DeepPavlov
DeepPavlov is an open-source framework for building conversational AI systems, offering a range of models for tasks like Q&A, NER, and classification.
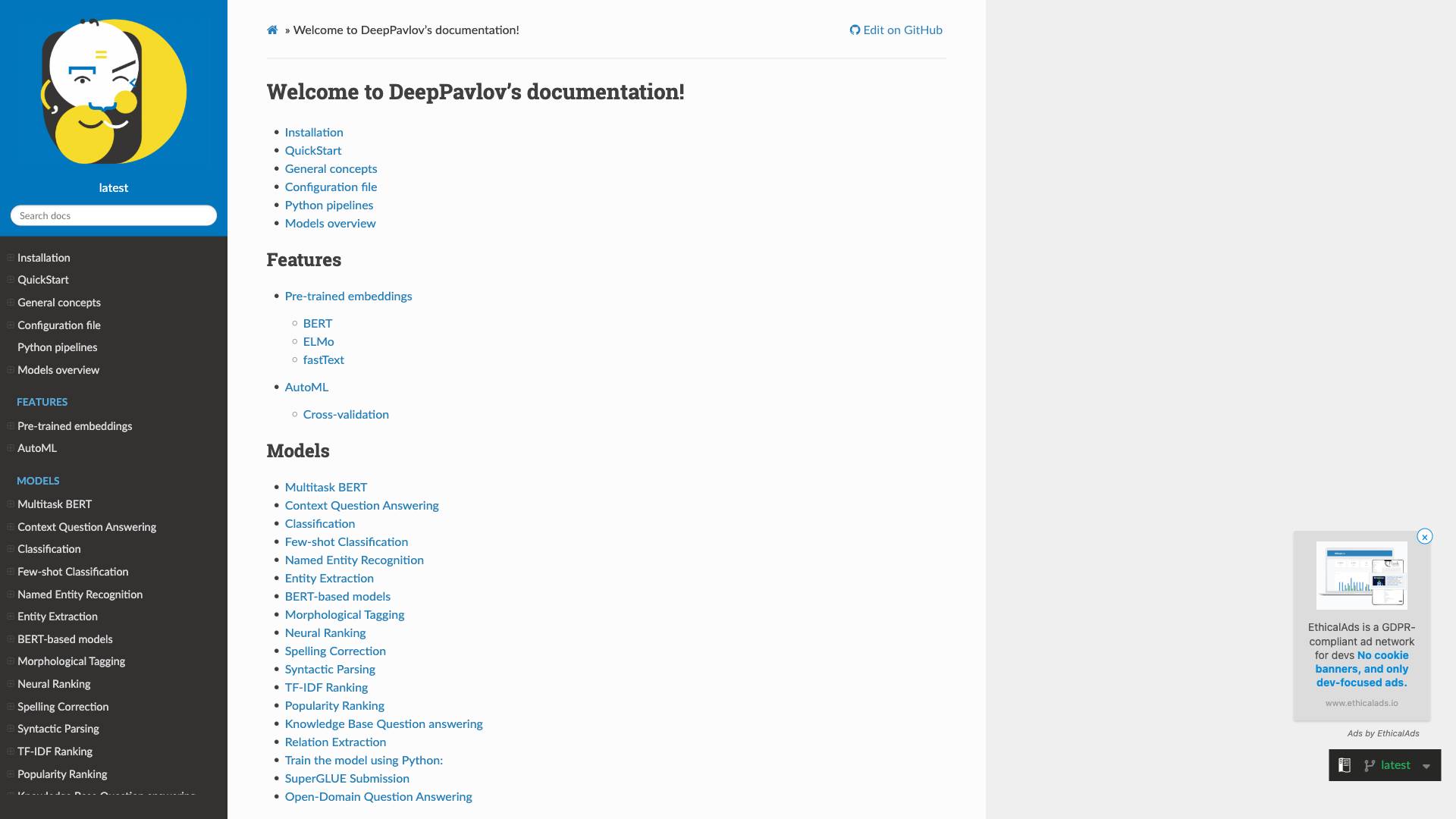
Tags
Useful for
- 1.What is DeepPavlov?
