Jupyter Notebook
Jupyter Notebook is a web-based platform for creating and sharing interactive computational documents across multiple programming languages.
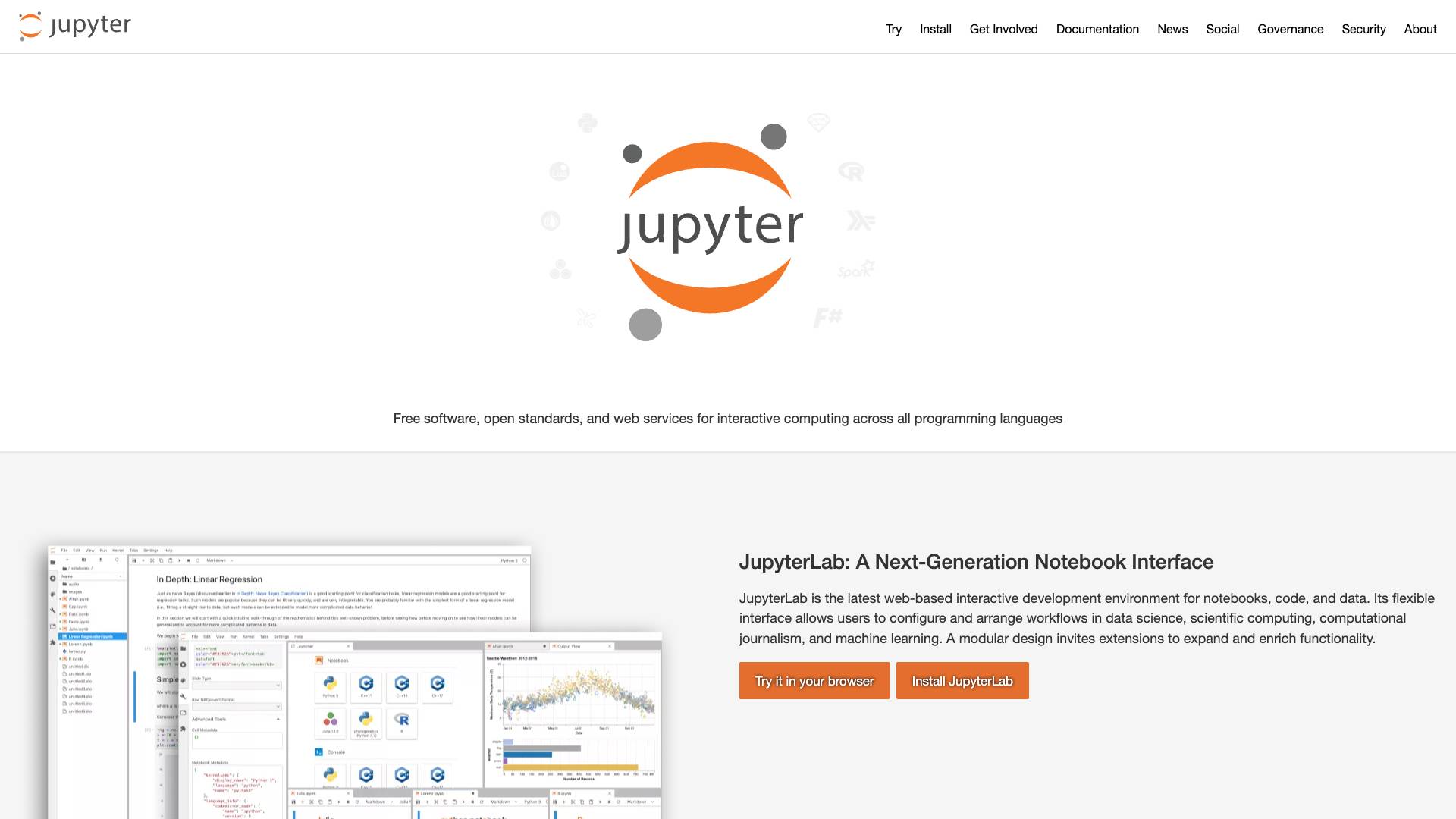
Tags
Useful for
- 1.What is Jupyter Notebook?
