NVIDIA Deep Learning SDK
NVIDIA Deep Learning SDK empowers developers to build high-performance, GPU-accelerated AI applications across various frameworks and industries.
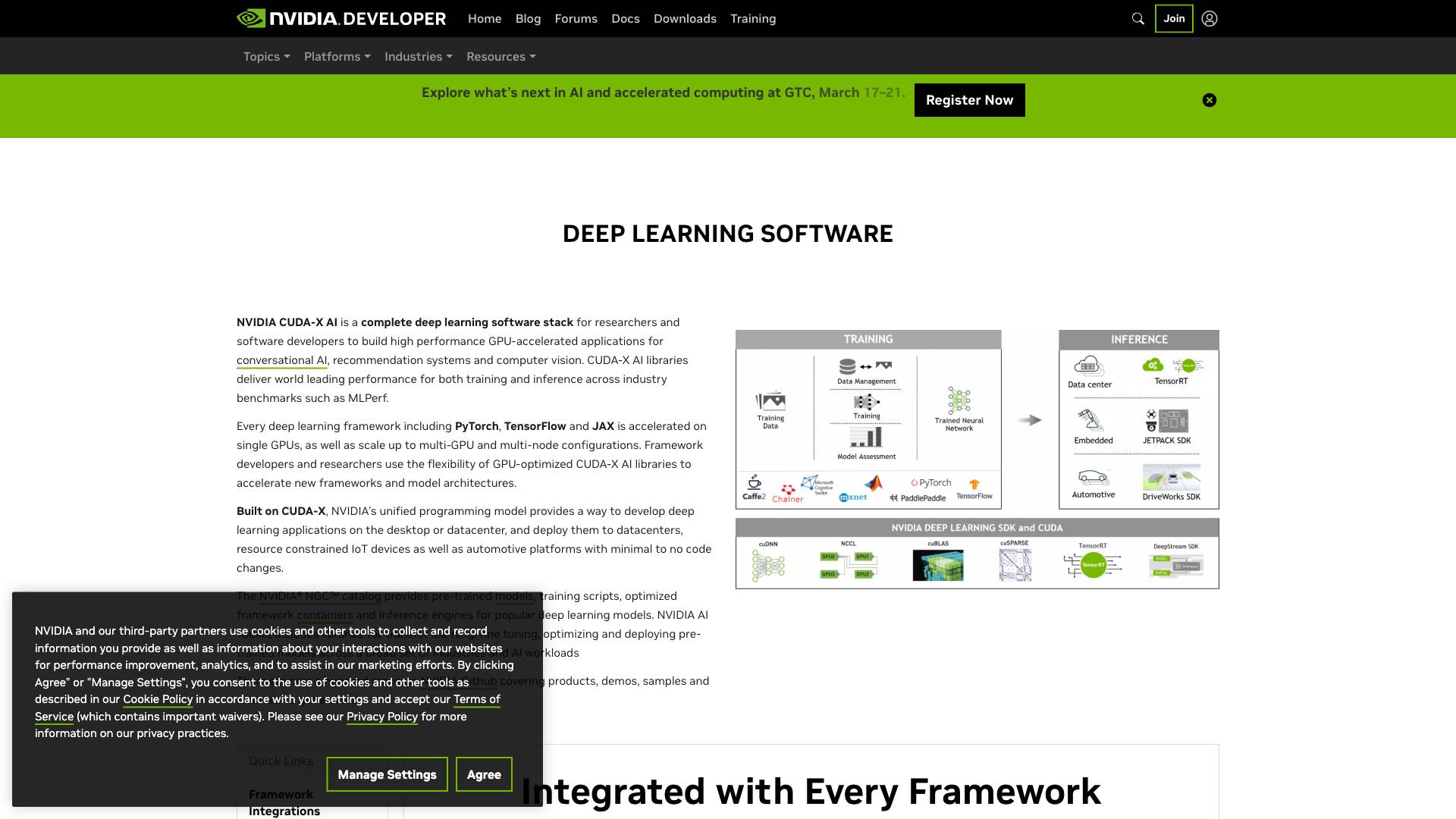
Tags
Useful for
- 1.What is NVIDIA Deep Learning SDK?
