
Metaflow
Metaflow is an open-source framework that simplifies building, managing, and deploying real-life ML and data science projects efficiently.
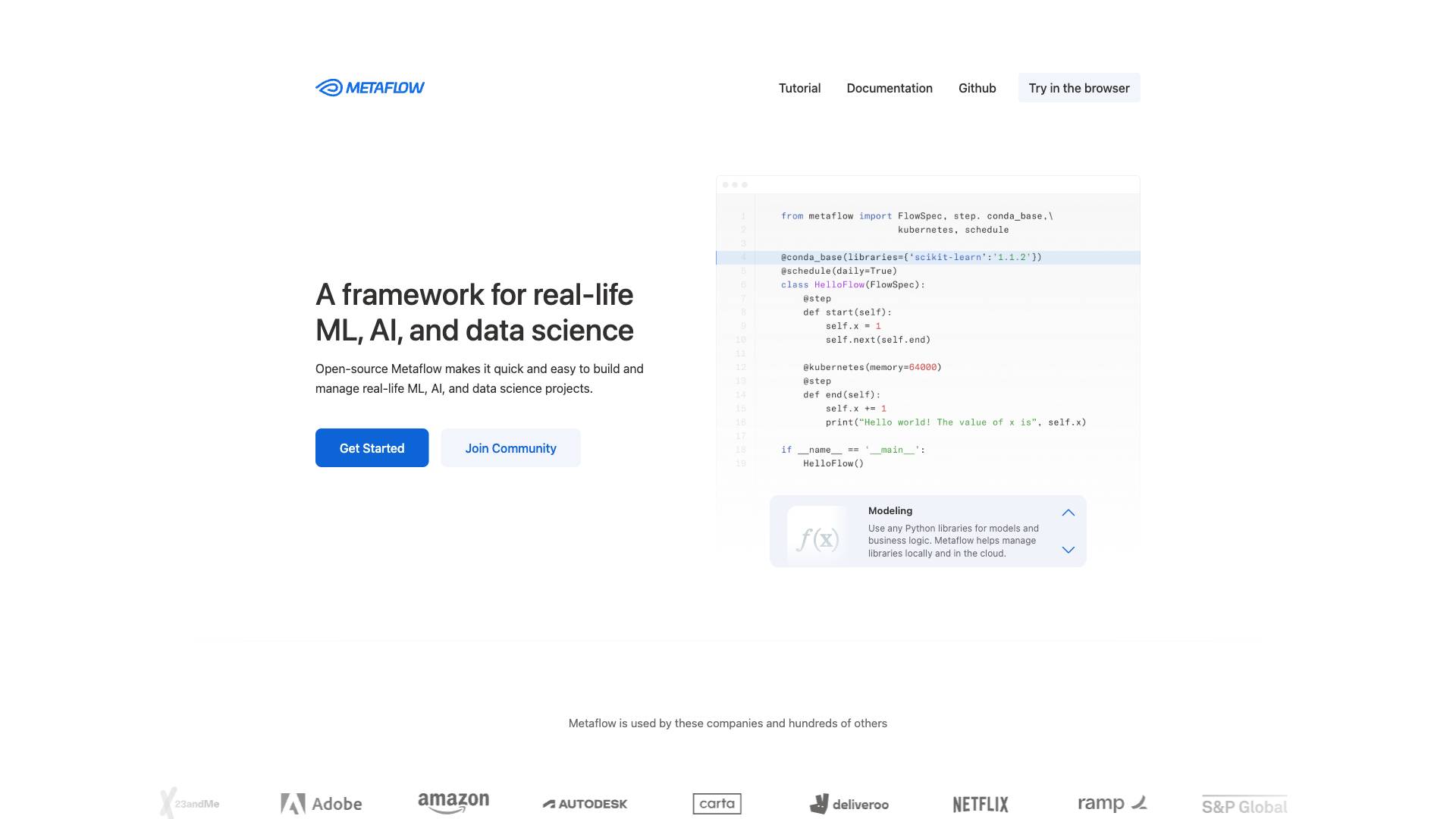
Tags
Useful for
- 1.What is Metaflow?
