BigDL
BigDL is an optimized library for running large language models on Intel hardware with low latency and advanced quantization support.
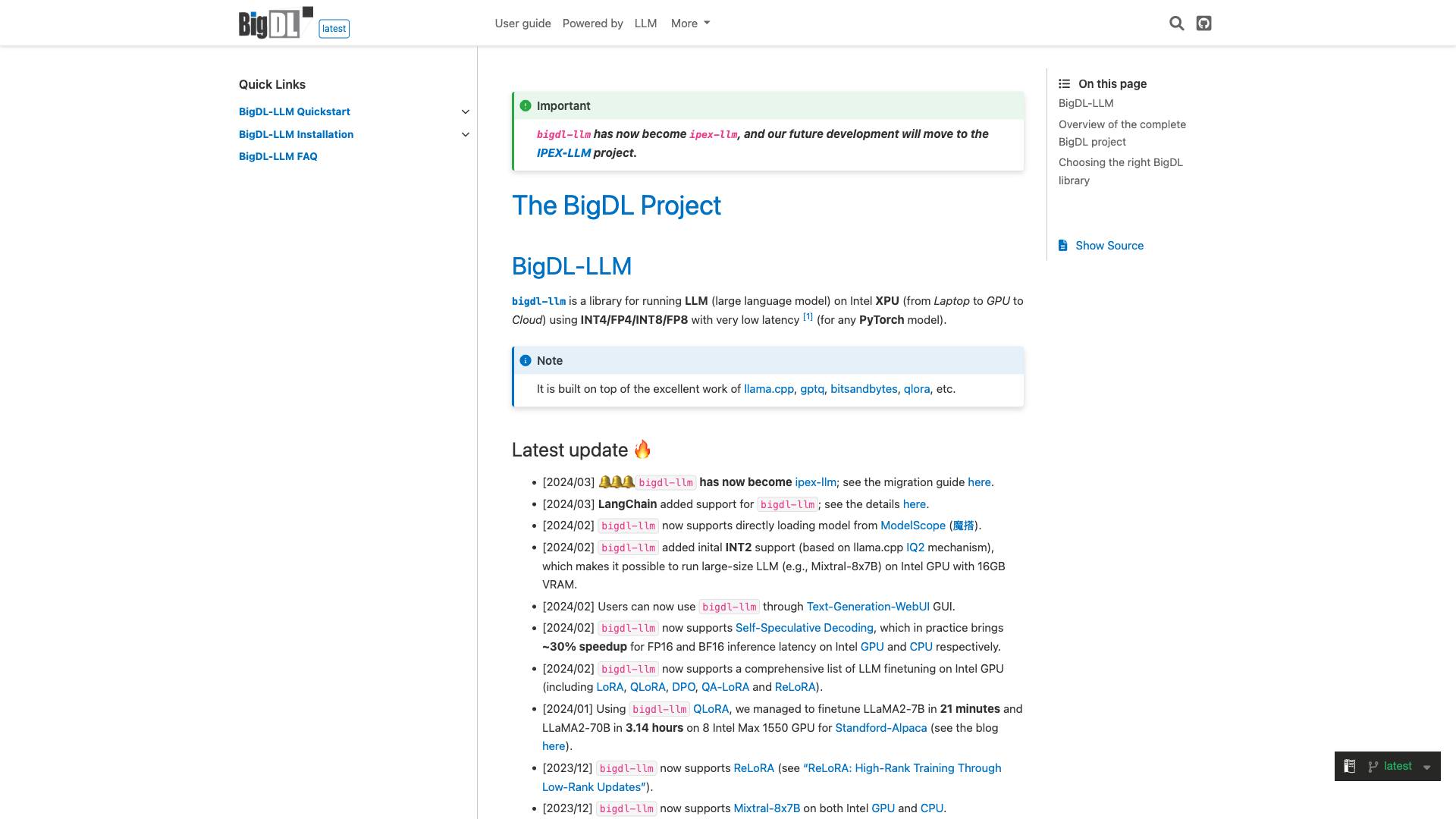
Tags
Useful for
- 1.What is BigDL?
