CNTK
CNTK is an open-source toolkit for distributed deep learning, enabling efficient neural network modeling and interoperability with ONNX.
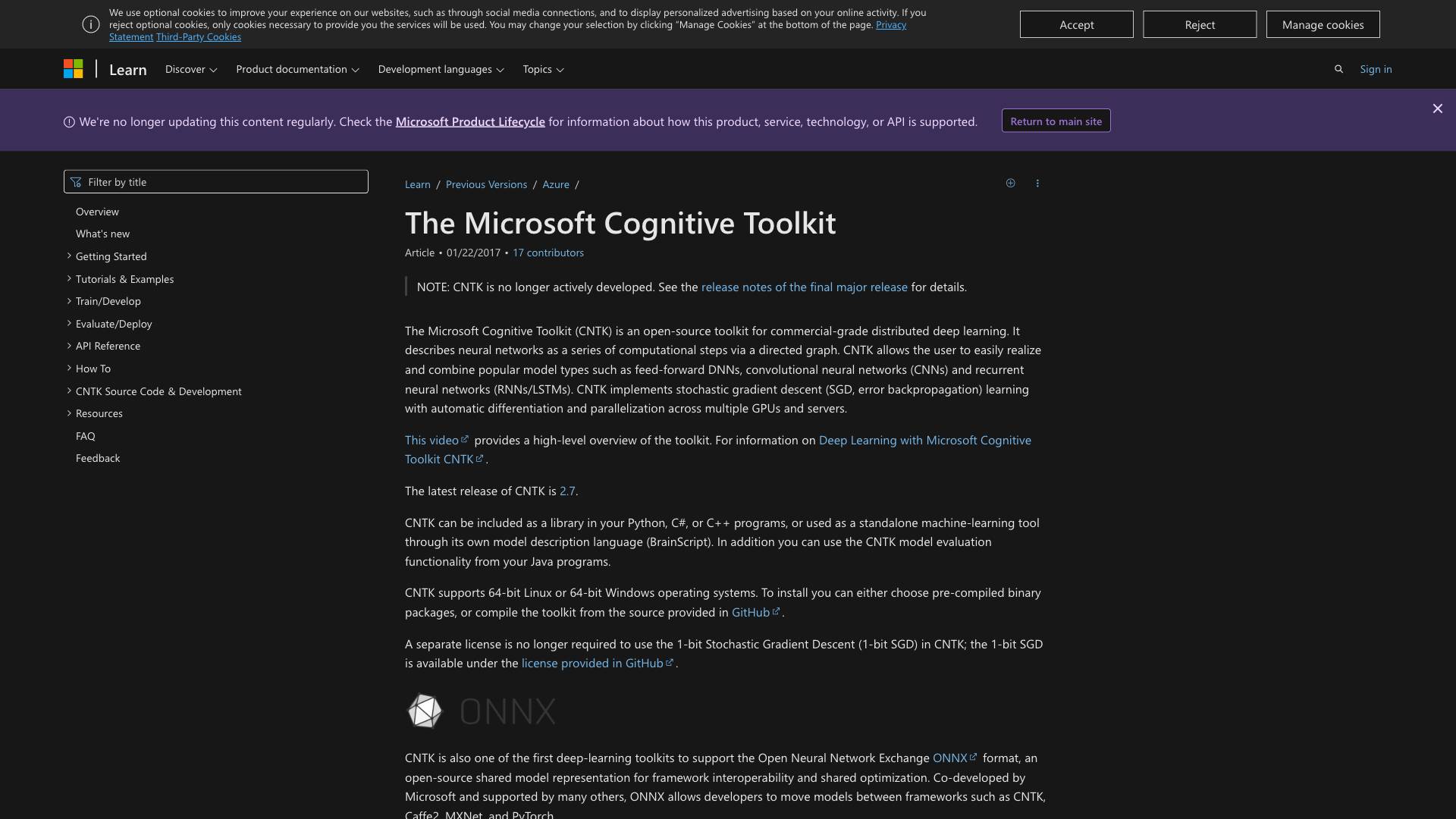
Tags
Useful for
- 1.What is CNTK?
