Rasa NLU
Rasa NLU is an open-source tool that enables developers to build conversational AI by understanding user intents and extracting entities from text.
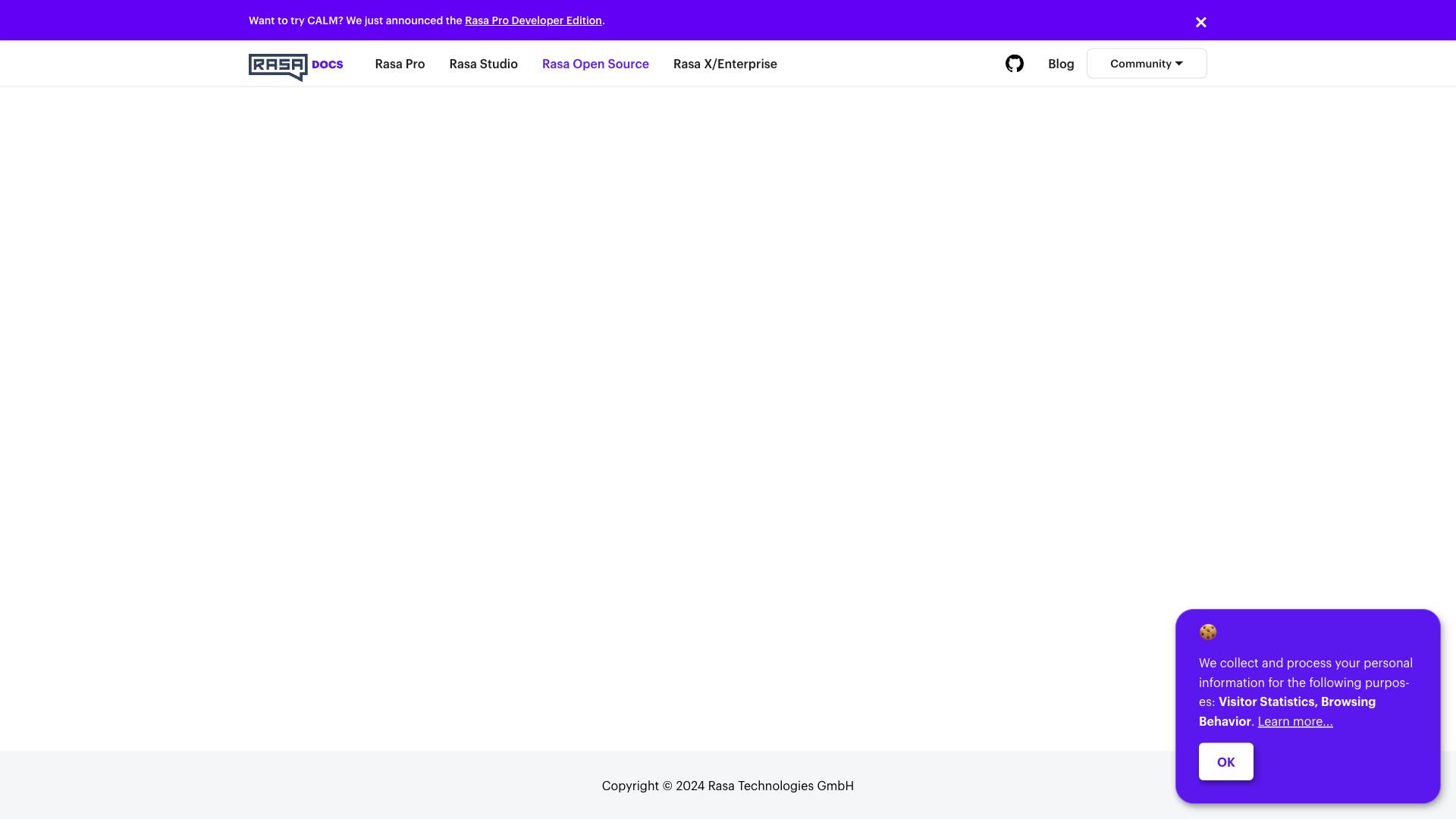
Tags
Useful for
- 1.Rasa NLU
- 1.1.What is Rasa NLU?