- 1.1.Features
- 1.1.1.1. CUDA-X AI Libraries
- 1.1.2.2. Pre-Trained Models and Training Scripts
- 1.1.3.3. High-Performance Inference SDKs
- 1.1.4.4. Multi-GPU and Multi-Node Scalability
- 1.1.5.5. Developer Tools
- 1.1.6.6. Data Loading and Augmentation
- 1.1.7.7. Interactive Tools for Model Management
- 1.2.Use Cases
- 1.2.1.1. Conversational AI
- 1.2.2.2. Recommendation Systems
- 1.2.3.3. Computer Vision
- 1.2.4.4. Natural Language Processing (NLP)
- 1.2.5.5. Healthcare Applications
- 1.3.Pricing
- 1.4.Comparison with Other Tools
- 1.4.1.1. Performance
- 1.4.2.2. Comprehensive Ecosystem
- 1.4.3.3. Scalability
- 1.4.4.4. Industry-Specific Solutions
- 1.4.5.5. Developer Support
- 1.5.FAQ
- 1.5.1.1. What deep learning frameworks are supported by the NVIDIA Deep Learning SDK?
- 1.5.2.2. Can I use the NVIDIA Deep Learning SDK on cloud platforms?
- 1.5.3.3. What types of applications can I build with the NVIDIA Deep Learning SDK?
- 1.5.4.4. Is there a free version of the NVIDIA Deep Learning SDK?
- 1.5.5.5. How does the NVIDIA Deep Learning SDK facilitate model deployment?
- 1.5.6.6. What resources are available for learning how to use the NVIDIA Deep Learning SDK?
- 1.5.7.7. Are there any specific hardware requirements for using the NVIDIA Deep Learning SDK?
What is NVIDIA Deep Learning SDK?
The NVIDIA Deep Learning SDK is a comprehensive software stack designed to empower researchers and software developers in building high-performance, GPU-accelerated applications. It caters to various applications, including conversational AI, recommendation systems, and computer vision. Leveraging the power of NVIDIA’s CUDA-X AI, this SDK provides a unified programming model that allows developers to create, train, and deploy deep learning models efficiently across a wide range of platforms, from desktops to data centers and even resource-constrained IoT devices.
The SDK encompasses a suite of libraries, tools, and pre-trained models that facilitate the development of deep learning applications. It is optimized for various deep learning frameworks such as PyTorch, TensorFlow, and JAX, ensuring that users can achieve world-class performance for both training and inference tasks.
Features
The NVIDIA Deep Learning SDK is packed with robust features that enhance the development and deployment of deep learning models. Here are some of its key features:
1. CUDA-X AI Libraries
- Performance Optimization: The SDK includes libraries optimized for GPU acceleration, delivering superior performance for training and inference tasks across multiple industry benchmarks.
- Framework Integration: It supports popular deep learning frameworks, such as PyTorch, TensorFlow, and JAX, enabling seamless integration and acceleration of models.
2. Pre-Trained Models and Training Scripts
- NVIDIA NGC Catalog: Access to a rich repository of pre-trained models, optimized framework containers, and training scripts that help developers kickstart their projects quickly.
- Transfer Learning: Libraries and tools to perform transfer learning and fine-tuning of pre-trained models, maximizing accuracy and performance for specific applications.
3. High-Performance Inference SDKs
- Low Latency and High Throughput: The SDK is designed to minimize latency and maximize throughput for deep learning inference applications, making it ideal for real-time applications.
- Optimizations for Production: Features such as lower precision inference (FP16 and INT8) significantly enhance performance compared to CPU-only platforms.
4. Multi-GPU and Multi-Node Scalability
- Scalability: The SDK supports scaling training jobs from a single GPU to thousands of GPUs in DGX SuperPods, allowing for the efficient handling of large models and datasets.
- NVIDIA Collective Communications Library (NCCL): This library accelerates multi-GPU communication, facilitating efficient model training across multiple GPUs.
5. Developer Tools
- Nsight Systems and Nsight Compute: Comprehensive performance analysis and profiling tools that help developers optimize GPU utilization and application performance.
- Kubernetes Integration: Enables seamless deployment and scaling of GPU-accelerated applications across multi-cloud environments.
6. Data Loading and Augmentation
- DALI (Data Loading Library): A GPU-accelerated library for optimizing data pipelines, ensuring efficient data loading and augmentation to enhance model training.
7. Interactive Tools for Model Management
- DIGITS: An interactive platform for managing data, designing, and training computer vision networks on multi-GPU systems, providing real-time performance monitoring.
Use Cases
The NVIDIA Deep Learning SDK can be applied across various industries and applications, showcasing its versatility and power. Here are some notable use cases:
1. Conversational AI
- Voice Assistants: Building sophisticated voice recognition systems that can understand and respond to natural language queries.