- 2.Features
- 2.1.1. Open-Source Toolkit
- 2.2.2. Flexible Model Representation
- 2.3.3. Support for Multiple Programming Languages
- 2.4.4. High-Performance Training
- 2.5.5. Support for Popular Neural Network Types
- 2.6.6. Integration with ONNX
- 2.7.7. Model Description Language (BrainScript)
- 2.8.8. Comprehensive Documentation
- 2.9.9. Community and Contributions
- 3.Use Cases
- 3.1.1. Image Classification
- 3.2.2. Natural Language Processing (NLP)
- 3.3.3. Speech Recognition
- 3.4.4. Recommender Systems
- 3.5.5. Time Series Forecasting
- 3.6.6. Anomaly Detection
- 4.Pricing
- 5.Comparison with Other Tools
- 5.1.1. Ease of Use
- 5.2.2. Performance
- 5.3.3. Community and Support
- 5.4.4. Model Interoperability
- 6.FAQ
- 6.1.1. Is CNTK still actively developed?
- 6.2.2. Can I use CNTK for commercial purposes?
- 6.3.3. What programming languages does CNTK support?
- 6.4.4. Is CNTK suitable for beginners?
- 6.5.5. How do I install CNTK?
- 6.6.6. What types of neural networks can I build with CNTK?
- 6.7.7. Can I use CNTK for real-time applications?
- 6.8.8. Where can I find resources to learn CNTK?
What is CNTK?
The Microsoft Cognitive Toolkit, commonly known as CNTK, is an open-source deep learning toolkit designed for commercial-grade distributed deep learning. It allows users to build, train, and evaluate deep learning models efficiently. CNTK represents neural networks as a series of computational steps organized in a directed graph, enabling complex model architectures to be created and manipulated with ease. Although CNTK is no longer actively developed, its features and capabilities make it a noteworthy tool in the realm of machine learning and artificial intelligence.
CNTK supports various model types including feed-forward deep neural networks (DNNs), convolutional neural networks (CNNs), and recurrent neural networks (RNNs/LSTMs). Its robust implementation of stochastic gradient descent (SGD) facilitates efficient training across multiple GPUs and servers, making it suitable for large-scale machine learning tasks.
Features
CNTK comes packed with a wide array of features that cater to both beginners and advanced users in the field of deep learning. Here are some of the most significant features:
1. Open-Source Toolkit
CNTK is an open-source project, which means that users can freely access, modify, and distribute the source code. This openness encourages collaboration and innovation within the community.
2. Flexible Model Representation
CNTK allows users to describe neural networks using a directed graph structure. This flexibility means that complex architectures can be easily constructed and modified.
3. Support for Multiple Programming Languages
Users can integrate CNTK into their applications using various programming languages, including:
- Python
- C#
- C++
- Java (for model evaluation)
4. High-Performance Training
CNTK implements advanced training techniques, including:
- Stochastic Gradient Descent (SGD): Efficiently optimizes model parameters.
- Automatic Differentiation: Simplifies the process of computing gradients for complex models.
- Parallelization: Leverages multiple GPUs and servers to speed up training processes.
5. Support for Popular Neural Network Types
CNTK supports several types of neural networks, including:
- Feed-forward DNNs: Ideal for tasks like image classification and regression.
- Convolutional Neural Networks (CNNs): Used primarily for image processing tasks.
- Recurrent Neural Networks (RNNs/LSTMs): Well-suited for sequential data tasks, like natural language processing (NLP).
6. Integration with ONNX
CNTK is one of the first deep learning toolkits to support the Open Neural Network Exchange (ONNX) format. This support facilitates model interoperability across different frameworks, such as Caffe2, MXNet, and PyTorch, allowing developers to transfer models easily.
7. Model Description Language (BrainScript)
CNTK features its own model description language called BrainScript, which allows users to define and train models using a text-based format. This can be particularly useful for users who prefer a declarative approach to model building.
8. Comprehensive Documentation
CNTK provides extensive documentation, tutorials, and examples, helping users quickly understand how to utilize the toolkit effectively.
9. Community and Contributions
While CNTK is no longer actively developed, it has a history of contributions from a diverse group of developers and researchers, fostering a rich ecosystem of shared knowledge and resources.