- 1.2.Features
- 1.2.1.1. Intent Recognition
- 1.2.2.2. Entity Extraction
- 1.2.3.3. Multi-language Support
- 1.2.4.4. Customizable Pipelines
- 1.2.5.5. Pre-trained Models
- 1.2.6.6. Training and Evaluation
- 1.2.7.7. Integration Capabilities
- 1.2.8.8. Community Support
- 1.2.9.9. Contextual Understanding
- 1.2.10.10. Open Source
- 1.3.Use Cases
- 1.3.1.1. Customer Support
- 1.3.2.2. E-commerce
- 1.3.3.3. Healthcare
- 1.3.4.4. Education
- 1.3.5.5. Travel and Hospitality
- 1.3.6.6. Entertainment
- 1.3.7.7. Internal Business Tools
- 1.4.Pricing
- 1.4.1.Enterprise Features (Optional)
- 1.5.Comparison with Other Tools
- 1.5.1.1. Open Source vs. Proprietary
- 1.5.2.2. Customization and Extensibility
- 1.5.3.3. Contextual Understanding
- 1.5.4.4. Community and Ecosystem
- 1.5.5.5. Language Support
- 1.5.6.6. Integration Capabilities
- 1.6.FAQ
- 1.6.1.Q1: Is Rasa NLU suitable for beginners?
- 1.6.2.Q2: Can I use Rasa NLU for commercial purposes?
- 1.6.3.Q3: How do I train a model in Rasa NLU?
- 1.6.4.Q4: What programming languages does Rasa NLU support?
- 1.6.5.Q5: Can Rasa NLU handle multiple intents in a single user input?
- 1.6.6.Q6: How does Rasa NLU handle entity recognition?
- 1.6.7.Q7: Is there a way to test my Rasa NLU model?
- 1.6.8.Q8: What are the system requirements for running Rasa NLU?
Rasa NLU
What is Rasa NLU?
Rasa NLU (Natural Language Understanding) is an open-source framework designed to facilitate the development of conversational AI applications. It provides developers with the tools needed to build chatbots and virtual assistants that can understand and respond to user inputs in a natural and meaningful way. Rasa NLU is part of the larger Rasa ecosystem, which also includes Rasa Core, a framework for managing dialogue and conversation flow.
Rasa NLU allows developers to create custom models that can interpret user intents, extract relevant entities, and handle complex conversational scenarios. Its flexibility and extensibility make it suitable for various applications, from simple FAQ bots to sophisticated customer support systems.
Features
Rasa NLU is packed with features that enhance its functionality and usability for developers:
1. Intent Recognition
Rasa NLU can accurately identify user intents based on the input text. This feature allows the system to determine what the user wants to achieve, whether it's asking a question, making a request, or initiating a command.
2. Entity Extraction
The framework can extract specific pieces of information from user inputs, known as entities. This is crucial for understanding user requests in detail, such as identifying dates, locations, names, and other relevant data.
3. Multi-language Support
Rasa NLU supports multiple languages, making it a versatile choice for global applications. Developers can train models in various languages, broadening the reach of their conversational agents.
4. Customizable Pipelines
Rasa NLU provides customizable processing pipelines that allow developers to tailor the natural language understanding process to their specific needs. Users can choose from different components for tokenization, intent classification, and entity recognition.
5. Pre-trained Models
Rasa NLU comes with pre-trained models that can be used out of the box. This feature allows developers to quickly get started with their projects without the need to build models from scratch.
6. Training and Evaluation
Rasa NLU offers tools for training and evaluating models, ensuring that developers can monitor performance and make necessary adjustments. The framework provides metrics and visualizations to help assess the accuracy of the models.
7. Integration Capabilities
Rasa NLU can be easily integrated with various platforms and services, including messaging applications, web interfaces, and backend systems. This flexibility allows developers to deploy their conversational agents in different environments.
8. Community Support
Being an open-source project, Rasa NLU has a vibrant community of developers and contributors. Users can access a wealth of resources, including documentation, tutorials, and community forums, to seek help and share knowledge.
9. Contextual Understanding
Rasa NLU can maintain the context of conversations, allowing for more meaningful interactions. This feature is essential for creating engaging and user-friendly experiences, as it enables the system to remember previous interactions and respond accordingly.
10. Open Source
Rasa NLU is completely open-source, which means developers have access to the source code and can modify it as needed. This transparency fosters innovation and collaboration within the developer community.
Use Cases
Rasa NLU can be applied in various domains and industries, showcasing its versatility and effectiveness in building conversational AI solutions. Here are some common use cases:
1. Customer Support
Businesses can use Rasa NLU to create chatbots that handle customer inquiries, provide support, and resolve issues. By automating responses to common questions, companies can improve efficiency and enhance customer satisfaction.
2. E-commerce
Rasa NLU can help e-commerce platforms build virtual shopping assistants that guide users through product searches, recommend items, and assist with transactions. This enhances the shopping experience and can lead to increased sales.
