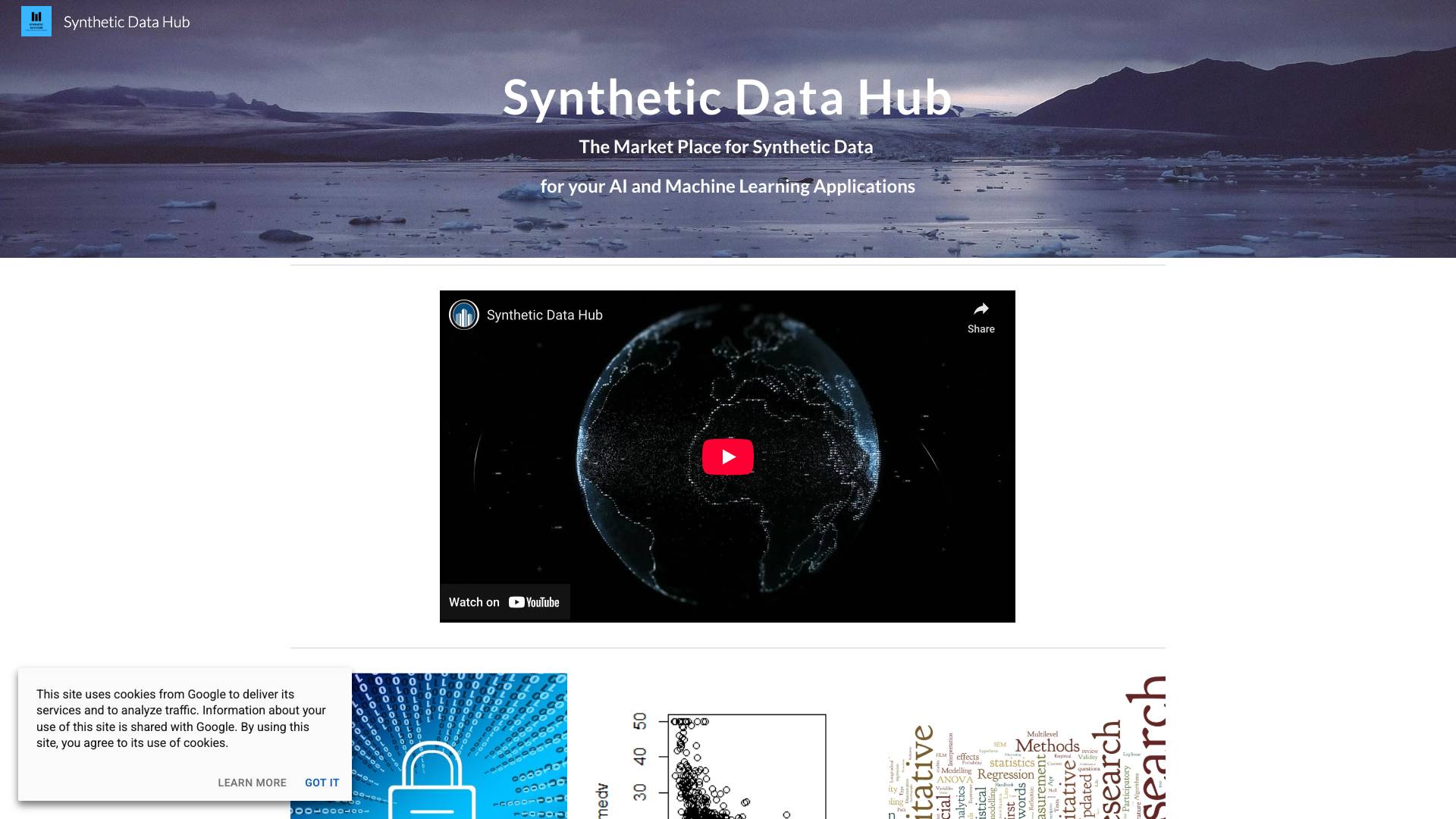
Tags
Useful for
- 1.What is Synthetic Data Hub?
