Keras Tool
Keras is a flexible, user-friendly API for building and deploying deep learning models across multiple frameworks, enhancing productivity and collaboration.
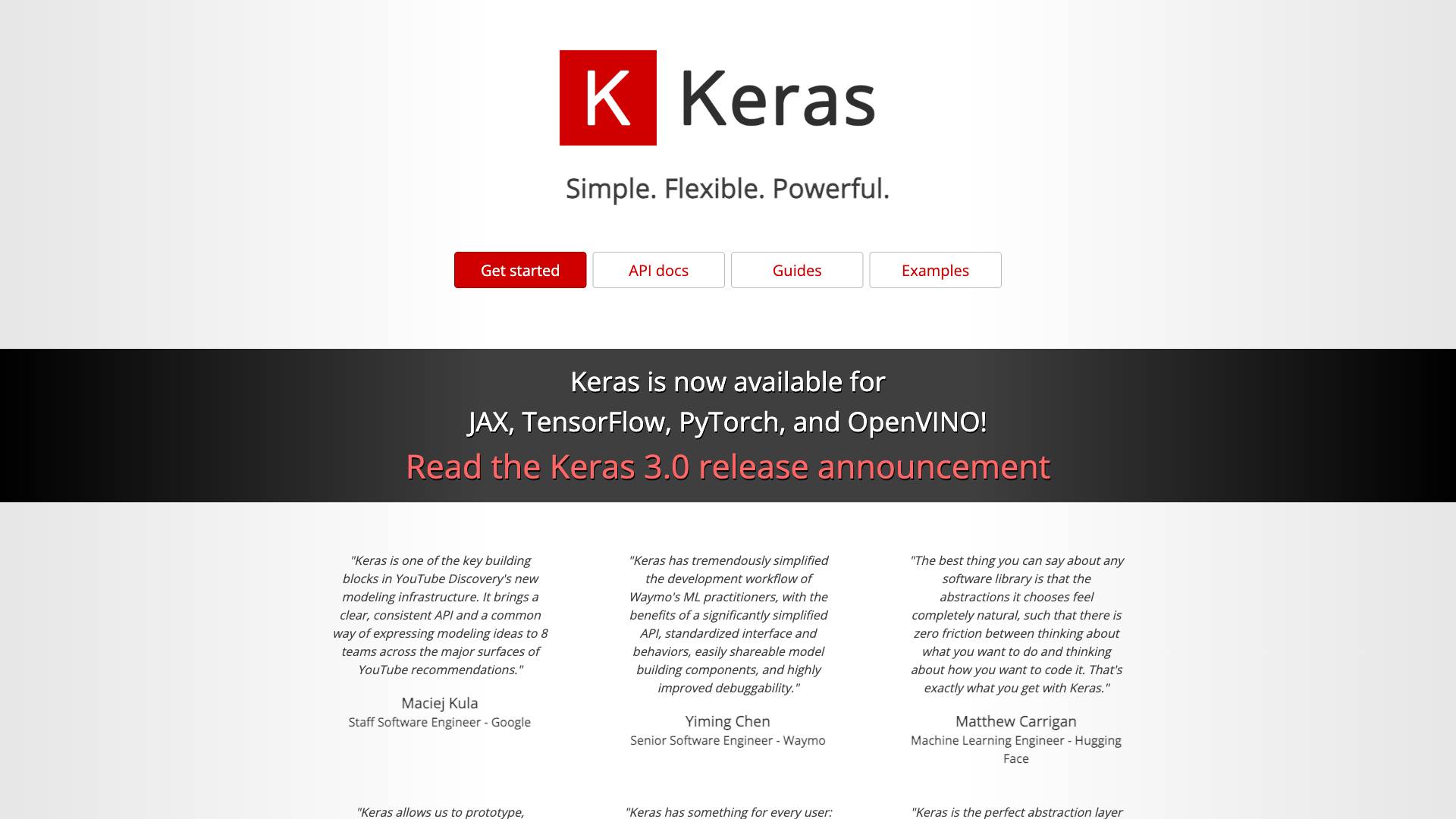
Tags
Useful for
- 1.What is Keras Tool?
