Ludwig
Ludwig is an AI-powered tool designed for machine learning model development, though its website currently cannot be accessed.
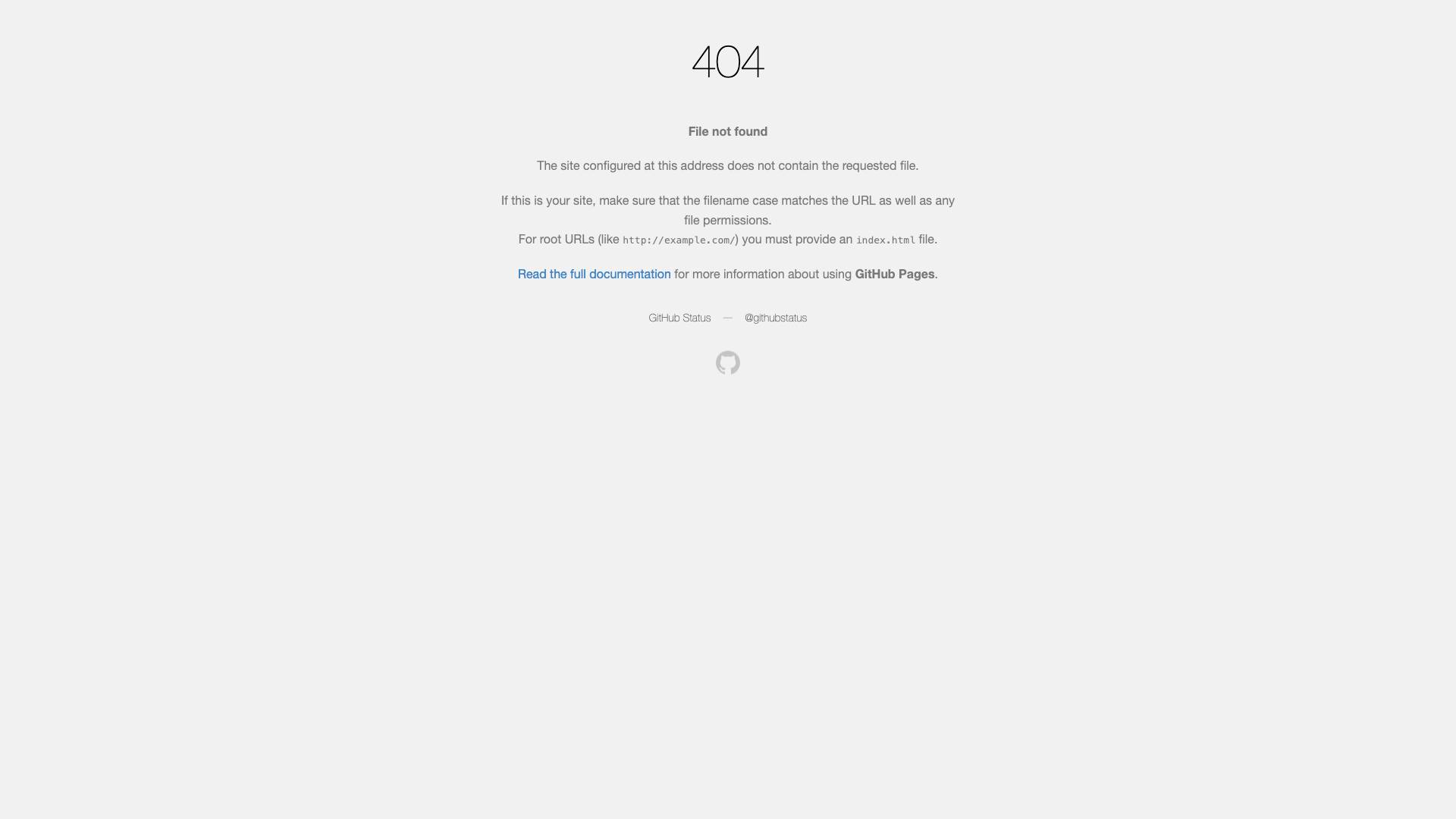
Tags
Useful for
- 1.What is Ludwig?
