protonAutoML
ProtonAutoML is an automated machine learning tool aimed at simplifying data analysis and model development, though its website is currently inaccessible.
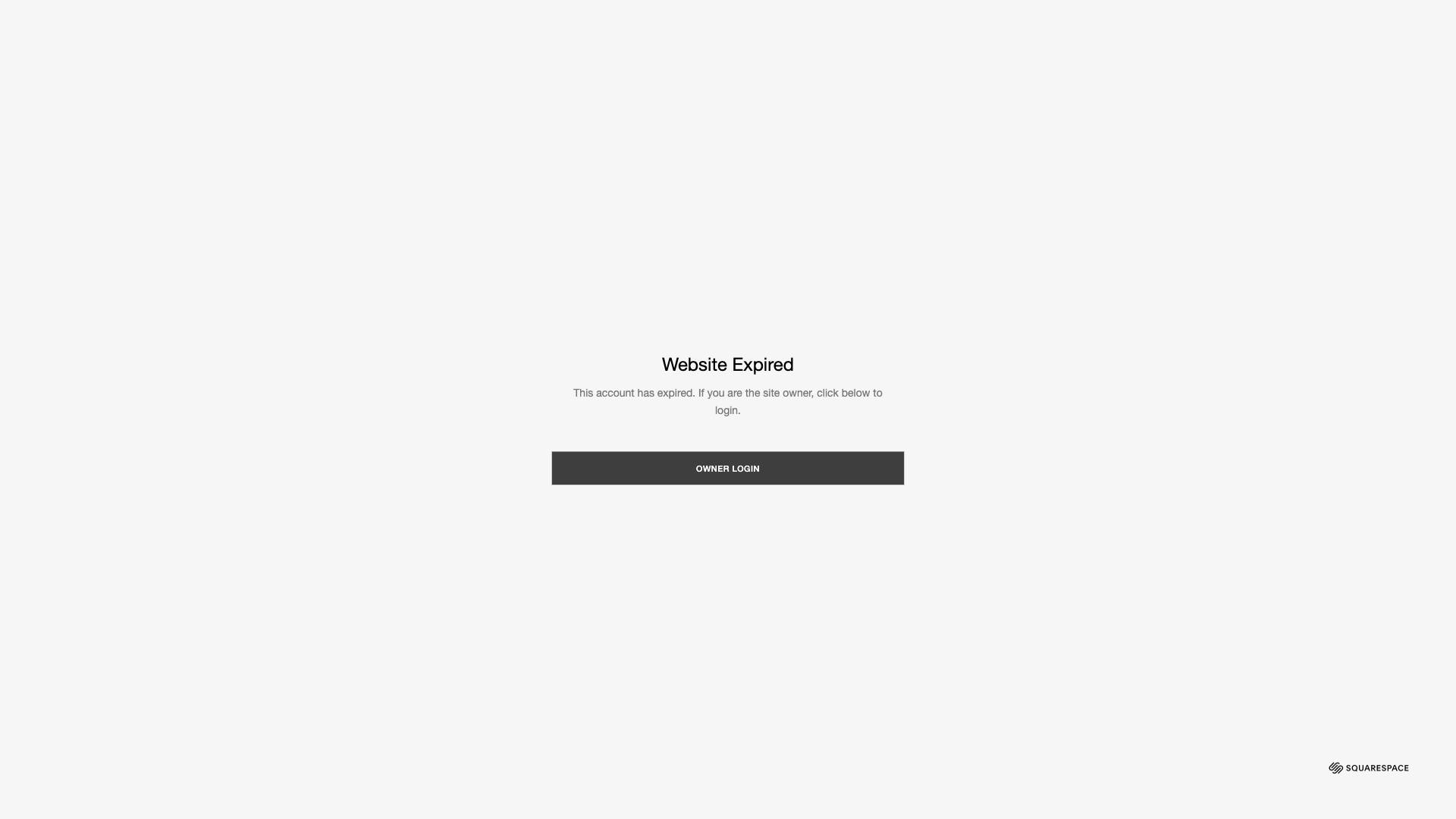
Tags
Useful for
- 1.What is protonAutoML?
