DistilBERT
DistilBERT is a lightweight, efficient transformer model that delivers 95% of BERT's performance with 40% fewer parameters and 60% faster processing.
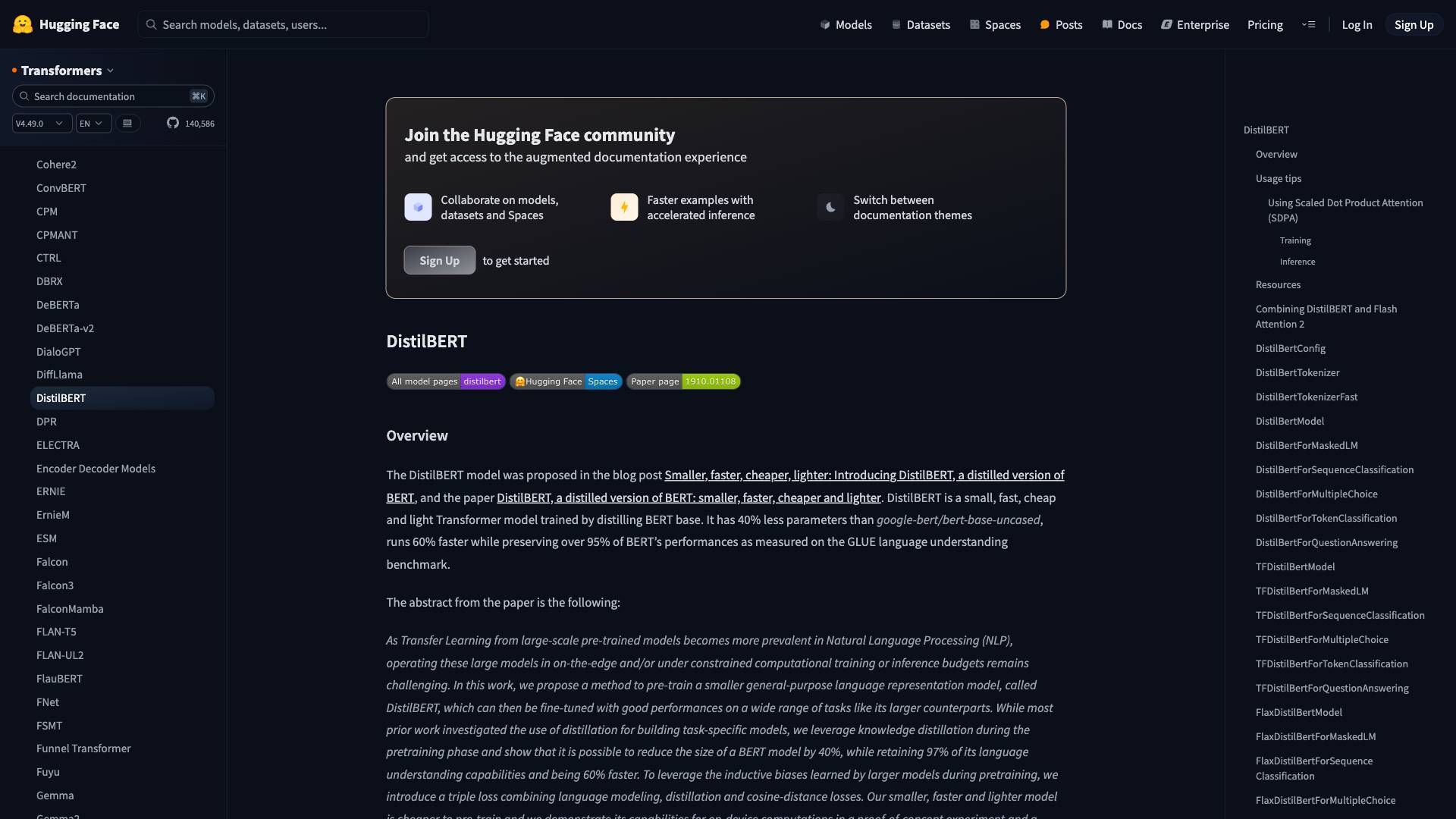
Tags
Useful for
- 1.What is DistilBERT?