- 2.Features
- 2.1.1. Declarative Model Definition
- 2.2.2. Support for Multiple Data Types
- 2.3.3. Pre-trained Models
- 2.4.4. Automatic Feature Engineering
- 2.5.5. Visualization Tools
- 2.6.6. Multi-Backend Support
- 2.7.7. Model Interpretability
- 2.8.8. Easy Integration
- 2.9.9. Community and Documentation
- 3.Use Cases
- 3.1.1. Text Classification
- 3.2.2. Image Recognition
- 3.3.3. Time Series Forecasting
- 3.4.4. Predictive Maintenance
- 3.5.5. Healthcare Analytics
- 3.6.6. Recommendation Systems
- 4.Pricing
- 5.Comparison with Other Tools
- 5.1.1. Ludwig vs. TensorFlow/Keras
- 5.2.2. Ludwig vs. Scikit-learn
- 5.3.3. Ludwig vs. AutoML Tools
- 5.4.4. Ludwig vs. PyTorch
- 6.FAQ
- 6.1.1. Is Ludwig suitable for beginners?
- 6.2.2. Can Ludwig handle large datasets?
- 6.3.3. What types of data can I use with Ludwig?
- 6.4.4. Is Ludwig open-source?
- 6.5.5. How can I get support for Ludwig?
- 6.6.6. Can I integrate Ludwig with other tools?
What is Ludwig?
Ludwig is an innovative tool designed to simplify the process of building and deploying machine learning models. It is an open-source framework that allows users to create models without requiring extensive programming knowledge. Ludwig leverages a user-friendly interface and a declarative approach to model training, enabling both beginners and experienced data scientists to harness the power of machine learning efficiently. With Ludwig, users can easily specify their data and desired model architecture, and the tool handles the rest, making it an ideal choice for various applications in data analysis, predictive modeling, and more.
Features
Ludwig boasts a range of features that make it a powerful yet accessible tool for machine learning practitioners. Here are some of its key features:
1. Declarative Model Definition
Ludwig allows users to define models using a simple YAML configuration file. This declarative approach makes it easy to specify input and output features, model parameters, and training settings without diving into complex code.
2. Support for Multiple Data Types
Ludwig supports various data types, including text, images, time series, and structured data. This versatility enables users to apply machine learning techniques to a wide array of datasets.
3. Pre-trained Models
Ludwig comes with a selection of pre-trained models that users can leverage for their tasks. This feature significantly reduces the time and effort needed to get started, as users can fine-tune existing models instead of building them from scratch.
4. Automatic Feature Engineering
Ludwig automates the feature engineering process, which is often one of the most time-consuming aspects of machine learning. It can automatically derive relevant features from raw data, helping users to focus on higher-level tasks.
5. Visualization Tools
Ludwig provides built-in visualization tools to help users understand their data and model performance. Users can visualize training metrics, model predictions, and feature importance, making it easier to interpret results and make informed decisions.
6. Multi-Backend Support
Ludwig supports multiple backends, including TensorFlow and PyTorch, allowing users to choose their preferred deep learning framework. This flexibility enables users to leverage the strengths of different frameworks based on their specific needs.
7. Model Interpretability
Ludwig offers tools for model interpretability, helping users understand how their models make predictions. This feature is particularly valuable in fields where understanding model decisions is critical, such as healthcare and finance.
8. Easy Integration
Ludwig can be easily integrated into existing workflows and systems. It supports various input formats, including CSV and JSON, and can output results in multiple formats, making it a versatile tool for data scientists.
9. Community and Documentation
As an open-source tool, Ludwig benefits from a vibrant community of users and contributors. Comprehensive documentation is available to help users get started and troubleshoot any issues they may encounter.
Use Cases
Ludwig's flexibility and ease of use make it suitable for a wide range of use cases across different industries. Here are some prominent examples:
1. Text Classification
Ludwig can be used to build models for text classification tasks, such as sentiment analysis, spam detection, and topic categorization. Users can easily input text data and train models to categorize or classify the text based on predefined labels.
