Nvidia Apex
Nvidia Apex is a powerful toolkit designed to optimize and accelerate deep learning training for AI developers.
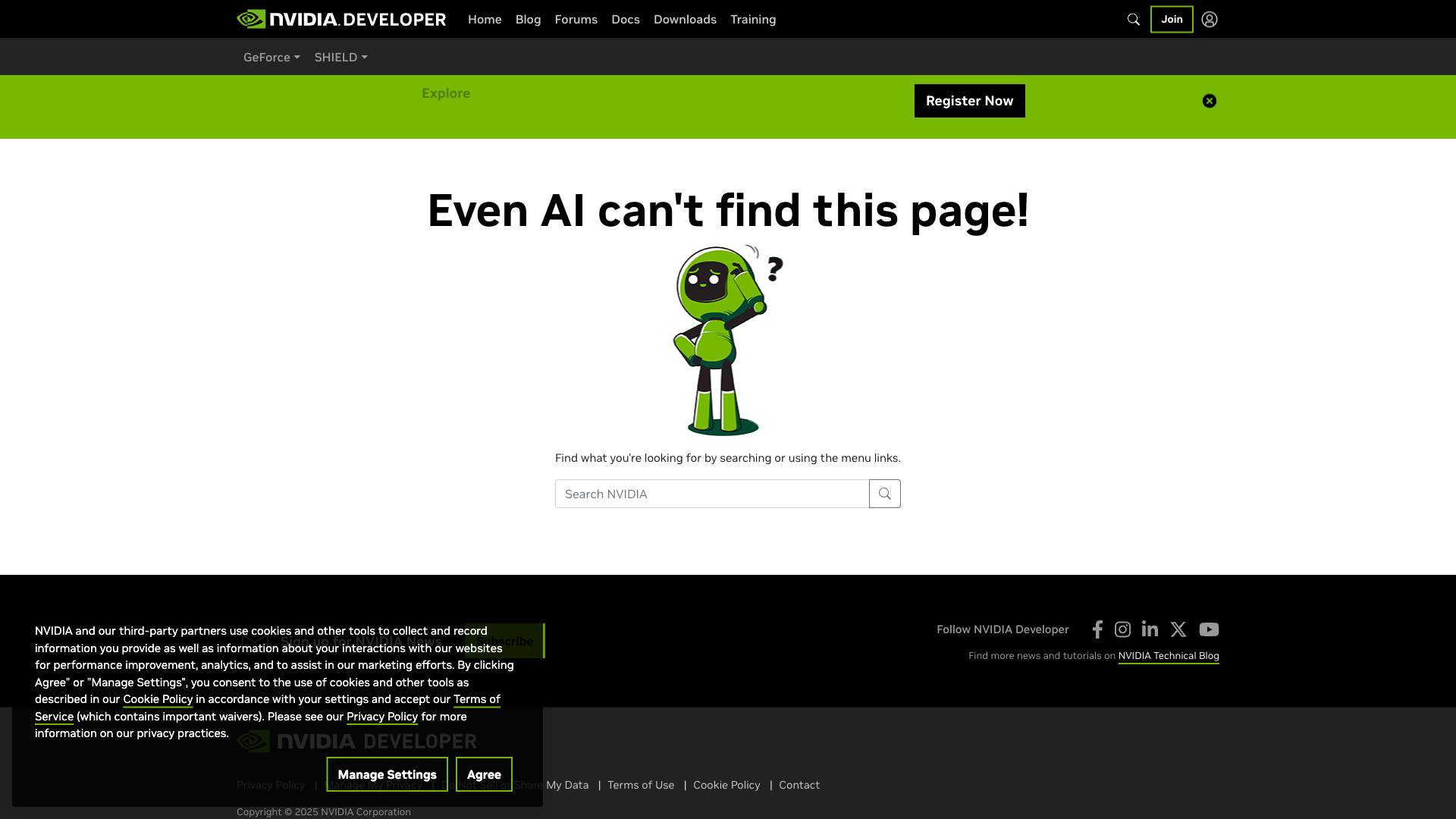
Tags
Useful for
- 1.What is Nvidia Apex?