- Chatbots: Developing AI-driven chatbots that can engage users in meaningful conversations, improving customer service experiences.
2. Recommendation Systems
- Personalized Content Delivery: Utilizing deep learning to analyze user behavior and preferences, delivering personalized content recommendations in real-time.
- E-commerce: Enhancing online shopping experiences by providing tailored product suggestions based on user interactions and historical data.
3. Computer Vision
- Image Classification: Training models to classify images into categories, which can be used in various applications such as medical imaging and autonomous vehicles.
- Object Detection: Developing systems that can identify and locate objects within images or video streams, useful in surveillance and security applications.
4. Natural Language Processing (NLP)
- Sentiment Analysis: Analyzing text data to determine sentiment, allowing businesses to gauge customer opinions and feedback effectively.
- Text Generation: Creating models that can generate coherent and contextually relevant text, enhancing content creation and automation.
5. Healthcare Applications
- Medical Imaging: Leveraging deep learning to analyze medical images for diagnosis and treatment planning, improving patient outcomes.
- Predictive Analytics: Using AI to predict patient outcomes based on historical data, aiding in proactive healthcare management.
Pricing
While specific pricing details for the NVIDIA Deep Learning SDK are not explicitly mentioned, it is important to note that the SDK is designed to work with NVIDIA's hardware products, such as GPUs. The cost may vary depending on the GPU hardware used, the scale of deployment, and any additional services or support required. Organizations interested in utilizing the SDK should consider their specific needs and consult with NVIDIA or authorized resellers for tailored pricing options.
Comparison with Other Tools
When comparing the NVIDIA Deep Learning SDK with other deep learning tools, several unique selling points emerge that set it apart:
1. Performance
- The NVIDIA Deep Learning SDK is specifically optimized for NVIDIA GPUs, ensuring superior performance for both training and inference tasks compared to CPU-based frameworks or those optimized for other hardware.
2. Comprehensive Ecosystem
- Unlike many other deep learning frameworks, the NVIDIA SDK offers a complete ecosystem that includes pre-trained models, optimized libraries, and tools for deployment, making it easier for developers to build and scale AI applications.
3. Scalability
- The ability to scale from single GPU setups to multi-node configurations enables organizations to handle increasingly complex models and larger datasets without significant changes to their codebase.
4. Industry-Specific Solutions
- The SDK provides tailored solutions for various industries, including healthcare, automotive, and finance, offering specialized tools and libraries that address specific challenges in these domains.
5. Developer Support
- NVIDIA offers extensive documentation, tutorials, and community support through forums and resources, making it easier for developers to get started and troubleshoot issues.
FAQ
1. What deep learning frameworks are supported by the NVIDIA Deep Learning SDK?
The SDK supports popular frameworks such as PyTorch, TensorFlow, and JAX, allowing developers to leverage GPU acceleration with their preferred tools.
2. Can I use the NVIDIA Deep Learning SDK on cloud platforms?
Yes, the SDK is designed to work seamlessly on cloud platforms, enabling organizations to deploy GPU-accelerated applications in a scalable and cost-effective manner.
3. What types of applications can I build with the NVIDIA Deep Learning SDK?
You can build a wide range of applications, including conversational AI, recommendation systems, computer vision applications, and natural language processing systems.
4. Is there a free version of the NVIDIA Deep Learning SDK?
While the SDK itself is free to use, it requires NVIDIA GPU hardware for optimal performance. Organizations should consider the costs associated with acquiring the necessary hardware.
5. How does the NVIDIA Deep Learning SDK facilitate model deployment?
The SDK provides tools and libraries that allow developers to easily deploy models across various environments, including data centers, edge devices, and cloud platforms, with minimal code changes.
6. What resources are available for learning how to use the NVIDIA Deep Learning SDK?
NVIDIA offers extensive documentation, tutorials, and community forums where developers can find resources, share knowledge, and seek support for their projects.
7. Are there any specific hardware requirements for using the NVIDIA Deep Learning SDK?
To achieve optimal performance, it is recommended to use NVIDIA GPUs that support CUDA, as the SDK is specifically optimized for these hardware components.
In conclusion, the NVIDIA Deep Learning SDK is an invaluable tool for researchers and developers looking to harness the power of deep learning for various applications. Its robust features, extensive use cases, and unique advantages make it a leading choice in the field of artificial intelligence.
Ready to try it out?
Go to NVIDIA Deep Learning SDK