- 1.1.Features
- 1.1.1.1. Multi-language Support
- 1.1.2.2. Interactive Output
- 1.1.3.3. Document-Centric Interface
- 1.1.4.4. Shareability
- 1.1.5.5. Big Data Integration
- 1.1.6.6. Extensibility
- 1.1.7.7. Multi-User Support
- 1.1.8.8. Pluggable Authentication
- 1.1.9.9. Container Friendly
- 1.1.10.10. Notebook Document Format
- 1.1.11.11. Interactive Computing Protocol
- 1.1.12.12. Kernel Support
- 1.2.Use Cases
- 1.2.1.1. Data Science
- 1.2.2.2. Machine Learning
- 1.2.3.3. Scientific Research
- 1.2.4.4. Education
- 1.2.5.5. Computational Journalism
- 1.2.6.6. Prototyping
- 1.2.7.7. Data Visualization
- 1.3.Pricing
- 1.4.Comparison with Other Tools
- 1.4.1.1. Vs. R Markdown
- 1.4.2.2. Vs. Google Colab
- 1.4.3.3. Vs. Spyder
- 1.4.4.4. Vs. MATLAB
- 1.4.5.5. Vs. Visual Studio Code
- 1.5.FAQ
- 1.5.1.1. What is the difference between Jupyter Notebook and JupyterLab?
- 1.5.2.2. Can I use Jupyter Notebook for production applications?
- 1.5.3.3. Is Jupyter Notebook suitable for collaborative work?
- 1.5.4.4. How do I install Jupyter Notebook?
- 1.5.5.5. What are the system requirements for running Jupyter Notebook?
- 1.5.6.6. Can I use Jupyter Notebook offline?
- 1.5.7.7. What is the role of kernels in Jupyter Notebook?
What is Jupyter Notebook?
Jupyter Notebook is an open-source web application that allows users to create and share documents that contain live code, equations, visualizations, and narrative text. It is part of the Jupyter ecosystem, which includes JupyterLab, a next-generation interface for notebooks, code, and data. Jupyter Notebook is widely used in data science, scientific computing, machine learning, and computational journalism, providing a user-friendly interface for both beginners and experienced programmers.
Features
Jupyter Notebook boasts a range of features that enhance its usability and functionality:
1. Multi-language Support
- Diverse Language Compatibility: Jupyter supports over 40 programming languages, including Python, R, Julia, and Scala, allowing users to work with the language they are most comfortable with or the one best suited for their project.
2. Interactive Output
- Rich Visualizations: Users can generate interactive outputs such as HTML, images, videos, LaTeX, and custom MIME types, making it easier to visualize data and results effectively.
3. Document-Centric Interface
- Streamlined Experience: The Jupyter Notebook provides a simple and intuitive document-centric experience, allowing users to create and share computational documents seamlessly.
4. Shareability
- Collaborative Features: Notebooks can be shared with others via email, Dropbox, GitHub, and the Jupyter Notebook Viewer, facilitating collaboration and knowledge sharing.
5. Big Data Integration
- Seamless Data Handling: Jupyter Notebook integrates with big data tools such as Apache Spark, enabling users to analyze large datasets using popular libraries like pandas, scikit-learn, ggplot2, and TensorFlow.
6. Extensibility
- Modular Design: The notebook's modular design allows for extensions that can enhance functionality, making it customizable to fit specific user needs.
7. Multi-User Support
- JupyterHub: A multi-user version of Jupyter Notebook is available for companies, classrooms, and research labs, allowing centralized management of users and resources.
8. Pluggable Authentication
- User Management: Jupyter Notebook supports various authentication methods, including PAM and OAuth, enabling organizations to integrate with their existing user management systems.
9. Container Friendly
- Deployment Flexibility: The notebook can be deployed using Docker and Kubernetes, allowing for scalability, isolated user processes, and simplified software installation.
10. Notebook Document Format
- Open Document Format: Jupyter Notebooks utilize an open document format based on JSON, which contains a complete record of user sessions, including code, narrative text, equations, and rich output.
11. Interactive Computing Protocol
- Kernel Communication: The notebook communicates with computational kernels using the Interactive Computing Protocol, facilitating seamless interaction between the user and the code execution environment.
12. Kernel Support
- Language-Specific Kernels: Each kernel is a process that runs interactive code in a specific programming language, responding to user requests for output, tab completion, and introspection.
Use Cases
Jupyter Notebook is versatile and can be applied in various domains:
1. Data Science
- Exploratory Data Analysis: Data scientists use Jupyter Notebook to conduct exploratory data analysis, visualize data, and build predictive models.
