InterpretML
InterpretML is an open-source toolkit that enhances model interpretability, enabling developers and stakeholders to understand, debug, and audit machine learning models.
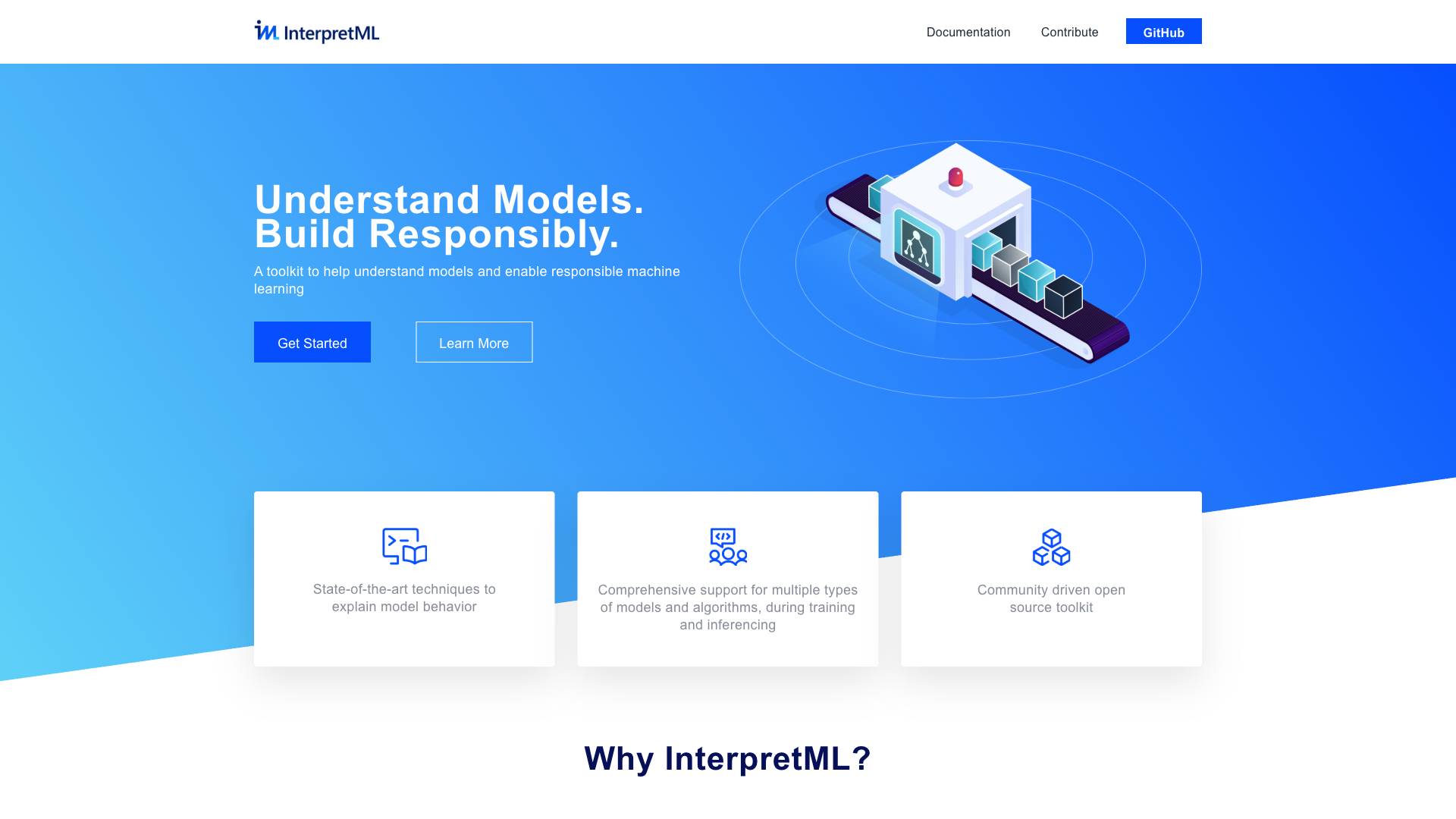
Tags
Useful for
- 1.What is InterpretML?
- 2.Features
- 2.1.1. State-of-the-Art Techniques
- 2.2.2. Comprehensive Model Support
- 2.2.1.Glass-Box Models
- 2.2.2.Black-Box Models
- 2.3.3. Global and Local Interpretability
- 2.4.4. What-If Analysis
- 2.5.5. Interactive Visualizations
- 2.6.6. Comprehensive Capabilities
- 2.7.7. Community-Driven Open Source
- 3.Use Cases
- 3.1.1. Data Scientists
- 3.2.2. Auditors
- 3.3.3. Business Leaders
- 3.4.4. Researchers
- 4.Pricing
- 5.Comparison with Other Tools
- 5.1.1. Comprehensive Support for Multiple Models
- 5.2.2. Unified API
- 5.3.3. Rich Visualizations
- 5.4.4. Community-Driven Development
- 5.5.5. Focus on Responsible Machine Learning
- 6.FAQ
- 6.1.1. What types of models does InterpretML support?
- 6.2.2. Is InterpretML free to use?
- 6.3.3. How can I contribute to InterpretML?
- 6.4.4. Can I use InterpretML for what-if analysis?
- 6.5.5. Who can benefit from using InterpretML?
- 6.6.6. How does InterpretML ensure model interpretability?
What is InterpretML?
InterpretML is an open-source toolkit designed to help users understand machine learning models and promote responsible machine learning practices. It provides state-of-the-art techniques for model interpretability, allowing developers, data scientists, and business stakeholders to gain insights into model behavior, debug models, explain predictions, and ensure compliance with regulatory requirements. By offering a unified API and rich visualizations, InterpretML makes it easier for users to access and implement various interpretability techniques across a wide range of machine learning models.
