CLIPSeg
CLIPSeg enables zero-shot and one-shot image segmentation using flexible text or image prompts, enhancing adaptability for diverse segmentation tasks.
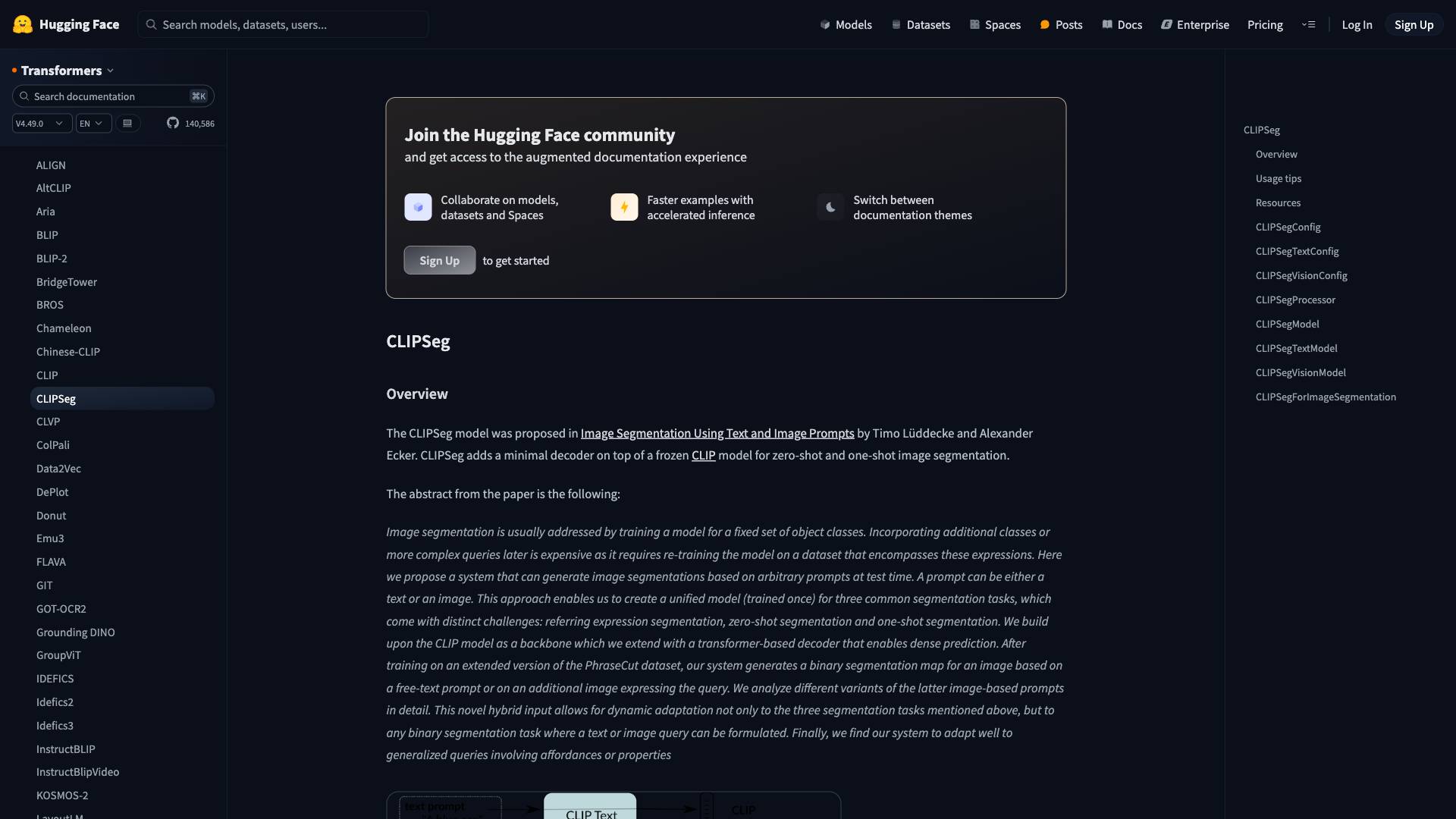
Tags
Useful for
- 1.What is CLIPSeg?
