
Ray
Ray is a powerful AI compute engine that seamlessly scales and optimizes any AI, ML, or Gen AI workload across diverse infrastructures.
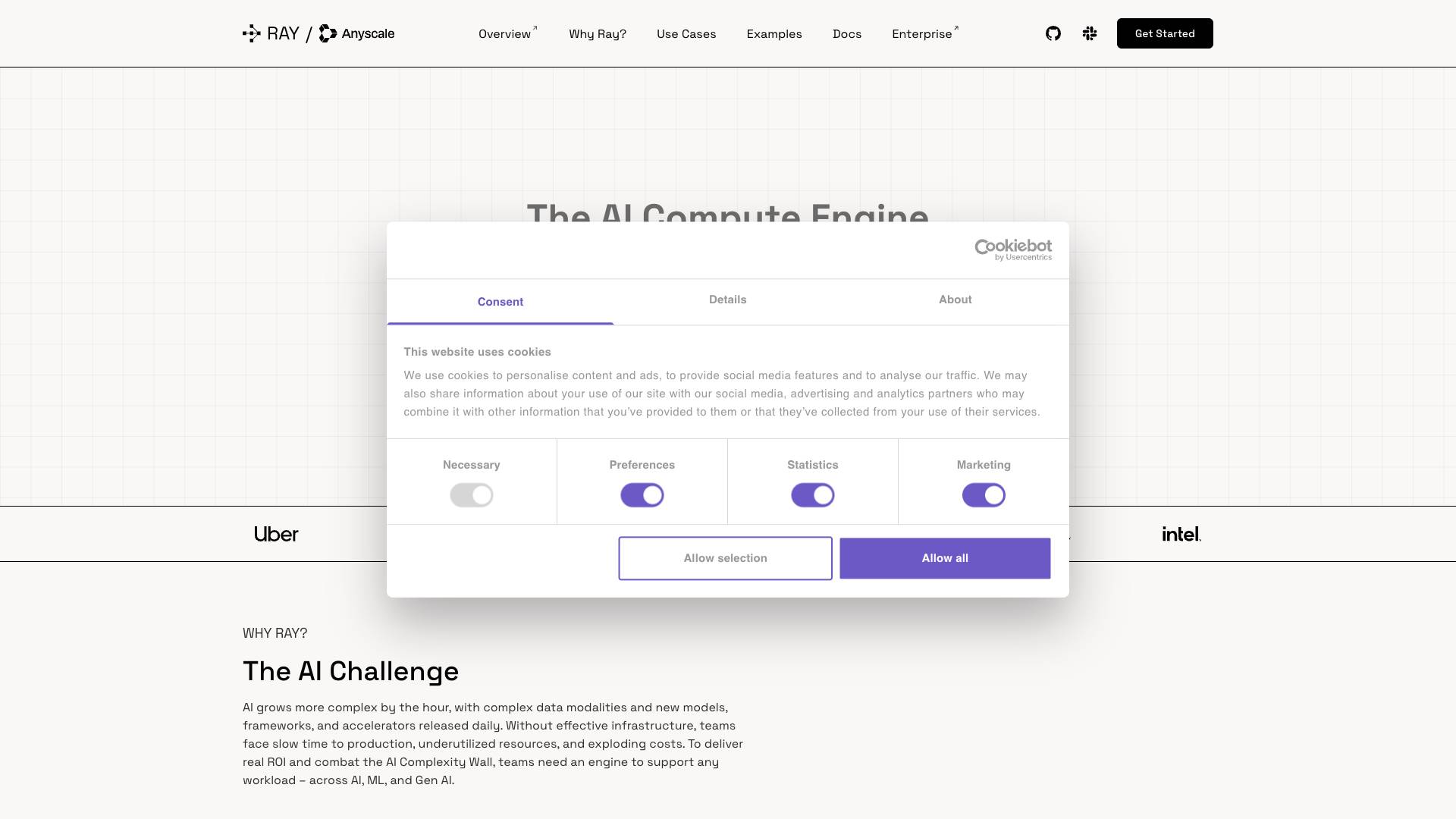
Tags
Useful for
- 1.What is Ray?