- 1.1.Features
- 1.1.1.1. Anonymity and Privacy
- 1.1.2.2. Data Augmentation
- 1.1.3.3. Robust and Tested APIs
- 1.1.4.4. Data Spec Sheets
- 1.1.5.5. Powered by QuSandbox
- 1.1.6.6. Subscription for Updates
- 1.2.Use Cases
- 1.2.1.1. Healthcare
- 1.2.2.2. Finance
- 1.2.3.3. Autonomous Vehicles
- 1.2.4.4. Retail and E-commerce
- 1.2.5.5. Telecommunications
- 1.3.Pricing
- 1.3.1.1. Pay-As-You-Go
- 1.3.2.2. Subscription Plans
- 1.3.3.3. Free Trials
- 1.4.Comparison with Other Tools
- 1.4.1.1. Quality of Datasets
- 1.4.2.2. Anonymization
- 1.4.3.3. Ease of Integration
- 1.4.4.4. Use Case Versatility
- 1.5.FAQ
- 1.5.1.1. What is synthetic data?
- 1.5.2.2. How does Synthetic Data Hub ensure data quality?
- 1.5.3.3. Can I integrate synthetic data into my existing machine learning workflows?
- 1.5.4.4. Is synthetic data suitable for all types of machine learning applications?
- 1.5.5.5. How do I get started with Synthetic Data Hub?
What is Synthetic Data Hub?
Synthetic Data Hub is an innovative marketplace designed to provide high-quality synthetic datasets for artificial intelligence (AI) and machine learning (ML) applications. As organizations increasingly rely on data to train their models, the need for diverse, anonymized, and comprehensive datasets has grown significantly. Synthetic Data Hub addresses this demand by offering a platform where users can access, purchase, and utilize synthetic data that enhances their machine learning efforts while ensuring privacy and compliance with data protection regulations.
Features
Synthetic Data Hub boasts a variety of features that make it an essential tool for AI and ML practitioners. Here are some of the key features:
1. Anonymity and Privacy
- Anonymized Datasets: The platform provides datasets that have been carefully anonymized to ensure that they do not contain personally identifiable information (PII). This feature is crucial for organizations that need to comply with data protection laws, such as GDPR and HIPAA.
- Data Compliance: By leveraging anonymized data, users can train their models without the risk of exposing sensitive information, thus maintaining compliance with various regulations.
2. Data Augmentation
- Supplement Traditional Datasets: Synthetic Data Hub allows users to enhance their existing datasets by providing new and varied synthetic data. This helps in overcoming common issues such as data scarcity and bias.
- Increased Model Robustness: By using diverse datasets, machine learning models can achieve better generalization, leading to improved performance in real-world applications.
3. Robust and Tested APIs
- Curated and Tested Datasets: All datasets available on Synthetic Data Hub are curated and tested using QuSandbox, ensuring that they meet high-quality standards and are ready for immediate use.
- API Integration: The platform provides robust APIs that allow for seamless integration with existing machine learning workflows, making it easy for users to incorporate synthetic data into their projects.
4. Data Spec Sheets
- Detailed Specifications: Each dataset comes with a comprehensive spec sheet that outlines its characteristics, including data types, dimensions, and potential use cases. This information helps users make informed decisions when selecting datasets for their projects.
5. Powered by QuSandbox
- Advanced Testing Framework: QuSandbox is a sophisticated testing framework that powers the Synthetic Data Hub, ensuring that all datasets are reliable and ready for deployment in AI and ML applications.
6. Subscription for Updates
- Stay Informed: Users can subscribe to receive updates about new datasets, features, and enhancements, ensuring they are always aware of the latest offerings from Synthetic Data Hub.
Use Cases
The versatility of Synthetic Data Hub makes it suitable for a wide range of applications across various industries. Here are some notable use cases:
1. Healthcare
- Patient Data Simulation: Healthcare organizations can use synthetic data to simulate patient records for research and training purposes without compromising patient privacy.
- Clinical Trials: Synthetic datasets can assist in designing and testing clinical trials by providing diverse patient scenarios that reflect real-world complexities.
2. Finance
- Fraud Detection: Financial institutions can utilize synthetic datasets to train machine learning models that identify fraudulent transactions, helping to enhance security measures.
- Risk Assessment: Synthetic data can be used to model various financial scenarios, allowing institutions to better assess risk and make informed decisions.
3. Autonomous Vehicles
- Simulation of Driving Scenarios: Companies developing autonomous vehicles can leverage synthetic data to create diverse driving scenarios for training their AI systems, ensuring they can handle a wide range of conditions and situations.