- 2.Features
- 3.Use Cases
- 4.Pricing
- 5.Comparison with Other Tools
- 6.FAQ
- 6.1.What is the primary advantage of using CLIPSeg over traditional segmentation models?
- 6.2.Can CLIPSeg handle multiple segmentation tasks?
- 6.3.What types of prompts can be used with CLIPSeg?
- 6.4.Is CLIPSeg suitable for real-time applications?
- 6.5.How can I customize the CLIPSeg model for my specific needs?
- 6.6.Where can I find resources to help me get started with CLIPSeg?
- 6.7.Is there a community or support network for CLIPSeg users?
What is CLIPSeg?
CLIPSeg is an advanced image segmentation model that leverages the capabilities of the CLIP (Contrastive Language-Image Pretraining) architecture. Proposed in the paper "Image Segmentation Using Text and Image Prompts" by Timo Lüddecke and Alexander Ecker, CLIPSeg introduces a minimal decoder on top of a frozen CLIP model, enabling zero-shot and one-shot image segmentation.
The primary innovation of CLIPSeg is its ability to generate image segmentations based on arbitrary prompts provided at test time. These prompts can be either text descriptions or additional images, allowing for versatile and dynamic segmentation tasks. This approach eliminates the need for extensive retraining when new object classes or complex queries are introduced, making it a highly efficient solution for various segmentation challenges.
Features
CLIPSeg comes with a range of features that enhance its usability and flexibility for image segmentation tasks:
-
Zero-shot Segmentation: CLIPSeg can perform segmentation without requiring prior training on specific classes, allowing it to adapt to new tasks on-the-fly.
-
One-shot Segmentation: The model can also segment images based on a single example, making it useful for scenarios where labeled data is scarce.
-
Text and Image Prompts: Users can provide prompts in the form of text descriptions or example images, granting the model the ability to understand complex queries and perform segmentation accordingly.
-
Hybrid Input Capabilities: CLIPSeg supports both text and image inputs, enabling it to tackle a wide array of segmentation tasks without the need for extensive retraining.
-
Unified Model for Multiple Tasks: The model is designed to handle three common segmentation challenges—referring expression segmentation, zero-shot segmentation, and one-shot segmentation—within a single framework.
-
Transformer-based Decoder: By extending the CLIP model with a transformer-based decoder, CLIPSeg can generate dense prediction outputs, enhancing the quality and granularity of segmentations.
-
Dynamic Adaptation: The model is capable of adapting to generalized queries involving various properties or affordances, making it versatile for different applications.
-
Pre-trained on Extensive Datasets: CLIPSeg is trained on an extended version of the PhraseCut dataset, ensuring that it has a robust understanding of various segmentation tasks from the outset.
-
Configuration Flexibility: Users can customize various parameters of the model, including the number of attention heads, dimensionality of layers, and dropout ratios, to optimize performance for specific use cases.
Use Cases
CLIPSeg is suitable for a wide range of applications across different domains, including:
-
Medical Imaging: In healthcare, CLIPSeg can assist in segmenting anatomical structures or lesions in medical images, aiding in diagnosis and treatment planning.
-
Autonomous Vehicles: The model can be employed in self-driving technology to identify and segment objects in the vehicle's environment, such as pedestrians, other vehicles, and road signs.
-
Augmented Reality: CLIPSeg can enhance AR experiences by accurately segmenting real-world objects, enabling interactive features and overlays.
-
Image Editing: Photographers and graphic designers can utilize CLIPSeg to isolate subjects in images for editing, retouching, or compositing tasks.
-
Robotics: In robotics, CLIPSeg can be used for object recognition and manipulation, allowing robots to interact with their surroundings more effectively.
