MLFlow
MLflow is an open-source MLOps platform that streamlines the entire machine learning and generative AI lifecycle, integrating with various tools for seamless model management.
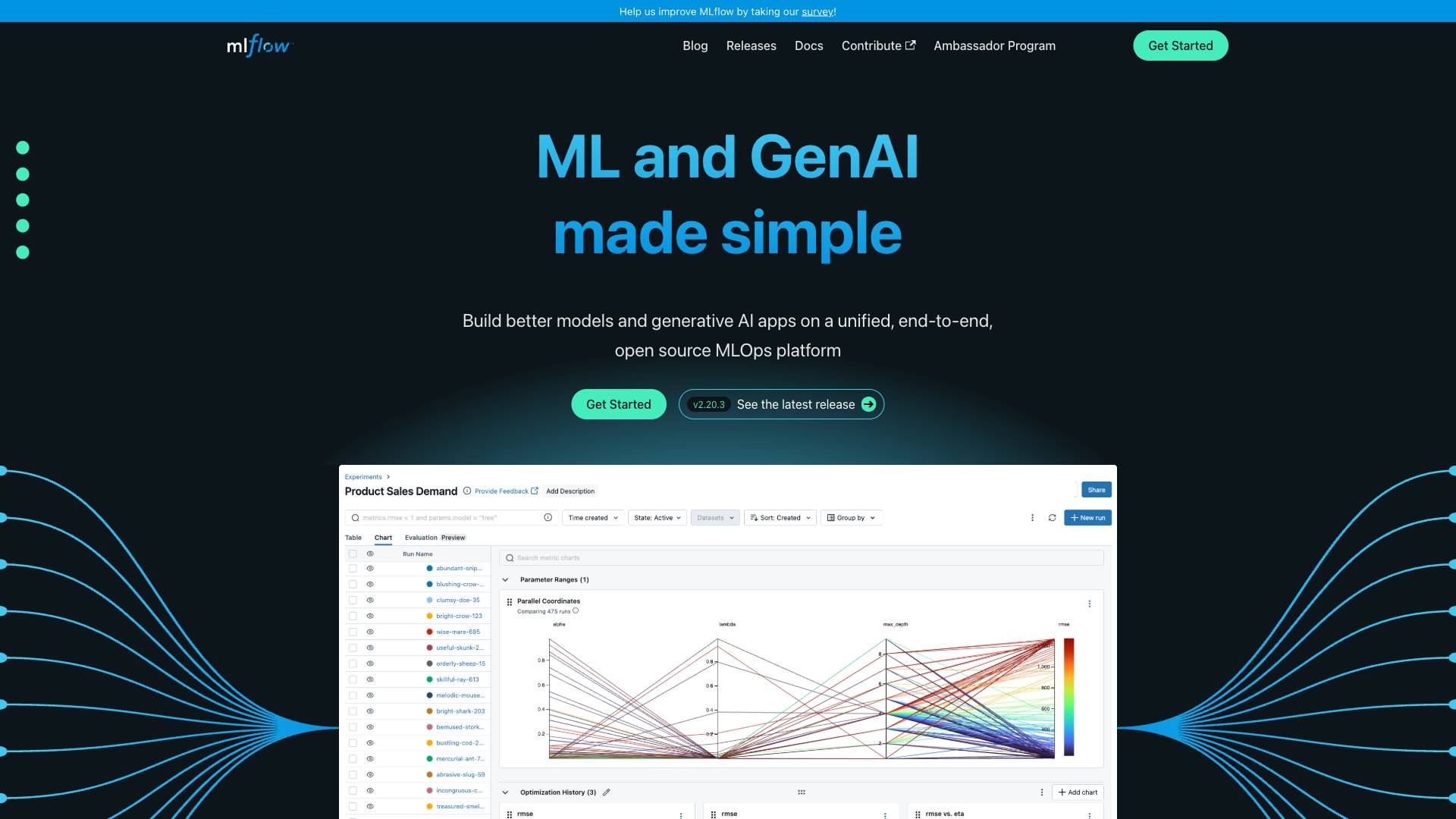
Tags
Useful for
- 1.What is MLFlow?