Use Cases
CNTK is suited for various applications in deep learning and machine learning. Here are some notable use cases:
1. Image Classification
Using CNNs, CNTK can be employed to classify images into predefined categories. This is widely used in applications such as facial recognition, object detection, and medical imaging.
2. Natural Language Processing (NLP)
RNNs and LSTMs in CNTK can be utilized for tasks like sentiment analysis, language translation, and text generation, making it a powerful tool for processing sequential data.
3. Speech Recognition
CNTK can be applied in speech recognition systems, allowing for the conversion of spoken language into text, which is useful in applications like virtual assistants and transcription services.
4. Recommender Systems
By leveraging DNNs, CNTK can be used to create models that provide personalized recommendations based on user behavior and preferences, enhancing user experiences in e-commerce and content platforms.
5. Time Series Forecasting
CNTK can be used to analyze and predict trends in time series data, which is valuable for applications in finance, weather forecasting, and inventory management.
6. Anomaly Detection
CNTK can help in identifying unusual patterns in data, which is crucial for fraud detection, network security, and fault detection in manufacturing processes.
Pricing
CNTK is an open-source toolkit, meaning it is available for free. Users can download and utilize the toolkit without any licensing fees. This makes CNTK an attractive option for startups, researchers, and organizations looking to implement deep learning solutions without incurring significant costs.
Comparison with Other Tools
When comparing CNTK to other popular deep learning frameworks like TensorFlow, PyTorch, and Keras, several factors come into play:
1. Ease of Use
- Keras: Known for its user-friendly API, Keras is often preferred by beginners for rapid prototyping.
- TensorFlow: While TensorFlow has improved its usability, it may still present a steeper learning curve compared to Keras.
- PyTorch: Offers a dynamic computational graph which is more intuitive for certain users, particularly in research settings.
- CNTK: While powerful, CNTK's unique model description language (BrainScript) may not be as intuitive for new users.
2. Performance
- CNTK: Excels in performance, particularly in scenarios involving distributed training across multiple GPUs and servers.
- TensorFlow and PyTorch: Both are highly optimized for performance but may require more configuration compared to CNTK.
- Keras: Performance depends on the backend (usually TensorFlow), and while it’s efficient, it may not match the raw performance of CNTK in specific scenarios.
3. Community and Support
- TensorFlow: Has a large and active community, providing extensive resources, tutorials, and support.
- PyTorch: Also boasts a growing community, especially in academic circles.
- Keras: Benefits from TensorFlow's community but may have fewer dedicated resources.
- CNTK: While it has contributed to the deep learning community, its lack of active development means fewer updates and community contributions.
4. Model Interoperability
- CNTK: Supports ONNX, allowing for easy model sharing between different frameworks.
- TensorFlow and PyTorch: Both have their own ecosystems but are increasingly supporting ONNX for interoperability.
- Keras: Primarily tied to TensorFlow but can also export models to ONNX with some additional steps.
FAQ
1. Is CNTK still actively developed?
No, CNTK is no longer actively developed. The last major release was 2.7, and users are encouraged to refer to the release notes for details.
2. Can I use CNTK for commercial purposes?
Yes, CNTK is open-source and can be used for commercial applications without any licensing fees.
3. What programming languages does CNTK support?
CNTK supports Python, C#, C++, and provides model evaluation functionality for Java programs.
4. Is CNTK suitable for beginners?
While CNTK has a steep learning curve compared to some other frameworks like Keras, its comprehensive documentation can help beginners get started.
5. How do I install CNTK?
CNTK can be installed using pre-compiled binary packages or by compiling the toolkit from the source code available on GitHub.
6. What types of neural networks can I build with CNTK?
CNTK supports a variety of neural network types, including feed-forward DNNs, CNNs, and RNNs/LSTMs.
7. Can I use CNTK for real-time applications?
Yes, CNTK can be optimized for real-time applications, especially when leveraging its parallelization capabilities across multiple GPUs.
8. Where can I find resources to learn CNTK?
CNTK provides extensive documentation, tutorials, and community forums to help users learn and troubleshoot their implementations.
In conclusion, while CNTK may not be the latest tool in the deep learning landscape, its robust features and capabilities make it a valuable option for various applications in machine learning and artificial intelligence.
Ready to try it out?
Go to CNTK