-
Content Creation: For content creators, the ability to segment images based on textual descriptions can streamline workflows in video production, animation, and digital art.
-
Fashion and Retail: Retailers can use CLIPSeg to segment clothing items in images, facilitating virtual try-ons and personalized shopping experiences.
-
Environmental Monitoring: The model can assist in segmenting and analyzing satellite images for applications such as land use classification, deforestation tracking, and urban planning.
Pricing
As of now, specific pricing details for CLIPSeg are not provided in the available documentation. However, the tool is part of the Hugging Face ecosystem, which often offers various pricing models including:
-
Free Tier: Basic access to the model with limited usage and features.
-
Subscription Plans: Paid plans that offer enhanced features, higher usage limits, and additional support.
-
Enterprise Solutions: Customized pricing for organizations requiring extensive usage, dedicated support, or integration into existing workflows.
It is advisable for potential users to check the Hugging Face website or contact their sales team for the most accurate and up-to-date pricing information.
Comparison with Other Tools
When compared to other image segmentation tools and models, CLIPSeg stands out in several key areas:
-
Flexibility: Unlike traditional segmentation models that require retraining for new classes, CLIPSeg's zero-shot and one-shot capabilities allow it to adapt to new tasks without extensive additional training.
-
Prompt-Based Segmentation: Many existing models rely solely on fixed class labels. CLIPSeg's ability to utilize both text and image prompts provides a more intuitive and versatile user experience.
-
Unified Framework: While many segmentation models are designed for specific tasks, CLIPSeg combines multiple segmentation methodologies into a single model, streamlining the workflow for users who need to perform various tasks.
-
Quality of Output: The integration of a transformer-based decoder enhances the quality and granularity of segmentations, making CLIPSeg suitable for applications requiring high precision.
-
Community and Support: Being part of the Hugging Face ecosystem means that users have access to a robust community and extensive resources, including documentation, tutorials, and forums for troubleshooting.
-
Customization Options: CLIPSeg offers a range of configuration options that allow users to tailor the model to their specific needs, providing greater control over performance and output.
FAQ
What is the primary advantage of using CLIPSeg over traditional segmentation models?
The primary advantage of CLIPSeg is its ability to perform zero-shot and one-shot segmentation based on arbitrary prompts. This flexibility allows users to adapt the model to new tasks without requiring retraining, making it a highly efficient tool for various applications.
Can CLIPSeg handle multiple segmentation tasks?
Yes, CLIPSeg is designed to handle multiple segmentation tasks, including referring expression segmentation, zero-shot segmentation, and one-shot segmentation, all within a unified model framework.
What types of prompts can be used with CLIPSeg?
CLIPSeg supports prompts in the form of text descriptions or additional images, allowing users to interact with the model in a more intuitive manner.
Is CLIPSeg suitable for real-time applications?
While CLIPSeg is capable of generating segmentations quickly, the suitability for real-time applications will depend on the specific hardware and implementation. Users may need to optimize the model for speed in such scenarios.
How can I customize the CLIPSeg model for my specific needs?
CLIPSeg offers a range of configuration options that allow users to adjust parameters such as the number of attention heads, dimensionality of layers, and dropout ratios. This customization enables users to optimize the model's performance for their specific use cases.
Where can I find resources to help me get started with CLIPSeg?
Resources for getting started with CLIPSeg, including documentation, tutorials, and community support, can be found within the Hugging Face ecosystem. Users can access these resources to understand how to implement and utilize the model effectively.
Is there a community or support network for CLIPSeg users?
Yes, CLIPSeg is part of the Hugging Face community, which provides a platform for users to collaborate, share resources, and seek support from other users and developers.
In conclusion, CLIPSeg is a powerful and flexible image segmentation tool that offers unique capabilities through its integration of the CLIP model and transformer-based decoding. Its ability to adapt to various tasks without extensive retraining, combined with its support for both text and image prompts, makes it an ideal choice for a wide range of applications across different industries.
Ready to try it out?
Go to CLIPSeg