- 2.Features
- 2.1.Experiment Tracking
- 2.2.Visualization
- 2.3.Model Registry
- 2.4.Model Serving
- 2.5.Generative AI Support
- 2.6.Integration with Popular Tools
- 2.7.Secure Hosting
- 2.8.Comprehensive Learning Resources
- 3.Use Cases
- 3.1.Model Development
- 3.2.Collaboration Among Teams
- 3.3.Deployment of Machine Learning Models
- 3.4.Generative AI Applications
- 3.5.Continuous Integration and Delivery (CI/CD)
- 3.6.Educational Purposes
- 4.Pricing
- 5.Comparison with Other Tools
- 5.1.Versatility
- 5.2.Open Source vs. Proprietary Solutions
- 5.3.Community Support
- 5.4.Comprehensive Features
- 5.5.Learning Curve
- 6.FAQ
- 6.1.What programming languages does MLFlow support?
- 6.2.Can I use MLFlow with any machine learning library?
- 6.3.Is MLFlow suitable for large-scale deployments?
- 6.4.How can I contribute to MLFlow?
- 6.5.Where can I find documentation and learning resources?
- 6.6.Does MLFlow provide support for generative AI models?
- 6.7.Is there a cost associated with using MLFlow?
- 6.8.How does MLFlow handle model versioning?
- 6.9.Can I integrate MLFlow into my existing workflows?
What is MLFlow?
MLFlow is an open-source platform designed to streamline the machine learning (ML) lifecycle, enabling developers and data scientists to manage end-to-end workflows for both traditional machine learning and generative AI applications. With its comprehensive set of tools, MLFlow simplifies the complexities involved in building, deploying, and monitoring machine learning models, making it easier for teams to collaborate and innovate.
The platform is designed to be versatile and can integrate with various ML libraries and platforms, allowing users to adopt it without being locked into a specific ecosystem. MLFlow is widely used by organizations around the globe, with millions of downloads and a vibrant community of contributors.
Features
MLFlow offers a rich set of features that cater to the diverse needs of machine learning practitioners. Here are some of the key features:
Experiment Tracking
MLFlow provides robust experiment tracking capabilities, enabling users to log parameters, metrics, and artifacts from their machine learning experiments. This feature helps teams monitor their progress and compare different models effectively.
Visualization
The platform includes visualization tools that allow users to analyze their experiments and results visually. This aids in understanding model performance and making data-driven decisions.
Model Registry
MLFlow features a model registry that allows users to manage their models in a centralized repository. This includes versioning, annotations, and lifecycle management, which are essential for ensuring reproducibility and collaboration.
Model Serving
With MLFlow, users can deploy their models for inference in a scalable and secure manner. The platform supports various deployment targets, including cloud services, on-premise servers, and local environments.
Generative AI Support
MLFlow is equipped to handle generative AI projects, providing tools for prompt engineering, evaluation, and observability. This is particularly useful for teams working on advanced AI applications that leverage large language models (LLMs).
Integration with Popular Tools
MLFlow integrates seamlessly with over 25 popular tools and platforms, such as PyTorch, TensorFlow, Keras, HuggingFace, OpenAI, and more. This flexibility allows users to incorporate MLFlow into their existing workflows without major disruptions.
Secure Hosting
The platform provides secure hosting options for large language models, ensuring that sensitive data is protected while still allowing for scalable model serving.
Comprehensive Learning Resources
MLFlow offers extensive documentation, how-to guides, and tutorials that help users get started quickly and effectively. This wealth of resources is invaluable for both beginners and experienced practitioners.
Use Cases
MLFlow can be applied in a variety of scenarios, making it a versatile tool for different industries and applications. Here are some common use cases:
Model Development
Data scientists can use MLFlow to track their experiments during the model development phase. By logging parameters and metrics, they can evaluate the performance of different algorithms and hyperparameters, leading to better model selection.
Collaboration Among Teams
In organizations with multiple data science teams, MLFlow facilitates collaboration by providing a centralized model registry. Teams can share models, track changes, and ensure that everyone is working with the latest versions.
Deployment of Machine Learning Models
MLFlow simplifies the deployment process, allowing teams to move models from development to production seamlessly. This is particularly useful for organizations looking to implement machine learning solutions rapidly and efficiently.