- 2.Features
- 2.1.1. Mixed Precision Training
- 2.2.2. Performance Optimization
- 2.3.3. Compatibility
- 2.4.4. User-Friendly API
- 2.5.5. Community and Support
- 3.Use Cases
- 3.1.1. Image Classification
- 3.2.2. Natural Language Processing (NLP)
- 3.3.3. Reinforcement Learning
- 3.4.4. Generative Models
- 3.5.5. Research and Development
- 4.Pricing
- 5.Comparison with Other Tools
- 5.1.1. TensorFlow Mixed Precision
- 5.2.2. Horovod
- 5.3.3. DeepSpeed
- 6.FAQ
- 6.1.1. What types of GPUs are compatible with Nvidia Apex?
- 6.2.2. Do I need to modify my existing code to use Nvidia Apex?
- 6.3.3. Is Nvidia Apex suitable for beginners in deep learning?
- 6.4.4. Can I use Nvidia Apex with frameworks other than PyTorch?
- 6.5.5. How often is Nvidia Apex updated?
What is Nvidia Apex?
Nvidia Apex is a powerful suite of tools and libraries designed to optimize deep learning and artificial intelligence (AI) applications on Nvidia GPUs. Developed by Nvidia, a leader in graphics processing and AI technology, Apex aims to streamline and enhance the training of neural networks, making it easier for developers and researchers to implement state-of-the-art models efficiently. Apex is particularly known for its support of mixed precision training, which allows for faster computation and reduced memory usage without sacrificing model accuracy.
Features
Nvidia Apex boasts a variety of features that cater to both novice and experienced developers in the field of deep learning:
1. Mixed Precision Training
- Automatic Mixed Precision (AMP): Apex provides an easy-to-use interface for implementing mixed precision training, which combines 16-bit and 32-bit floating-point arithmetic. This approach significantly speeds up training times while conserving GPU memory.
- Dynamic Loss Scaling: To preserve numerical stability during training, Apex includes dynamic loss scaling, which adjusts the scale of the loss value based on the gradients. This ensures that small gradient values do not underflow when using lower precision.
2. Performance Optimization
- Efficient Memory Usage: By using mixed precision, Apex reduces the memory footprint of models, allowing for larger batch sizes and more complex architectures to be trained on a single GPU.
- Speed Improvements: The optimization techniques integrated into Apex can lead to substantial reductions in training time, making it possible to experiment with more models and configurations in a shorter period.
3. Compatibility
- Seamless Integration: Apex is built to work seamlessly with popular deep learning frameworks such as PyTorch. This compatibility allows developers to easily incorporate Apex into their existing workflows without extensive code modifications.
- Support for Various Nvidia GPUs: Apex is optimized for a wide range of Nvidia GPUs, from consumer-grade cards to high-end data center models, ensuring broad accessibility for users.
4. User-Friendly API
- Simplified Implementation: Apex provides a straightforward API that abstracts away the complexities of mixed precision training, making it accessible for developers at all skill levels.
- Extensive Documentation: Nvidia offers comprehensive documentation and examples to help users get started quickly and effectively utilize Apex’s features.
5. Community and Support
- Active Community: As part of the Nvidia ecosystem, Apex benefits from an active community of developers and researchers who contribute to its improvement and provide support through forums and discussions.
- Regular Updates: Nvidia frequently updates Apex to include new features, performance enhancements, and compatibility improvements, ensuring that users have access to the latest advancements in deep learning technology.
Use Cases
Nvidia Apex is versatile and can be applied across various domains and industries where deep learning is utilized. Some of the most prominent use cases include:
1. Image Classification
Apex can be used to train convolutional neural networks (CNNs) for image classification tasks. By leveraging mixed precision training, developers can train larger models with higher accuracy in less time, making it ideal for applications in fields such as healthcare, security, and autonomous vehicles.
2. Natural Language Processing (NLP)
In NLP tasks, such as sentiment analysis or machine translation, Apex can accelerate the training of transformer models like BERT and GPT. The reduction in training time allows for more rapid iterations and experimentation, leading to improved model performance.