- Safety Testing: Synthetic datasets can be used to simulate rare but critical driving situations, allowing for thorough testing of safety features.
4. Retail and E-commerce
- Customer Behavior Analysis: Retailers can use synthetic data to analyze customer behavior patterns, helping them to optimize marketing strategies and improve customer experiences.
- Inventory Management: Synthetic datasets can aid in forecasting demand and managing inventory by simulating various market conditions.
5. Telecommunications
- Network Optimization: Telecommunications companies can utilize synthetic data to model network traffic and optimize performance, enhancing service quality for customers.
- Churn Prediction: By analyzing synthetic datasets, telecom providers can identify patterns that lead to customer churn and implement strategies to retain subscribers.
Pricing
While specific pricing details may vary, Synthetic Data Hub typically offers several pricing tiers to accommodate different user needs. Here are some common pricing structures:
1. Pay-As-You-Go
- Users can purchase individual datasets on a pay-per-use basis, allowing for flexibility and minimizing upfront costs.
2. Subscription Plans
- Monthly/Annual Subscriptions: Users may opt for subscription plans that provide access to a certain number of datasets per month or year, often at a discounted rate compared to individual purchases.
- Enterprise Solutions: Organizations with larger data needs can inquire about customized enterprise solutions that cater to their specific requirements.
3. Free Trials
- Some platforms may offer free trials or limited access to datasets for new users, allowing them to explore the features and benefits of Synthetic Data Hub before committing to a purchase.
Comparison with Other Tools
When evaluating Synthetic Data Hub, it's essential to compare it with other synthetic data generation tools available in the market. Here are some key differentiators:
1. Quality of Datasets
- Synthetic Data Hub: Datasets are curated and tested using the QuSandbox framework, ensuring high quality and reliability.
- Other Tools: Some alternatives may not have the same level of rigorous testing, potentially leading to lower-quality datasets.
2. Anonymization
- Synthetic Data Hub: Focuses on providing fully anonymized datasets that comply with data protection regulations, making it suitable for sensitive applications.
- Other Tools: Not all synthetic data tools prioritize anonymization, which can pose risks for organizations handling sensitive information.
3. Ease of Integration
- Synthetic Data Hub: Offers robust APIs that facilitate easy integration into existing workflows, streamlining the data incorporation process.
- Other Tools: Some alternatives may lack user-friendly integration options, making it challenging for users to implement synthetic data into their projects.
4. Use Case Versatility
- Synthetic Data Hub: Supports a wide array of use cases across different industries, making it a versatile option for various applications.
- Other Tools: Some tools may focus on niche applications, limiting their usability in broader contexts.
FAQ
1. What is synthetic data?
Synthetic data is artificially generated data that mimics the characteristics of real-world data without containing any actual personal or sensitive information. It is used to train machine learning models while ensuring privacy and compliance with data protection regulations.
2. How does Synthetic Data Hub ensure data quality?
Synthetic Data Hub ensures data quality by curating and testing all datasets using the QuSandbox framework. This rigorous testing process guarantees that users receive high-quality, reliable datasets ready for deployment.
3. Can I integrate synthetic data into my existing machine learning workflows?
Yes, Synthetic Data Hub provides robust APIs that allow for seamless integration of synthetic data into existing machine learning workflows, making it easy to incorporate new datasets into your projects.
4. Is synthetic data suitable for all types of machine learning applications?
While synthetic data is versatile and can be used in various applications, its effectiveness may depend on the specific use case and the quality of the generated data. It is essential to evaluate whether synthetic data meets the requirements of your particular application.
5. How do I get started with Synthetic Data Hub?
To get started, you can visit the Synthetic Data Hub platform, explore available datasets, and choose a pricing plan that suits your needs. You may also subscribe for updates to stay informed about new datasets and features.
In conclusion, Synthetic Data Hub is a powerful tool designed to meet the growing demand for high-quality synthetic datasets in AI and machine learning applications. Its focus on anonymity, data augmentation, and robust APIs makes it an invaluable resource for organizations looking to enhance their machine learning efforts while ensuring compliance with data protection regulations.
Ready to try it out?
Go to Synthetic Data Hub