- 2.Features
- 2.1.1. Modeling
- 2.2.2. Deployment
- 2.3.3. Versioning
- 2.4.4. Orchestration
- 2.5.5. Compute
- 2.6.6. Data Access
- 2.7.7. Human-Centric Design
- 2.8.8. Cloud Compatibility
- 2.9.9. Recent Updates
- 3.Use Cases
- 3.1.1. Machine Learning Model Development
- 3.2.2. Data Pipeline Management
- 3.3.3. AI Research and Experimentation
- 3.4.4. Productionizing ML Models
- 3.5.5. Collaboration Among Data Teams
- 4.Pricing
- 5.Comparison with Other Tools
- 5.1.1. Human-Centric Design
- 5.2.2. Seamless Local and Cloud Integration
- 5.3.3. Automatic Versioning
- 5.4.4. Robust Community Support
- 5.5.5. Multi-Cloud Compatibility
- 6.FAQ
- 6.1.Q1: What programming languages does Metaflow support?
- 6.2.Q2: Is Metaflow suitable for beginners?
- 6.3.Q3: Can I use Metaflow with existing ML libraries?
- 6.4.Q4: How does Metaflow handle data security?
- 6.5.Q5: Is there a community for Metaflow users?
- 6.6.Q6: How often is Metaflow updated?
What is Metaflow?
Metaflow is an open-source framework designed to simplify the process of building and managing real-life machine learning (ML), artificial intelligence (AI), and data science projects. Originally developed at Netflix, Metaflow was created to meet the needs of developers and data scientists working on complex ML and AI applications. Since its open-sourcing in 2019, Metaflow has been adopted by numerous organizations across various industries, making it a popular choice for teams looking to streamline their data science workflows.
Metaflow aims to provide a human-centric approach to data science, enabling users to focus on their models and business logic rather than the underlying infrastructure. With its straightforward design and powerful features, Metaflow empowers data scientists to efficiently manage their workflows, from experimentation to production deployment.
Features
Metaflow is packed with features that cater to the needs of data scientists and ML engineers. Here are some of the key features that make Metaflow a compelling choice for managing data science projects:
1. Modeling
- Flexible Model Integration: Metaflow allows users to leverage any Python libraries for modeling and business logic, making it easy to integrate existing code and tools.
- Local and Cloud Management: It helps manage libraries both locally and in the cloud, ensuring that users can work seamlessly in their preferred environments.
2. Deployment
- Single Command Deployment: Users can deploy workflows to production with a single command, simplifying the transition from development to production.
- Seamless Integration: Metaflow integrates with surrounding systems, making it easier to connect various components of the data science pipeline.
3. Versioning
- Automatic Tracking: Metaflow automatically tracks and stores variables within the flow, facilitating easy experiment tracking and debugging.
- Easy Experimentation: This feature allows data scientists to experiment with different models and approaches without losing track of their work.
4. Orchestration
- Robust Workflow Creation: Users can create complex workflows using plain Python, which helps maintain code readability and simplicity.
- Local Development and Debugging: Metaflow supports local development and debugging, allowing users to test their workflows before deploying them to production.
5. Compute
- Cloud Scalability: Metaflow enables users to leverage cloud resources to execute functions at scale, utilizing GPUs, multiple cores, and large amounts of memory as needed.
- Efficient Resource Management: The framework organizes work for easy collaboration and efficient resource utilization.
6. Data Access
- Seamless Data Handling: Metaflow facilitates data access from data warehouses and manages data flow across steps while versioning everything along the way.
- Support for Various Data Formats: Users can work with different data types and formats, ensuring flexibility in data handling.
7. Human-Centric Design
- Built for Data Scientists: Metaflow is designed specifically for ML/AI engineers and data scientists, prioritizing their needs and workflows.
- Community Support: Users can join a community of fellow data scientists, sharing knowledge, resources, and experiences.
8. Cloud Compatibility
- Multi-Cloud Support: Metaflow can be deployed on various cloud platforms, including AWS, Azure, and Google Cloud, as well as on-premise Kubernetes clusters.
- Bring Your Own Cloud: Users can easily start on a laptop and scale to their cloud account when ready, ensuring flexibility in deployment.
9. Recent Updates
Metaflow continuously evolves, with recent updates enhancing its functionality:
- Checkpointing: Users can checkpoint long-running model training and other tasks using the new @checkpoint decorator.