2. Machine Learning
- Model Development and Evaluation: The notebook provides an interactive environment for developing machine learning models, training algorithms, and evaluating performance.
3. Scientific Research
- Reproducible Research: Researchers can document their experiments, methodologies, and findings in a reproducible format, facilitating collaboration and peer review.
4. Education
- Teaching and Learning: Educators leverage Jupyter Notebook to teach programming, data analysis, and scientific computing concepts in an interactive manner.
5. Computational Journalism
- Data-Driven Storytelling: Journalists can analyze data, visualize results, and share insights through notebooks, enhancing their storytelling with data-driven evidence.
6. Prototyping
- Rapid Development: Developers can quickly prototype applications and algorithms using the interactive features of Jupyter Notebook.
7. Data Visualization
- Creating Visual Representations: Users can create visualizations to present data insights clearly and effectively, using libraries such as Matplotlib and Seaborn.
Pricing
Jupyter Notebook itself is open-source and free to use. However, organizations may incur costs when deploying JupyterHub for multi-user environments, especially if they choose to host it on cloud services or require additional features and support. The pricing for such deployments typically depends on the infrastructure used (e.g., cloud services, on-premises servers) and any additional resources needed for maintenance and scalability.
Comparison with Other Tools
When comparing Jupyter Notebook with other data analysis and computational tools, several unique selling points emerge:
1. Vs. R Markdown
- Interactivity: Unlike R Markdown, which is primarily focused on static reports, Jupyter Notebook provides an interactive environment where users can run code and visualize results in real-time.
2. Vs. Google Colab
- Local Environment: Jupyter Notebook can be run locally on a user’s machine, providing better control over the environment and dependencies compared to Google Colab, which is cloud-based.
3. Vs. Spyder
- Document-Centric Approach: Jupyter Notebook's document-centric interface allows for a more narrative-driven approach to data analysis, unlike Spyder, which is more focused on code editing and debugging.
4. Vs. MATLAB
- Open Source: Jupyter Notebook is open-source, making it accessible to a broader audience compared to MATLAB, which requires a paid license. Additionally, Jupyter supports multiple languages, while MATLAB is primarily focused on its proprietary language.
5. Vs. Visual Studio Code
- Focus on Data Science: While Visual Studio Code is a versatile code editor, Jupyter Notebook is specifically designed for data science and computational tasks, providing features tailored to these workflows.
FAQ
1. What is the difference between Jupyter Notebook and JupyterLab?
JupyterLab is the next-generation interface for Jupyter notebooks, offering a more flexible and modular design compared to the classic Jupyter Notebook interface. It allows users to arrange multiple documents and tools in a single workspace, enhancing productivity.
2. Can I use Jupyter Notebook for production applications?
While Jupyter Notebook is primarily designed for interactive computing and exploratory data analysis, it can be used in production environments when integrated with other tools and frameworks. However, it is not typically recommended for deploying production-level applications directly.
3. Is Jupyter Notebook suitable for collaborative work?
Yes, Jupyter Notebook supports collaborative work through features like sharing via GitHub, Dropbox, and email. Additionally, JupyterHub allows for multi-user access, making it ideal for classrooms and research labs.
4. How do I install Jupyter Notebook?
Jupyter Notebook can be easily installed using package managers like pip or conda. Users can also install it as part of the Anaconda distribution, which includes many other useful data science packages.
5. What are the system requirements for running Jupyter Notebook?
Jupyter Notebook can run on any system that supports Python. It can be installed on Windows, macOS, and Linux. The specific requirements depend on the libraries and tools used within the notebooks.
6. Can I use Jupyter Notebook offline?
Yes, Jupyter Notebook can be run locally on your machine, allowing you to work offline. However, some features that rely on internet access, such as sharing notebooks via external platforms, may not be available.
7. What is the role of kernels in Jupyter Notebook?
Kernels are processes that run interactive code in a specific programming language. They execute the code written in the notebook and return the output to the user, enabling real-time interaction with the code.
In conclusion, Jupyter Notebook is a powerful tool that provides an interactive computing environment suitable for various applications, from data analysis to education. Its rich features, multi-language support, and collaborative capabilities make it a go-to choice for data scientists, researchers, and educators alike.
Ready to try it out?
Go to Jupyter Notebook