- 2.Features
- 2.1.1. Support for Any AI or ML Workload
- 2.2.2. Multi-Modal Data Processing
- 2.3.3. Heterogeneous Compute
- 2.4.4. Seamless Scaling
- 2.5.5. Parallel Python Code Execution
- 2.6.6. Model Training and Serving
- 2.7.7. Batch Inference
- 2.8.8. Reinforcement Learning
- 2.9.9. Generative AI Support
- 2.10.10. Community and Ecosystem
- 3.Use Cases
- 3.1.1. Model Training
- 3.2.2. Data Processing
- 3.3.3. Real-Time Inference
- 3.4.4. Batch Inference Workflows
- 3.5.5. Reinforcement Learning Applications
- 3.6.6. Generative AI Workflows
- 3.7.7. Simulation and Backtesting
- 4.Pricing
- 5.Comparison with Other Tools
- 5.1.1. Ray vs. Apache Spark
- 5.2.2. Ray vs. TensorFlow
- 5.3.3. Ray vs. Dask
- 6.FAQ
- 6.1.Q: Is Ray suitable for small-scale projects?
- 6.2.Q: Can I use Ray with my existing AI frameworks?
- 6.3.Q: Is Ray open-source?
- 6.4.Q: What kind of support is available for Ray users?
- 6.5.Q: How does Ray handle resource management?
- 6.6.Q: What types of data can Ray process?
- 6.7.Q: Can I run reinforcement learning workflows with Ray?
What is Ray?
Ray is an advanced AI compute engine designed to address the complexities of modern artificial intelligence (AI) workloads. It is particularly adept at orchestrating distributed workloads across a variety of accelerators, such as GPUs and CPUs, making it a versatile tool for developers aiming to streamline their AI and machine learning (ML) processes. Ray is Python-native, which means it is built specifically for Python developers, allowing them to scale and distribute their applications with ease.
Ray is an open-source project backed by a vibrant community, continuously evolving to meet the demands of the AI landscape. Its capabilities make it suitable for a range of applications, from model training to serving and inference, all while optimizing resource utilization and reducing operational costs.
Features
Ray comes packed with a variety of features that make it a powerful tool for AI development:
1. Support for Any AI or ML Workload
Ray can handle a wide array of workloads, including traditional machine learning, deep learning, and generative AI. This versatility allows teams to use a single platform for multiple applications.
2. Multi-Modal Data Processing
Ray can process both structured and unstructured data, including images, videos, and audio. This feature is essential for modern AI applications that require diverse data types.
3. Heterogeneous Compute
Ray allows for the use of different types of hardware, including various models of GPUs and CPUs. This capability enables fine-grained scaling and maximizes resource utilization.
4. Seamless Scaling
Ray can scale from a single laptop to thousands of GPUs, making it suitable for both small-scale projects and large enterprise applications.
5. Parallel Python Code Execution
Ray is designed for Python developers, allowing them to easily scale and distribute any Python code. This feature is particularly useful for simulation and backtesting applications.
6. Model Training and Serving
Ray simplifies the process of training and serving models. With just one line of code, users can initiate distributed training for various model types, including foundation models and traditional ML models.
7. Batch Inference
Ray streamlines offline batch inference workflows by leveraging heterogeneous compute resources, which helps increase utilization and decrease costs.
8. Reinforcement Learning
Ray RLlib supports production-level reinforcement learning workflows and provides a unified API for various industry applications, making it easier to implement complex RL algorithms.
9. Generative AI Support
Ray facilitates the creation of end-to-end generative AI workflows, supporting multimodal models and retrieval-augmented generation (RAG) applications.
10. Community and Ecosystem
Ray is backed by a growing open-source community, which contributes to its continuous improvement and evolution. The ecosystem includes tools for deploying Ray clusters, debugging, and optimizing applications.
Use Cases
Ray is versatile and can be applied in various domains and industries. Here are some notable use cases:
1. Model Training
Organizations can use Ray to run distributed training for a wide range of models, from deep learning networks to traditional machine learning algorithms. This capability is particularly beneficial for teams working with large datasets or complex model architectures.
2. Data Processing
Ray's ability to handle both structured and unstructured data makes it ideal for preprocessing data before training models. This includes tasks like cleaning, transforming, and enriching datasets.