- 2.Features
- 2.1.1. Automated Data Preprocessing
- 2.2.2. Model Selection
- 2.3.3. Hyperparameter Tuning
- 2.4.4. Model Evaluation
- 2.5.5. Deployment Options
- 2.6.6. User-Friendly Interface
- 2.7.7. Collaboration Features
- 3.Use Cases
- 3.1.1. Healthcare
- 3.2.2. Finance
- 3.3.3. Retail
- 3.4.4. Manufacturing
- 3.5.5. Marketing
- 4.Pricing
- 5.Comparison with Other Tools
- 5.1.1. H2O.ai
- 5.2.2. Google AutoML
- 5.3.3. DataRobot
- 5.4.4. RapidMiner
- 6.FAQ
- 6.1.What types of data can protonAutoML handle?
- 6.2.Do I need programming skills to use protonAutoML?
- 6.3.Can I integrate protonAutoML with my existing systems?
- 6.4.Is there support available for users?
- 6.5.How does protonAutoML ensure model performance?
- 6.6.Can I collaborate with my team on projects?
What is protonAutoML?
protonAutoML is an automated machine learning (AutoML) tool designed to simplify the process of building, training, and deploying machine learning models. By leveraging advanced algorithms and user-friendly interfaces, protonAutoML aims to empower both novice and experienced data scientists to create predictive models without the need for extensive programming knowledge or expertise in machine learning.
The tool automates various stages of the machine learning workflow, including data preprocessing, feature selection, model selection, hyperparameter tuning, and model evaluation. This allows users to focus on deriving insights from their data rather than getting bogged down in the technical complexities of machine learning.
Features
protonAutoML offers a range of features that cater to different aspects of the machine learning process. Here are some of the key features:
1. Automated Data Preprocessing
- Data Cleaning: Automatically identifies and handles missing values, outliers, and duplicates.
- Feature Engineering: Suggests and generates new features based on existing data to improve model performance.
- Normalization and Scaling: Applies necessary transformations to ensure that data is in the right format for modeling.
2. Model Selection
- Wide Range of Algorithms: Supports various machine learning algorithms, including decision trees, random forests, gradient boosting, and neural networks.
- Algorithm Benchmarking: Compares the performance of different algorithms on the same dataset to recommend the best option.
3. Hyperparameter Tuning
- Automated Optimization: Uses techniques like grid search and random search to find the optimal hyperparameters for selected models.
- Cross-Validation: Employs k-fold cross-validation to ensure that model performance is robust and not dependent on a specific train-test split.
4. Model Evaluation
- Performance Metrics: Provides a variety of metrics (accuracy, precision, recall, F1-score, ROC-AUC) to evaluate model performance.
- Visualizations: Offers graphical representations of model performance, including confusion matrices and ROC curves.
5. Deployment Options
- Export Models: Allows users to export trained models in various formats (e.g., PMML, ONNX) for integration into applications.
- API Integration: Facilitates easy deployment of models via RESTful APIs, enabling real-time predictions.
6. User-Friendly Interface
- Intuitive Dashboard: Features a clean and organized dashboard that simplifies navigation and enhances user experience.
- Guided Workflows: Offers step-by-step guidance throughout the machine learning process, making it accessible for beginners.
7. Collaboration Features
- Team Workspaces: Enables multiple users to collaborate on projects, share insights, and manage workflows collectively.
- Version Control: Keeps track of changes made to models and datasets, allowing teams to revert to previous versions if necessary.
Use Cases
protonAutoML can be applied across various industries and domains, offering solutions to diverse challenges. Here are some common use cases:
1. Healthcare
- Predictive Analytics: Analyze patient data to predict disease outcomes, identify high-risk patients, and optimize treatment plans.
- Medical Imaging: Automate the analysis of imaging data (e.g., X-rays, MRIs) to assist radiologists in diagnosing conditions.