- 2.Features
- 2.1.Pre-trained Models
- 2.2.Multitask Learning
- 2.3.Advanced Configuration
- 2.4.REST API Integration
- 2.5.Socket API
- 2.6.AutoML and Cross-Validation
- 2.7.Extensive Documentation
- 2.8.Developer Guides
- 3.Use Cases
- 3.1.Chatbots and Virtual Assistants
- 3.2.Question Answering Systems
- 3.3.Named Entity Recognition (NER)
- 3.4.Text Classification
- 3.5.Relation Extraction
- 3.6.Syntactic Parsing
- 4.Pricing
- 5.Comparison with Other Tools
- 5.1.Open Source vs. Proprietary Solutions
- 5.2.Comprehensive Feature Set
- 5.3.Community Support
- 5.4.Ease of Use
- 5.5.Integration Capabilities
- 6.FAQ
- 6.1.What programming languages does DeepPavlov support?
- 6.2.Can I use my own datasets with DeepPavlov?
- 6.3.Is DeepPavlov suitable for production use?
- 6.4.How can I contribute to DeepPavlov?
- 6.5.Is there a community for DeepPavlov users?
- 6.6.Where can I find more information about DeepPavlov?
What is DeepPavlov?
DeepPavlov is an open-source framework designed for building conversational AI systems and natural language processing (NLP) applications. It provides a comprehensive suite of tools and pre-trained models that facilitate the development and deployment of intelligent agents capable of understanding and generating human language. The framework is built on top of popular machine learning libraries, making it accessible to both researchers and developers looking to implement state-of-the-art NLP solutions.
DeepPavlov is particularly focused on enabling developers to create complex dialogue systems, question answering models, and various other NLP tasks with minimal effort. Its modular architecture allows users to customize and extend existing models, making it a versatile choice for various applications in the field of AI.
Features
DeepPavlov comes packed with a rich set of features that cater to a variety of NLP tasks. Here are some of the key features:
Pre-trained Models
DeepPavlov provides a range of pre-trained models that can be utilized for various tasks, including:
- BERT: A transformer-based model that excels in understanding the context of words in search queries and text.
- ELMo: A deep contextualized word representation model that captures the meaning of words based on their context.
- fastText: A library for efficient learning of word representations and text classification.
Multitask Learning
The framework supports multitask learning, allowing users to train models that can perform multiple tasks simultaneously. This feature is particularly useful in creating more robust and versatile AI systems.
Advanced Configuration
DeepPavlov enables advanced configuration options, allowing developers to fine-tune their models and pipelines according to specific requirements. Users can manage settings files, environment variables, and dialog logging to optimize performance.
REST API Integration
DeepPavlov provides a REST API that allows easy integration of NLP models into web applications. This feature simplifies the process of deploying models and interacting with them in real-time.
Socket API
In addition to the REST API, DeepPavlov offers a Socket API for real-time communication with models. This is particularly beneficial for applications that require low-latency interactions, such as chatbots.
AutoML and Cross-Validation
DeepPavlov includes features for automated machine learning (AutoML) and cross-validation, which streamline the process of model training and evaluation. This helps users achieve better performance without extensive manual tuning.
Extensive Documentation
The framework comes with comprehensive documentation that covers installation, configuration, model training, and usage examples. This makes it easy for new users to get started and for experienced developers to find specific information quickly.
Developer Guides
DeepPavlov offers detailed developer guides that assist users in contributing to the project, registering their models, and understanding the framework's architecture.
Use Cases
DeepPavlov is versatile and can be applied to a wide range of use cases in natural language processing and conversational AI. Here are some prominent examples:
Chatbots and Virtual Assistants
DeepPavlov is ideal for building chatbots and virtual assistants that can understand user queries, provide relevant information, and engage in natural conversations. Its advanced NLP capabilities enable the creation of intelligent agents that can handle complex dialogue.