- 2.Features
- 2.1.1. User-Friendly API
- 2.2.2. Flexibility and Customizability
- 2.3.3. Support for Multiple Backends
- 2.4.4. Efficient Model Deployment
- 2.5.5. High Performance
- 2.6.6. Comprehensive Documentation
- 2.7.7. State-of-the-Art Research Support
- 2.8.8. Debugging and Maintainability
- 3.Use Cases
- 3.1.1. Image Classification
- 3.2.2. Natural Language Processing (NLP)
- 3.3.3. Time Series Forecasting
- 3.4.4. Reinforcement Learning
- 3.5.5. Medical Image Analysis
- 3.6.6. Autonomous Vehicles
- 3.7.7. Recommendation Systems
- 4.Pricing
- 5.Comparison with Other Tools
- 5.1.Keras vs. TensorFlow
- 5.2.Keras vs. PyTorch
- 5.3.Keras vs. Scikit-learn
- 6.FAQ
- 6.1.What programming languages does Keras support?
- 6.2.Can I use Keras for production applications?
- 6.3.Is Keras suitable for beginners?
- 6.4.What types of models can I build with Keras?
- 6.5.How does Keras handle large datasets?
- 6.6.Can I integrate Keras with other libraries?
What is Keras Tool?
Keras is an open-source deep learning framework that provides a user-friendly API for building and training neural networks. Originally developed as a high-level interface for TensorFlow, Keras has evolved into a versatile tool that now supports multiple backends including JAX, PyTorch, and OpenVINO. Its primary goal is to simplify the process of creating deep learning models, making it accessible to both beginners and experienced practitioners in the field of machine learning.
Keras is designed to facilitate rapid prototyping and experimentation, enabling users to quickly iterate on their ideas and deploy models across various platforms. With its consistent and intuitive API, Keras has gained widespread adoption among developers, researchers, and organizations, serving as a foundational building block for various machine learning applications.
Features
Keras offers a wide range of features that cater to the needs of different users, from academic researchers to industry professionals. Here are some of the key features that make Keras a powerful tool for deep learning:
1. User-Friendly API
Keras is designed with a human-centric approach, emphasizing ease of use and readability. The API minimizes cognitive load by providing consistent and simple interfaces, making it easier for users to focus on their modeling ideas rather than the underlying complexities.
2. Flexibility and Customizability
Keras allows users to create custom models and layers, enabling researchers to implement novel architectures and experiment with different modeling approaches. This flexibility is crucial for academic research where innovative ideas often require tailored implementations.
3. Support for Multiple Backends
One of Keras's standout features is its compatibility with multiple deep learning frameworks, including JAX, TensorFlow, and PyTorch. This framework-agnostic approach allows users to leverage the strengths of different ecosystems and move models seamlessly across platforms.
4. Efficient Model Deployment
Keras simplifies the deployment process by integrating with various serving components from the TensorFlow and PyTorch ecosystems. Users can easily deploy their models across different environments, such as servers, mobile devices, browsers, and embedded systems.
5. High Performance
Keras leverages the power of XLA compilation with JAX and TensorFlow, resulting in faster model execution. This performance enhancement is particularly beneficial for training complex models on large datasets or utilizing distributed computing resources.
6. Comprehensive Documentation
Keras prioritizes developer experience by providing extensive documentation, guides, and examples. This wealth of resources helps users quickly learn how to use the framework effectively and troubleshoot issues as they arise.
7. State-of-the-Art Research Support
Keras is widely used in cutting-edge research across various scientific organizations, including CERN, NASA, and the NIH. It offers the low-level flexibility needed to implement advanced research ideas while providing high-level convenience features to accelerate experimentation.
8. Debugging and Maintainability
Keras emphasizes debugging speed, code elegance, and maintainability. The framework's design encourages modular code, making it easier for teams to collaborate, share components, and iterate on their models without introducing unnecessary complexity.
Use Cases
Keras is a versatile tool that can be applied across various domains and industries. Here are some common use cases that highlight its capabilities:
1. Image Classification
Keras is widely used for image classification tasks, such as identifying objects in photographs or categorizing images into predefined classes. Its convolutional neural network (CNN) architectures make it particularly effective for these applications.
2. Natural Language Processing (NLP)
Keras can be utilized for various NLP tasks, including sentiment analysis, text classification, and language translation. Its support for recurrent neural networks (RNNs) and transformer architectures enables users to build models that understand and generate human language.