2. Finance
- Credit Scoring: Build models to assess the creditworthiness of loan applicants based on their financial history and behavior.
- Fraud Detection: Identify patterns of fraudulent transactions and flag suspicious activities in real-time.
3. Retail
- Customer Segmentation: Use clustering algorithms to segment customers based on purchasing behavior, enabling targeted marketing strategies.
- Sales Forecasting: Predict future sales trends based on historical data, allowing for better inventory management and resource allocation.
4. Manufacturing
- Predictive Maintenance: Analyze sensor data from machinery to predict equipment failures and schedule maintenance proactively.
- Quality Control: Automate the inspection of products using machine learning models to detect defects and ensure quality standards.
5. Marketing
- Churn Prediction: Analyze customer data to predict churn rates and develop retention strategies to keep customers engaged.
- Campaign Optimization: Evaluate the effectiveness of marketing campaigns and recommend adjustments based on data-driven insights.
Pricing
While specific pricing details for protonAutoML are not available due to the website's expiration, AutoML tools typically offer various pricing tiers based on features and usage. Here’s a general idea of how pricing structures are often organized:
- Free Tier: Limited access to basic features, ideal for individual users or small projects.
- Basic Plan: A monthly subscription that includes essential features for small teams or businesses.
- Pro Plan: Advanced features and capabilities, suitable for larger teams and organizations with more complex needs.
- Enterprise Solutions: Custom pricing based on specific requirements, including dedicated support and tailored features.
It is advisable for potential users to reach out to the protonAutoML team for a detailed pricing structure and available plans.
Comparison with Other Tools
When comparing protonAutoML with other AutoML tools in the market, several factors come into play, such as ease of use, range of features, and pricing. Here’s how protonAutoML stacks up against some popular alternatives:
1. H2O.ai
- Similarities: Both tools offer automated data preprocessing, model selection, and hyperparameter tuning.
- Differences: H2O.ai has a steeper learning curve and may require more technical knowledge compared to the user-friendly interface of protonAutoML.
2. Google AutoML
- Similarities: Both platforms provide robust automated machine learning capabilities.
- Differences: Google AutoML is more integrated into the Google Cloud ecosystem, which may be a barrier for organizations not using Google services. protonAutoML, on the other hand, is designed to be platform-agnostic.
3. DataRobot
- Similarities: Both tools focus on automating the machine learning workflow and provide collaboration features.
- Differences: DataRobot tends to have a higher price point and may be more suited for large enterprises, while protonAutoML aims to be accessible to a broader audience.
4. RapidMiner
- Similarities: Both tools offer visual interfaces and support for various machine learning algorithms.
- Differences: RapidMiner has a more extensive focus on data science workflows beyond just AutoML, which may be overwhelming for users solely interested in automated model building.
FAQ
What types of data can protonAutoML handle?
protonAutoML is designed to work with various types of structured data, including numerical, categorical, and time-series data. It may also support unstructured data, depending on the specific features offered.
Do I need programming skills to use protonAutoML?
No, protonAutoML is built to be user-friendly and accessible for users without programming skills. Its intuitive interface and guided workflows make it suitable for beginners in machine learning.
Can I integrate protonAutoML with my existing systems?
Yes, protonAutoML offers API integration, allowing users to deploy models and make predictions in real-time using their existing applications and systems.
Is there support available for users?
While specific support options are not detailed due to the website's expiration, most AutoML tools typically offer customer support through documentation, forums, and direct assistance.
How does protonAutoML ensure model performance?
protonAutoML employs techniques like cross-validation and provides various performance metrics to evaluate model effectiveness. Users can also visualize model performance through graphical representations.
Can I collaborate with my team on projects?
Yes, protonAutoML includes collaboration features that allow multiple users to work together on projects, share insights, and manage workflows effectively.
In conclusion, protonAutoML is a powerful and user-friendly tool that democratizes access to machine learning by automating complex processes. Its comprehensive feature set, diverse use cases, and collaborative capabilities make it a valuable asset for organizations looking to leverage data-driven insights.
Ready to try it out?
Go to protonAutoML