3. Healthcare
In the healthcare sector, Rasa NLU can be utilized to develop chatbots that provide medical information, schedule appointments, and answer patient queries. This can improve patient engagement and streamline administrative tasks.
4. Education
Educational institutions can leverage Rasa NLU to create tutoring systems or virtual assistants that help students with their studies. These systems can answer questions, provide resources, and track progress.
5. Travel and Hospitality
Rasa NLU can be employed in the travel industry to create chatbots that assist users in booking flights, hotels, and activities. These bots can provide personalized recommendations based on user preferences.
6. Entertainment
In the entertainment sector, Rasa NLU can power interactive storytelling applications, games, and virtual companions that engage users in dynamic conversations and experiences.
7. Internal Business Tools
Organizations can use Rasa NLU to develop internal chatbots that assist employees with HR inquiries, IT support, and other administrative tasks, improving efficiency and productivity.
Pricing
As an open-source tool, Rasa NLU is free to use, which makes it an attractive option for developers and organizations looking to implement conversational AI without incurring licensing costs. However, Rasa also offers enterprise solutions that include additional features, support, and services for businesses that require more robust capabilities and assistance.
Enterprise Features (Optional)
- Enhanced Support: Access to dedicated support and consulting services.
- Advanced Analytics: Tools for analyzing user interactions and improving models.
- Collaboration Tools: Features that facilitate teamwork among developers and stakeholders.
- Deployment Options: Solutions for deploying Rasa NLU in cloud or on-premises environments.
Comparison with Other Tools
When comparing Rasa NLU with other conversational AI frameworks, several unique selling points emerge:
1. Open Source vs. Proprietary
Rasa NLU's open-source nature allows for greater flexibility and customization compared to many proprietary solutions that lock users into specific features and pricing models.
2. Customization and Extensibility
Rasa NLU provides a high degree of customization through its pipelines and components, enabling developers to tailor the framework to their specific needs. Many other tools offer limited customization options.
3. Contextual Understanding
Rasa NLU's ability to maintain conversation context sets it apart from simpler chatbot solutions that may struggle to handle multi-turn conversations effectively.
4. Community and Ecosystem
The thriving community around Rasa NLU offers a wealth of resources, including tutorials, documentation, and forums, which can be invaluable for developers looking for support and guidance.
5. Language Support
Rasa NLU supports multiple languages, making it a suitable choice for global applications, whereas some other tools may focus primarily on English or a limited number of languages.
6. Integration Capabilities
Rasa NLU's flexibility in integration allows developers to connect their conversational agents with various platforms and services, enhancing the overall functionality of their applications.
FAQ
Q1: Is Rasa NLU suitable for beginners?
A1: Yes, Rasa NLU provides extensive documentation and tutorials that can help beginners get started with building conversational AI applications. The community support also offers valuable resources for learning.
Q2: Can I use Rasa NLU for commercial purposes?
A2: Absolutely! Rasa NLU is open-source and can be used for commercial applications without any licensing fees. However, if you require enterprise-level support or features, consider exploring Rasa's enterprise offerings.
Q3: How do I train a model in Rasa NLU?
A3: Training a model in Rasa NLU involves defining intents, entities, and training data in a specific format. You can then use the Rasa CLI or API to train your model and evaluate its performance.
Q4: What programming languages does Rasa NLU support?
A4: Rasa NLU is primarily built in Python, but it can be integrated with applications developed in various programming languages through APIs and webhooks.
Q5: Can Rasa NLU handle multiple intents in a single user input?
A5: Yes, Rasa NLU can recognize multiple intents within a single user input, allowing for more complex interactions and responses.
Q6: How does Rasa NLU handle entity recognition?
A6: Rasa NLU uses various machine learning algorithms and natural language processing techniques to identify and extract entities from user inputs, ensuring that relevant information is captured accurately.
Q7: Is there a way to test my Rasa NLU model?
A7: Yes, Rasa NLU provides evaluation tools that allow you to test your model's performance using various metrics. You can also simulate user interactions to see how the model responds.
Q8: What are the system requirements for running Rasa NLU?
A8: Rasa NLU can run on any system that supports Python, but it is recommended to use a machine with sufficient memory and processing power, especially for training large models.
By leveraging Rasa NLU, developers can create powerful, customizable conversational agents that enhance user engagement and streamline interactions in a variety of applications. Its open-source nature, extensive features, and supportive community make it a compelling choice for anyone looking to delve into the world of conversational AI.
Ready to try it out?
Go to Rasa NLU