3. Time Series Forecasting
Keras is suitable for time series forecasting applications, where users need to predict future values based on historical data. The framework's ability to handle sequential data makes it a popular choice for this type of analysis.
4. Reinforcement Learning
Keras can be employed in reinforcement learning scenarios, where agents learn to make decisions based on interactions with their environment. Its flexibility allows researchers to implement custom reward functions and learning algorithms.
5. Medical Image Analysis
In the healthcare sector, Keras is used for tasks such as medical image analysis, disease detection, and predictive modeling. Its ability to process high-dimensional data makes it valuable for developing diagnostic tools and treatment recommendations.
6. Autonomous Vehicles
Keras plays a crucial role in the development of machine learning models for autonomous vehicles. It is used for tasks such as object detection, path planning, and sensor fusion, enabling vehicles to navigate safely and efficiently.
7. Recommendation Systems
Keras is utilized in building recommendation systems that provide personalized content to users. Its flexibility allows developers to experiment with different algorithms and architectures to optimize user engagement.
Pricing
Keras is an open-source framework, which means it is free to use and distribute. Users can download and install Keras without any licensing fees. However, while Keras itself is free, users may incur costs associated with the infrastructure needed to run their models, such as cloud computing resources, GPU instances, or other hardware.
For organizations that require additional support, training, or consulting services, there may be costs associated with hiring experts or purchasing enterprise-level solutions. However, the core Keras framework remains accessible to everyone at no cost.
Comparison with Other Tools
When comparing Keras with other deep learning frameworks, several unique selling points and distinctions emerge:
Keras vs. TensorFlow
- Ease of Use: Keras is built on top of TensorFlow and provides a more user-friendly interface, making it easier for newcomers to get started with deep learning.
- Flexibility: While TensorFlow offers extensive capabilities for building complex models, Keras simplifies the process of model creation and experimentation, allowing for rapid prototyping.
Keras vs. PyTorch
- API Design: Keras emphasizes a high-level API that abstracts away many low-level details, while PyTorch provides more flexibility and control over model architecture.
- Deployment: Keras offers seamless integration with TensorFlow's serving components, making it easier to deploy models in production environments compared to PyTorch.
Keras vs. Scikit-learn
- Deep Learning Focus: Keras is specifically designed for deep learning tasks, while Scikit-learn is more suited for traditional machine learning algorithms. Keras excels in handling large datasets and complex models, whereas Scikit-learn is ideal for simpler tasks and data preprocessing.
- Model Complexity: Keras supports deep neural networks with multiple layers and advanced architectures, whereas Scikit-learn is limited to shallower models.
FAQ
What programming languages does Keras support?
Keras is primarily designed for Python, which is the most commonly used language in the machine learning community. However, it can be integrated with other languages through various APIs or interfaces.
Can I use Keras for production applications?
Yes, Keras is widely used in production environments. Its integration with TensorFlow and other serving components makes it easy to deploy models across different platforms, including servers, mobile devices, and embedded systems.
Is Keras suitable for beginners?
Absolutely! Keras is designed to be user-friendly and accessible, making it an excellent choice for beginners who are new to deep learning. The extensive documentation and community support further enhance the learning experience.
What types of models can I build with Keras?
Keras supports a wide range of model types, including feedforward neural networks, convolutional neural networks (CNNs), recurrent neural networks (RNNs), and more. Users can also create custom models and layers to suit their specific needs.
How does Keras handle large datasets?
Keras is capable of scaling to large datasets and can utilize distributed computing resources, such as GPU clusters or TPU pods, for training complex models. This scalability is essential for handling real-world machine learning tasks.
Can I integrate Keras with other libraries?
Yes, Keras can be easily integrated with other Python libraries and frameworks, such as NumPy, Pandas, and Matplotlib, allowing users to leverage the strengths of these tools for data manipulation, visualization, and analysis.
In conclusion, Keras is a powerful and flexible deep learning framework that caters to a wide range of users and applications. Its user-friendly design, extensive features, and support for multiple backends make it a top choice for developers, researchers, and organizations looking to harness the power of machine learning. Whether you're a beginner or an experienced practitioner, Keras provides the tools and resources needed to bring your deep learning projects to life.
Ready to try it out?
Go to Keras Tool