- Configurable Flows: The new Config object allows for greater flexibility in configuring flows.
- Programmatic Deployment: New APIs enable users to run and deploy Metaflow in notebooks and scripts.
- Real-Time Updates: Build observable ML/AI systems with cards that update in real-time.
Use Cases
Metaflow is versatile and can be applied to various data science and machine learning tasks. Here are some common use cases:
1. Machine Learning Model Development
Data scientists can use Metaflow to develop, test, and deploy machine learning models efficiently. The framework's versioning and orchestration features allow for easy experimentation and tracking of model performance.
2. Data Pipeline Management
Metaflow is suitable for managing data pipelines, enabling users to create workflows that handle data ingestion, transformation, and storage seamlessly. The ability to version data and track changes enhances the reliability of data pipelines.
3. AI Research and Experimentation
Researchers can leverage Metaflow to conduct experiments with different algorithms and approaches, tracking results and performance metrics throughout the process. This is particularly useful in the fast-paced field of AI research.
4. Productionizing ML Models
Metaflow simplifies the transition from experimentation to production, allowing teams to deploy models confidently with minimal changes to the code. This is crucial for organizations looking to integrate ML models into their operations.
5. Collaboration Among Data Teams
Metaflow's design fosters collaboration among data science teams, enabling multiple users to work on the same project without conflicts. The framework's organization of workflows and data helps streamline teamwork.
Pricing
Metaflow is an open-source framework, which means it is free to use. However, organizations may incur costs associated with the cloud infrastructure they choose to deploy Metaflow on, such as AWS, Azure, or Google Cloud. These costs will vary based on the resources utilized, such as compute instances, storage, and data transfer.
For organizations looking for enterprise support or additional features, there may be options for commercial offerings or support services. It’s advisable for teams to evaluate their specific needs and consider any associated costs when planning their deployment.
Comparison with Other Tools
When comparing Metaflow with other data science frameworks and tools, several unique selling points emerge:
1. Human-Centric Design
Metaflow is specifically designed for data scientists and ML engineers, focusing on their workflows and challenges. This contrasts with many other tools that may prioritize machine-centric approaches.
2. Seamless Local and Cloud Integration
While many frameworks require significant changes to transition from local development to cloud deployment, Metaflow allows users to develop and debug locally and deploy to production without changes. This flexibility is a significant advantage.
3. Automatic Versioning
The automatic tracking and versioning of variables within workflows set Metaflow apart from other tools that may require manual intervention for tracking experiments and results.
4. Robust Community Support
Metaflow has a growing community of users who contribute to its development and share knowledge, making it easier for new users to get started and find support.
5. Multi-Cloud Compatibility
With support for various cloud providers and the ability to bring your own cloud, Metaflow offers flexibility that may not be available in other frameworks that are tied to specific cloud environments.
FAQ
Q1: What programming languages does Metaflow support?
Metaflow primarily supports Python, making it accessible for data scientists familiar with this popular programming language.
Q2: Is Metaflow suitable for beginners?
Yes, Metaflow is designed to be user-friendly, making it suitable for both beginners and experienced data scientists. Its straightforward syntax and comprehensive documentation help newcomers get started quickly.
Q3: Can I use Metaflow with existing ML libraries?
Absolutely! Metaflow is designed to work with any Python libraries, allowing users to integrate their preferred ML libraries and tools into their workflows seamlessly.
Q4: How does Metaflow handle data security?
Metaflow integrates with existing infrastructure, security, and data governance policies, ensuring that data security is maintained throughout the workflow process.
Q5: Is there a community for Metaflow users?
Yes, there is an active community of Metaflow users who share resources, knowledge, and support. Users can join community forums and discussions to connect with others in the field.
Q6: How often is Metaflow updated?
Metaflow is continuously evolving, with regular updates that introduce new features and improvements. Users can stay informed about the latest releases through the official documentation and community channels.
In conclusion, Metaflow stands out as a powerful, user-friendly framework for managing ML, AI, and data science projects. Its robust features, flexibility, and focus on collaboration make it an excellent choice for data scientists and engineers looking to streamline their workflows and maximize productivity.
Ready to try it out?
Go to Metaflow