Features
InterpretML comes packed with features that enhance model interpretability and usability. Below are some of the key features that make InterpretML stand out:
1. State-of-the-Art Techniques
InterpretML incorporates the latest advancements in model interpretability, allowing users to leverage cutting-edge techniques to explain model behavior effectively.
2. Comprehensive Model Support
The toolkit supports a variety of machine learning models, including both glass-box and black-box models. This flexibility ensures that users can apply InterpretML to a wide range of algorithms and scenarios.
Glass-Box Models
- Explainable Boosting Machines (EBM)
- Linear Models
- Decision Trees
Glass-box models are inherently interpretable due to their structure and provide lossless explanations that can be edited by domain experts.
Black-Box Models
While black-box models are typically less interpretable, InterpretML offers techniques to explain their predictions and behaviors.
3. Global and Local Interpretability
InterpretML provides tools for both global and local interpretability:
- Global Interpretability: Understand overall model behavior and identify top features influencing predictions through global feature importance.
- Local Interpretability: Explain individual predictions and analyze features contributing to specific outcomes using local feature importance.
4. What-If Analysis
With InterpretML, users can perform what-if analyses to see how changes to input features impact predictions. This feature is particularly useful for understanding model behavior and conducting sensitivity analyses.
5. Interactive Visualizations
The toolkit offers rich, interactive visualizations that help users explore model attributes such as performance, feature importance, and predictions. This visual approach makes it easier to communicate findings to stakeholders.
6. Comprehensive Capabilities
InterpretML allows users to:
- Explore model performance across different subsets of data.
- Compare multiple models simultaneously to identify the best-performing algorithms.
- Analyze dataset statistics and distributions to gain insights into the underlying data.
7. Community-Driven Open Source
InterpretML is a community-driven project, encouraging contributions from developers, researchers, and users. This collaborative approach ensures that the toolkit evolves with the latest interpretability techniques and user needs.
Use Cases
InterpretML is versatile and can be applied across various domains and use cases. Here are some of the primary use cases for the toolkit:
1. Data Scientists
Data scientists can leverage InterpretML to:
- Understand the intricacies of their machine learning models.
- Debug models to identify and rectify issues.
- Explain model predictions to non-technical stakeholders, enhancing transparency and trust.
2. Auditors
Auditors can use InterpretML to:
- Validate models before deployment, ensuring they meet performance and ethical standards.
- Audit models post-deployment to ensure compliance with regulatory requirements and maintain accountability.
3. Business Leaders
Business leaders can benefit from InterpretML by:
- Gaining insights into how models behave, which allows them to provide transparency about predictions to customers.
- Making informed decisions based on a thorough understanding of model outputs.
4. Researchers
Researchers can utilize InterpretML to:
- Easily integrate new interpretability techniques into their workflows.
- Compare different algorithms and interpretability methods to advance the field of machine learning.
Pricing
InterpretML is an open-source toolkit, which means it is available for free. Users can download and install the toolkit without any licensing fees. This makes it an attractive option for organizations looking to enhance model interpretability without incurring additional costs.
Comparison with Other Tools
When comparing InterpretML with other model interpretability tools, several unique selling points emerge:
1. Comprehensive Support for Multiple Models
Unlike some interpretability tools that focus on specific model types, InterpretML offers support for a wide variety of models, including both glass-box and black-box models. This versatility allows users to apply the toolkit across different machine learning frameworks.
2. Unified API
InterpretML provides a unified API that simplifies the process of accessing various interpretability techniques. This consistency makes it easier for users to experiment with different algorithms and visualization methods.
3. Rich Visualizations
The toolkit’s interactive visualizations enhance user experience by making it easier to explore model behavior and communicate findings to stakeholders. Many other tools may lack the same level of visual interactivity.
4. Community-Driven Development
InterpretML is developed and maintained by a community of contributors, which fosters innovation and ensures that the toolkit remains up-to-date with the latest research and techniques in model interpretability.
5. Focus on Responsible Machine Learning
InterpretML emphasizes responsible machine learning practices, making it a suitable choice for organizations that prioritize ethical AI development and compliance with regulatory standards.
FAQ
1. What types of models does InterpretML support?
InterpretML supports a variety of models, including glass-box models like Explainable Boosting Machines, linear models, and decision trees, as well as black-box models.
2. Is InterpretML free to use?
Yes, InterpretML is an open-source toolkit and is available for free. Users can download and install it without incurring any licensing fees.
3. How can I contribute to InterpretML?
InterpretML encourages contributions from the community. Users can provide feedback, suggest new algorithms, and share ideas to help evolve the toolkit.
4. Can I use InterpretML for what-if analysis?
Yes, InterpretML includes features for performing what-if analysis, allowing users to manipulate input features and observe the impact on model predictions.
5. Who can benefit from using InterpretML?
InterpretML can benefit a range of users, including data scientists, auditors, business leaders, and researchers, by providing insights into model behavior and enhancing transparency.
6. How does InterpretML ensure model interpretability?
InterpretML employs state-of-the-art techniques to explain model behavior, allowing users to gain a comprehensive understanding of their machine learning models and ensuring compliance with regulatory requirements.
In conclusion, InterpretML is a powerful and versatile toolkit that enhances model interpretability and promotes responsible machine learning practices. With its comprehensive features, diverse use cases, and commitment to community-driven development, it stands out as a valuable resource for anyone working with machine learning models.
Ready to try it out?
Go to InterpretML