- 2.Features
- 3.Use Cases
- 4.Pricing
- 5.Comparison with Other Tools
- 6.FAQ
- 6.1.What is knowledge distillation, and how does it apply to DistilBERT?
- 6.2.How does DistilBERT perform compared to BERT on various NLP tasks?
- 6.3.Can DistilBERT be fine-tuned for specific tasks?
- 6.4.Is DistilBERT suitable for deployment on mobile devices?
- 6.5.What are the hardware requirements for running DistilBERT?
- 6.6.How can I get started with DistilBERT?
- 6.7.Is there a community or support available for DistilBERT users?
What is DistilBERT?
DistilBERT is a state-of-the-art natural language processing (NLP) model that represents a distilled version of the well-known BERT (Bidirectional Encoder Representations from Transformers) model. Developed by Hugging Face, DistilBERT was introduced to address some of the limitations associated with using large-scale pre-trained models in real-world applications, particularly in scenarios with constrained computational resources. By leveraging knowledge distillation, DistilBERT significantly reduces the size and computational requirements of the original BERT model while retaining a high level of performance.
The primary goal of DistilBERT is to make powerful language representation models more accessible and efficient for various applications, enabling developers to deploy them in on-device and edge computing environments without sacrificing performance.
Features
DistilBERT comes with a range of features that set it apart from other NLP models:
-
Reduced Model Size: DistilBERT has 40% fewer parameters compared to the original BERT base model, making it significantly smaller and easier to deploy.
-
Faster Inference: The model runs 60% faster than BERT, which is crucial for applications that require real-time processing and quick responses.
-
High Performance: Despite its smaller size, DistilBERT retains over 95% of BERT's performance as measured on the GLUE language understanding benchmark, ensuring that users do not have to compromise on accuracy.
-
Knowledge Distillation: DistilBERT utilizes a unique training method that combines language modeling, distillation, and cosine-distance losses to effectively learn from the larger BERT model, allowing it to capture essential language features.
-
Compatibility: DistilBERT is compatible with the Hugging Face Transformers library, allowing users to easily integrate it into their existing workflows and applications.
-
Support for Multiple Tasks: The model is versatile and can be fine-tuned for various NLP tasks, including text classification, token classification, question answering, and more.
-
Optimized for On-Device Computation: DistilBERT is designed to be lightweight, making it suitable for deployment on devices with limited computational power, such as smartphones and IoT devices.
-
Efficient Training Techniques: The model supports efficient training methods, including distributed training and quantization, which further enhance its performance and scalability.
-
Ease of Use: The model is easy to use, with straightforward APIs for loading, training, and fine-tuning, making it accessible even for those new to NLP.
Use Cases
DistilBERT can be applied to a wide range of use cases across various industries. Some notable applications include:
-
Sentiment Analysis: Businesses can use DistilBERT to analyze customer feedback, reviews, and social media posts to gauge public sentiment towards their products or services.
-
Chatbots and Virtual Assistants: The model can power conversational agents that understand and respond to user queries in natural language, providing a more engaging user experience.
-
Text Classification: DistilBERT can be employed for categorizing documents, emails, or articles into predefined categories, streamlining information management and retrieval.
-
Named Entity Recognition (NER): Organizations can utilize DistilBERT to identify and extract relevant entities from text, such as names, dates, and locations, which is beneficial for data processing and analysis.
-
Question Answering Systems: The model can be integrated into systems that answer user questions based on a given context, enhancing customer support and information retrieval.
-
Multi-label Classification: DistilBERT can handle scenarios where multiple labels need to be assigned to a single input, making it useful for applications like topic detection in news articles.
-
Language Translation: While not its primary function, DistilBERT can assist in language translation tasks by providing contextual embeddings that improve translation quality.
-
Content Generation: The model can be used to generate coherent and contextually relevant text, which can be applied in creative writing, marketing, and content creation.