3. Real-Time Inference
Ray can be used to deploy machine learning models for real-time inference. With its efficient resource management, businesses can serve models to end-users with minimal latency.
4. Batch Inference Workflows
Organizations can leverage Ray for offline batch inference, where they need to process large volumes of data in a cost-effective manner. The ability to utilize both CPUs and GPUs in the same pipeline enhances efficiency.
5. Reinforcement Learning Applications
Ray RLlib can be employed in industries like gaming, robotics, and finance, where reinforcement learning is essential for developing adaptive systems that learn from their environment.
6. Generative AI Workflows
Ray supports the development of generative AI applications, such as chatbots and content generation tools, by facilitating the training and deployment of multimodal models.
7. Simulation and Backtesting
Ray can be used for financial simulations and backtesting trading algorithms, allowing developers to test their strategies against historical data efficiently.
Pricing
Ray is an open-source tool, which means that it is free to use. However, organizations that require additional support, training, or managed services may consider utilizing Anyscale, the fully managed AI platform built specifically for Ray. Anyscale provides enterprise governance, advanced developer tooling, and expert support for teams looking to maximize their investment in Ray.
While the core functionality of Ray is free, the costs associated with deploying Ray on cloud infrastructure, maintaining hardware, and utilizing Anyscale's managed services will vary based on the specific needs of the organization.
Comparison with Other Tools
Ray stands out in the crowded landscape of AI and ML tools due to its unique features and capabilities. Here’s how it compares to some other popular tools:
1. Ray vs. Apache Spark
- Scalability: Ray can scale efficiently from a single laptop to thousands of GPUs, while Spark is primarily designed for distributed computing on large clusters.
- Flexibility: Ray supports a wider variety of workloads, including reinforcement learning and generative AI, whereas Spark is more focused on big data processing.
- Resource Utilization: Ray allows for heterogeneous compute, enabling users to mix different types of hardware in a single pipeline, which can lead to better resource utilization compared to Spark.
2. Ray vs. TensorFlow
- Ease of Use: Ray is designed to be Python-native, making it easier for Python developers to scale their applications. TensorFlow can be more complex to set up for distributed training.
- Versatility: While TensorFlow excels in deep learning, Ray supports a broader range of AI and ML workloads, including reinforcement learning and batch inference.
- Community Support: Ray's open-source community is rapidly growing, providing a wealth of resources and contributions, similar to TensorFlow's established community.
3. Ray vs. Dask
- Performance: Ray is optimized for high-performance distributed computing, especially for AI workloads, while Dask focuses on parallel computing with a more general approach.
- Specialization: Ray is tailored for AI and ML tasks, whereas Dask is more of a general-purpose parallel computing library.
- Integration: Ray offers seamless integration with various AI frameworks, making it easier to deploy models across different environments.
FAQ
Q: Is Ray suitable for small-scale projects?
A: Yes, Ray can scale from a single laptop to large clusters, making it suitable for both small and large-scale projects.
Q: Can I use Ray with my existing AI frameworks?
A: Absolutely! Ray is compatible with various AI frameworks, allowing you to leverage its capabilities without having to change your existing setup.
Q: Is Ray open-source?
A: Yes, Ray is an open-source project, and it is backed by a growing community of contributors.
Q: What kind of support is available for Ray users?
A: While Ray itself is open-source, users can opt for managed services and expert support through Anyscale, the platform built specifically for Ray.
Q: How does Ray handle resource management?
A: Ray efficiently manages resources by allowing for fine-grained scaling and the ability to utilize heterogeneous compute resources, optimizing performance and cost.
Q: What types of data can Ray process?
A: Ray can process both structured and unstructured data, including images, videos, and audio, making it versatile for various AI applications.
Q: Can I run reinforcement learning workflows with Ray?
A: Yes, Ray RLlib supports production-level reinforcement learning workflows, making it suitable for applications in gaming, robotics, and finance.
In conclusion, Ray is a powerful and flexible AI compute engine that addresses the complexities of modern AI workloads. Its unique features, extensive use cases, and strong community support make it an ideal choice for organizations looking to optimize their AI development processes. Whether you are a developer looking to scale your applications or a business aiming to reduce operational costs, Ray offers the tools and capabilities you need to succeed in the fast-evolving world of AI.
Ready to try it out?
Go to Ray