3. Reinforcement Learning
Apex is also suitable for reinforcement learning applications, where training can be computationally intensive. Its ability to optimize memory usage and reduce training times makes it easier to train complex agents in environments that require extensive exploration and learning.
4. Generative Models
For tasks involving generative models, such as GANs (Generative Adversarial Networks), Apex helps in efficiently training models that generate high-quality images or other data types. The performance improvements enable researchers to explore more intricate architectures and training strategies.
5. Research and Development
Researchers in academia and industry can utilize Apex to experiment with new deep learning techniques and algorithms. The combination of performance optimizations and user-friendly API allows for rapid prototyping and testing of innovative ideas.
Pricing
Nvidia Apex is a free tool available to all users who have compatible Nvidia GPUs and the required deep learning frameworks installed. There are no licensing fees associated with its use, making it an attractive option for developers and researchers looking to optimize their deep learning workflows without incurring additional costs.
Comparison with Other Tools
When comparing Nvidia Apex with other deep learning optimization tools, several factors come into play, including performance, ease of use, and community support. Here are some key points of comparison:
1. TensorFlow Mixed Precision
- Performance: Both Nvidia Apex and TensorFlow's mixed precision training offer similar performance benefits. However, Apex is specifically optimized for Nvidia GPUs, which may yield better results in certain scenarios.
- Ease of Use: Apex provides a more straightforward API for mixed precision training compared to TensorFlow, making it easier for users to implement without extensive modifications to their code.
2. Horovod
- Distributed Training: While Horovod focuses on distributed training across multiple GPUs and nodes, Apex is primarily concerned with optimizing single-GPU training through mixed precision. Users looking for distributed training solutions may choose Horovod, while those focused on single-GPU optimization may prefer Apex.
- Integration: Both tools can be integrated into existing workflows, but Apex's seamless compatibility with PyTorch makes it particularly appealing for users of that framework.
3. DeepSpeed
- Performance: DeepSpeed is another tool designed to optimize deep learning training, with a focus on large-scale models. While it offers impressive performance improvements, Apex's mixed precision training is easier to implement for developers working with PyTorch.
- Complexity: DeepSpeed may require more configuration and setup compared to Apex, making it less accessible for users who are new to deep learning optimization.
Overall, Nvidia Apex stands out for its user-friendly approach to mixed precision training and its optimization for Nvidia hardware, making it a preferred choice for many developers and researchers.
FAQ
1. What types of GPUs are compatible with Nvidia Apex?
Nvidia Apex is compatible with a wide range of Nvidia GPUs, from consumer-grade models like the GeForce RTX series to professional-grade GPUs such as the Tesla and A100 series. Users should ensure that their GPU supports CUDA for optimal performance.
2. Do I need to modify my existing code to use Nvidia Apex?
While some modifications may be necessary to implement mixed precision training, Apex is designed to be user-friendly and minimize the amount of code changes required. The extensive documentation and examples provided by Nvidia can help guide users through the process.
3. Is Nvidia Apex suitable for beginners in deep learning?
Yes, Nvidia Apex is designed with ease of use in mind. Its straightforward API and comprehensive documentation make it accessible for developers at all skill levels, including those who are new to deep learning.
4. Can I use Nvidia Apex with frameworks other than PyTorch?
Currently, Nvidia Apex is primarily optimized for use with PyTorch. While it may be possible to integrate it with other frameworks, users may not experience the same level of performance and support as with PyTorch.
5. How often is Nvidia Apex updated?
Nvidia regularly updates Apex to include new features, performance enhancements, and compatibility improvements. Users can expect ongoing support and updates as Nvidia continues to innovate in the field of deep learning.
In conclusion, Nvidia Apex is a robust tool that provides significant benefits for deep learning practitioners. With its focus on mixed precision training, performance optimization, and user-friendly design, it has become an essential resource for developers and researchers looking to enhance their AI applications.
Ready to try it out?
Go to Nvidia Apex