-
Educational Tools: DistilBERT can support educational applications by providing language understanding capabilities for tutoring systems, language learning apps, and more.
Pricing
DistilBERT is open-source and available for free as part of the Hugging Face Transformers library. This makes it accessible to developers, researchers, and organizations looking to implement advanced NLP capabilities without incurring licensing fees. However, users may need to consider potential costs associated with cloud computing resources or infrastructure if they choose to deploy DistilBERT in a production environment.
For organizations that require additional support, consulting, or custom solutions, Hugging Face offers enterprise-level services that may come with associated costs. These services can include advanced model training, deployment assistance, and ongoing support.
Comparison with Other Tools
When comparing DistilBERT to other NLP models, several key differentiators emerge:
-
Versus BERT: While BERT is a powerful model, it is large and resource-intensive. DistilBERT addresses these limitations by providing a smaller, faster alternative that retains most of BERT's performance.
-
Versus ALBERT: ALBERT (A Lite BERT) is another lightweight version of BERT that reduces model size through parameter sharing. However, DistilBERT's knowledge distillation approach allows it to maintain a high level of performance with fewer parameters.
-
Versus RoBERTa: RoBERTa is an optimized version of BERT that improves performance through training on larger datasets and longer training times. While RoBERTa may outperform BERT and DistilBERT in certain benchmarks, it is also larger and slower, making DistilBERT a better choice for applications with resource constraints.
-
Versus GPT-3: GPT-3 (Generative Pre-trained Transformer 3) is a powerful language model known for its generative capabilities. However, GPT-3 is also significantly larger and requires substantial computational resources. DistilBERT, while not as versatile in generation tasks, excels in understanding and classification tasks with a much smaller footprint.
-
Versus Other Distilled Models: Other distilled models exist, but DistilBERT is particularly noteworthy for its balance of size, speed, and performance. It has been widely adopted and is supported by a robust community and ecosystem.
FAQ
What is knowledge distillation, and how does it apply to DistilBERT?
Knowledge distillation is a training technique where a smaller model (the student) is trained to replicate the behavior of a larger, pre-trained model (the teacher). In the case of DistilBERT, the model is trained to predict the same probabilities for masked tokens as the larger BERT model while also minimizing the distance between their hidden states. This allows DistilBERT to learn essential language features from BERT while being more compact and efficient.
How does DistilBERT perform compared to BERT on various NLP tasks?
DistilBERT retains over 95% of BERT's performance on the GLUE language understanding benchmark, making it highly effective for a range of NLP tasks. While it may not outperform BERT in every scenario, its efficiency and speed make it a strong contender for applications with limited resources.
Can DistilBERT be fine-tuned for specific tasks?
Yes, DistilBERT can be easily fine-tuned for various NLP tasks, including text classification, token classification, and question answering. The Hugging Face Transformers library provides straightforward APIs and examples for fine-tuning the model on specific datasets.
Is DistilBERT suitable for deployment on mobile devices?
Yes, DistilBERT is designed to be lightweight and efficient, making it suitable for deployment on mobile devices and edge computing environments. Its reduced model size and faster inference times enable it to run effectively on devices with limited computational power.
What are the hardware requirements for running DistilBERT?
DistilBERT can run on standard hardware configurations, including CPUs and GPUs. For optimal performance, especially during training and inference, it is recommended to use a GPU. The model is compatible with popular deep learning frameworks like PyTorch and TensorFlow, allowing users to leverage their existing hardware setups.
How can I get started with DistilBERT?
To get started with DistilBERT, you can install the Hugging Face Transformers library and follow the provided documentation and tutorials. The library offers pre-trained models, example scripts, and notebooks to help users quickly implement DistilBERT in their projects.
Is there a community or support available for DistilBERT users?
Yes, DistilBERT is part of the Hugging Face community, which provides extensive documentation, forums, and resources for users to connect, share knowledge, and seek assistance. The community actively contributes to the development and enhancement of DistilBERT and other models in the Transformers library.
Ready to try it out?
Go to DistilBERT