Question Answering Systems
With its pre-trained models and support for context-aware processing, DeepPavlov can be used to develop question answering systems that extract information from large datasets or knowledge bases. This is particularly valuable in customer support and information retrieval applications.
Named Entity Recognition (NER)
DeepPavlov includes models for named entity recognition, making it suitable for applications that require the identification of entities in text, such as names, organizations, and locations. This is essential for tasks like information extraction and data analysis.
Text Classification
The framework supports various text classification tasks, enabling users to categorize text into predefined labels. This is useful in sentiment analysis, topic categorization, and spam detection.
Relation Extraction
DeepPavlov's relation extraction capabilities allow the identification of relationships between entities in text, which can be applied in knowledge graph construction and enhancing search capabilities.
Syntactic Parsing
DeepPavlov provides tools for syntactic parsing, helping users analyze the grammatical structure of sentences. This is crucial for applications that require a deep understanding of language, such as language translation and grammar checking.
Pricing
DeepPavlov is an open-source framework, which means it is free to use and distribute. Users can download and install the framework without any licensing fees, making it an attractive option for startups, researchers, and developers looking to experiment with NLP technologies. However, while the software itself is free, users may incur costs related to cloud hosting, computational resources, or additional services they choose to integrate with their applications.
Comparison with Other Tools
When comparing DeepPavlov with other NLP frameworks and tools, several unique selling points emerge:
Open Source vs. Proprietary Solutions
DeepPavlov is completely open-source, allowing users to modify and customize the code according to their needs. In contrast, many other NLP tools may require expensive licenses and may not offer the same level of flexibility.
Comprehensive Feature Set
While there are various NLP frameworks available, DeepPavlov stands out due to its extensive feature set, including pre-trained models, multitask learning, and advanced configuration options. This makes it a one-stop solution for many NLP tasks.
Community Support
As an open-source project, DeepPavlov benefits from a community of developers and researchers who contribute to its improvement. This collaborative environment fosters innovation and ensures that the framework stays up to date with the latest advancements in NLP.
Ease of Use
DeepPavlov is designed with usability in mind, featuring comprehensive documentation and a straightforward setup process. This makes it accessible to beginners while still offering advanced features for experienced users.
Integration Capabilities
DeepPavlov's support for REST and Socket APIs simplifies the integration of NLP models into applications, making it easier for developers to deploy their solutions in real-world scenarios.
FAQ
What programming languages does DeepPavlov support?
DeepPavlov is primarily built for Python, which is one of the most widely used programming languages in the field of data science and machine learning. Users should have a basic understanding of Python to effectively use the framework.
Can I use my own datasets with DeepPavlov?
Yes, DeepPavlov allows users to train models on their own datasets. The framework provides tools for data preprocessing and model training, making it easy to adapt existing models to specific use cases.
Is DeepPavlov suitable for production use?
Absolutely! DeepPavlov is designed to be production-ready, with features such as REST API and Socket API integration that facilitate real-time interactions. Many organizations use DeepPavlov in production environments for various NLP applications.
How can I contribute to DeepPavlov?
DeepPavlov encourages contributions from the community. Users can contribute by reporting issues, submitting pull requests, or providing feedback on the documentation. Detailed developer guides are available to assist new contributors.
Is there a community for DeepPavlov users?
Yes, DeepPavlov has an active community of users and developers who share knowledge, provide support, and collaborate on projects. Users can engage with the community through forums, social media, and GitHub discussions.
Where can I find more information about DeepPavlov?
Comprehensive documentation is available on the DeepPavlov website, covering installation, configuration, model training, and usage examples. This resource is invaluable for both new and experienced users looking to leverage the framework's capabilities.
In summary, DeepPavlov is a powerful and flexible framework for building conversational AI systems and NLP applications. With its extensive feature set, ease of use, and active community support, it is an excellent choice for developers and researchers looking to create intelligent language-based solutions.
Ready to try it out?
Go to DeepPavlov