Generative AI Applications
For teams working on generative AI projects, MLFlow provides specific tools for evaluating and managing large language models. This includes tracking fine-tuning processes and enhancing model observability.
Continuous Integration and Delivery (CI/CD)
MLFlow can be integrated into CI/CD pipelines, enabling automated testing and deployment of machine learning models. This helps organizations maintain high standards of quality and reliability in their ML applications.
Educational Purposes
Educators and trainers can leverage MLFlow to teach machine learning concepts effectively. The platform's comprehensive documentation and user-friendly interface make it an excellent resource for learning and experimentation.
Pricing
MLFlow is an open-source platform, which means that it is free to use. Users can download and install it without incurring any licensing fees. This makes MLFlow an attractive option for startups, individual developers, and organizations looking to implement machine learning solutions without a significant financial investment.
However, organizations may incur costs associated with infrastructure, such as cloud hosting or on-premise servers, depending on their deployment choices. Additionally, while the core functionalities are free, organizations may choose to invest in consulting or support services to maximize their use of the platform.
Comparison with Other Tools
When evaluating MLFlow, it is essential to compare it with other MLOps tools available in the market. Here are some key points of comparison:
Versatility
Unlike some MLOps tools that are tailored for specific frameworks or workflows, MLFlow is designed to be versatile and integrate with a wide range of ML libraries and platforms. This flexibility allows users to adopt MLFlow without being locked into a particular technology stack.
Open Source vs. Proprietary Solutions
MLFlow is open-source, which provides users with the freedom to customize and extend the platform according to their needs. In contrast, many proprietary MLOps solutions come with licensing fees and may limit customization options.
Community Support
MLFlow has a large and active community of contributors, which means users can benefit from a wealth of shared knowledge, resources, and best practices. This community-driven approach can be advantageous compared to proprietary tools that may have limited user support options.
Comprehensive Features
MLFlow offers a comprehensive set of features that cover the entire machine learning lifecycle, from experimentation to deployment. While some tools may focus on specific aspects of the MLOps process, MLFlow provides an all-in-one solution.
Learning Curve
MLFlow's user-friendly interface and extensive documentation make it accessible to users with varying levels of expertise. While some tools may have steeper learning curves, MLFlow's resources help users get up to speed quickly.
FAQ
What programming languages does MLFlow support?
MLFlow supports multiple programming languages, including Python, R, and Java. This makes it accessible to a wide range of developers and data scientists.
Can I use MLFlow with any machine learning library?
Yes, MLFlow is designed to integrate with any machine learning library or framework, including popular options like TensorFlow, PyTorch, and scikit-learn.
Is MLFlow suitable for large-scale deployments?
Absolutely. MLFlow provides features for secure hosting and scalable model serving, making it suitable for large-scale deployments in production environments.
How can I contribute to MLFlow?
MLFlow is an open-source project, and contributions are welcome. Users can participate by reporting issues, submitting code, or improving documentation. The community is active, and there are many opportunities for collaboration.
Where can I find documentation and learning resources?
MLFlow offers extensive documentation, how-to guides, and tutorials on its website. These resources cover a wide range of topics, from getting started to advanced features.
Does MLFlow provide support for generative AI models?
Yes, MLFlow includes specific features for managing and evaluating generative AI models, making it an excellent choice for teams working on advanced AI applications.
Is there a cost associated with using MLFlow?
MLFlow is free to use as an open-source platform. However, users may incur costs related to infrastructure, such as cloud hosting or on-premise servers.
How does MLFlow handle model versioning?
MLFlow's model registry allows users to manage model versions effectively. Users can log different versions of their models, annotate them, and track changes over time.
Can I integrate MLFlow into my existing workflows?
Yes, MLFlow is designed to integrate seamlessly with existing workflows and tools, making it easy to adopt without significant disruptions.
In conclusion, MLFlow is a powerful and flexible MLOps platform that caters to the diverse needs of machine learning practitioners. Its comprehensive features, open-source nature, and strong community support make it an excellent choice for organizations looking to streamline their ML workflows and enhance collaboration among teams. Whether you're developing traditional machine learning models or working on cutting-edge generative AI applications, MLFlow provides the tools you need to succeed.
Ready to try it out?
Go to MLFlow