2. Image Recognition
With its support for image data, Ludwig is well-suited for image recognition tasks. Users can train models to identify objects, classify images, or even generate captions for images, making it a valuable tool for computer vision applications.
3. Time Series Forecasting
Ludwig can handle time series data, allowing users to build models for forecasting tasks. This capability is useful in industries such as finance, retail, and supply chain management, where predicting future trends is critical.
4. Predictive Maintenance
In manufacturing and industrial settings, Ludwig can be used to predict equipment failures and maintenance needs. By analyzing historical data, users can build models that alert them to potential issues before they become costly problems.
5. Healthcare Analytics
Ludwig's interpretability features make it an excellent choice for healthcare analytics. Users can build models to predict patient outcomes, analyze treatment efficacy, and identify risk factors, all while maintaining transparency in model decision-making.
6. Recommendation Systems
Ludwig can also be employed to create recommendation systems that suggest products, content, or services based on user preferences and behaviors. This application is particularly valuable in e-commerce and entertainment industries.
Pricing
Ludwig is an open-source tool, which means that it is available for free to users. There are no licensing fees or subscription costs associated with using Ludwig. Users can download and install the software from its repository and start building machine learning models without any financial investment.
However, while Ludwig itself is free, users may incur costs related to the infrastructure needed to run their models, such as cloud computing resources or hardware for local installations. Additionally, organizations may choose to invest in training or consulting services to maximize their use of Ludwig, but these are optional and not required to use the tool.
Comparison with Other Tools
When evaluating Ludwig, it's essential to compare it with other machine learning tools and frameworks. Here are some comparisons with popular alternatives:
1. Ludwig vs. TensorFlow/Keras
While TensorFlow and Keras are powerful frameworks for building deep learning models, they often require a more extensive understanding of programming and model architecture. Ludwig simplifies this process with its declarative approach, making it more accessible for non-programmers.
2. Ludwig vs. Scikit-learn
Scikit-learn is a popular library for traditional machine learning tasks, but it may not provide the same level of support for deep learning as Ludwig. Ludwig excels in handling complex data types and architectures that require deep learning techniques, while Scikit-learn is better suited for simpler models.
3. Ludwig vs. AutoML Tools
AutoML tools automate the machine learning process, but they can sometimes be a black box, making it challenging to understand how models are built. Ludwig strikes a balance between automation and interpretability, allowing users to define models while still maintaining control over their configurations.
4. Ludwig vs. PyTorch
PyTorch is a powerful deep learning framework, but it requires users to write code for model training and evaluation. Ludwig abstracts much of this complexity, allowing users to focus on data and model definitions rather than coding.
FAQ
1. Is Ludwig suitable for beginners?
Yes, Ludwig is designed to be user-friendly and accessible for beginners. Its declarative approach allows users to define models without extensive programming knowledge, making it an excellent choice for those new to machine learning.
2. Can Ludwig handle large datasets?
Ludwig is capable of handling large datasets, but performance may vary depending on the computational resources available. Users should ensure they have adequate hardware or cloud resources to manage large-scale training tasks.
3. What types of data can I use with Ludwig?
Ludwig supports various data types, including text, images, time series, and structured data. This versatility allows users to apply machine learning techniques to a wide range of datasets.
4. Is Ludwig open-source?
Yes, Ludwig is an open-source tool, and it is available for free. Users can download and use it without any licensing fees.
5. How can I get support for Ludwig?
Ludwig has a vibrant community of users and contributors. Users can access comprehensive documentation, participate in community forums, and seek help from other users to resolve any issues they may encounter.
6. Can I integrate Ludwig with other tools?
Yes, Ludwig can be easily integrated into existing workflows and systems. It supports various input and output formats, making it a versatile tool for data scientists.
In conclusion, Ludwig is a powerful and accessible tool for building machine learning models, offering a range of features that cater to both beginners and experienced practitioners. Its unique selling points, such as declarative model definitions, support for multiple data types, and automatic feature engineering, make it a valuable asset in the machine learning landscape. Whether you are working on text classification, image recognition, or predictive analytics, Ludwig provides the tools you need to succeed.
Ready to try it out?
Go to Ludwig