- 2.Features
- 2.1.1. Optimized Inference
- 2.2.2. Model Compatibility
- 2.3.3. Fine-Tuning Capabilities
- 2.4.4. User-Friendly Interfaces
- 2.5.5. Performance Enhancements
- 2.6.6. Comprehensive Documentation
- 3.Use Cases
- 3.1.1. Chatbots and Conversational AI
- 3.2.2. Content Generation
- 3.3.3. Sentiment Analysis
- 3.4.4. Question Answering Systems
- 3.5.5. Language Translation
- 3.6.6. Research and Development
- 4.Pricing
- 5.Comparison with Other Tools
- 5.1.1. Intel Optimization
- 5.2.2. Quantization Techniques
- 5.3.3. Fine-Tuning Support
- 5.4.4. Integration with Existing Ecosystems
- 5.5.5. User-Friendly Interfaces
- 6.FAQ
- 6.1.1. What is the difference between BigDL and IPEX-LLM?
- 6.2.2. Can I use BigDL on non-Intel hardware?
- 6.3.3. Is BigDL suitable for production use?
- 6.4.4. What programming languages does BigDL support?
- 6.5.5. How can I get started with BigDL?
- 6.6.6. Is there a community or support for BigDL?
What is BigDL?
BigDL is an open-source library designed to facilitate the deployment and optimization of large language models (LLMs) on Intel architectures, including CPUs and GPUs. Initially known as bigdl-llm, the project has evolved into ipex-llm, focusing on high-performance inference and fine-tuning of LLMs using advanced quantization techniques such as INT4, FP4, INT8, and FP8. This library leverages the power of Intel's hardware to deliver low-latency performance for various machine learning applications.
BigDL is built on the foundation of several noteworthy projects, including llama.cpp, gptq, bitsandbytes, and qlora. It integrates seamlessly with PyTorch, making it a valuable tool for developers and researchers working in the field of natural language processing (NLP) and artificial intelligence (AI).
Features
BigDL offers a wide range of features that enhance its usability and performance. Key features include:
1. Optimized Inference
- Low Latency: BigDL provides optimized inference capabilities, enabling users to achieve very low latency when running LLMs on Intel hardware.
- Quantization Support: The library supports multiple quantization formats (INT4, FP4, INT8, FP8), which help reduce model size and improve inference speed without significantly impacting accuracy.
2. Model Compatibility
- Hugging Face Integration: Users can easily load and optimize models from the Hugging Face Transformers library, allowing for a smooth transition from other frameworks.
- Support for Various Models: BigDL has been verified with over 30 different models, including popular ones like LLaMA, ChatGLM, Mistral, and Whisper.
3. Fine-Tuning Capabilities
- Comprehensive Fine-Tuning: The library supports various fine-tuning techniques, including LoRA, QLoRA, DPO, QA-LoRA, and ReLoRA, enabling users to adapt models to specific tasks efficiently.
- Fast Fine-Tuning: BigDL boasts impressive fine-tuning speeds, such as the ability to fine-tune LLaMA2-7B in just 21 minutes on Intel hardware.
4. User-Friendly Interfaces
- Text-Generation-WebUI: BigDL can be accessed through a user-friendly GUI, making it easier for users to interact with the models without extensive programming knowledge.
- Docker Support: The library provides a Docker image for easy deployment and testing of models in containerized environments.
5. Performance Enhancements
- Self-Speculative Decoding: This feature enhances the performance of FP16 and BF16 inference by approximately 30%, improving overall processing speed on Intel CPUs and GPUs.
- Continuous Batching Support: BigDL supports vLLM continuous batching, which optimizes resource utilization and improves throughput during inference.
6. Comprehensive Documentation
- In-Depth Guides: BigDL offers extensive documentation, including installation guides, API references, and tutorials to help users get started and make the most of the library.
Use Cases
BigDL is designed for a variety of use cases in the realm of natural language processing and machine learning. Some of the prominent use cases include:
1. Chatbots and Conversational AI
BigDL can be used to develop chatbots that require the processing of natural language input and generation of human-like responses. The library's optimized inference capabilities ensure that chatbots can respond quickly, providing a seamless user experience.
2. Content Generation
Users can leverage BigDL to generate text for various applications, including marketing content, social media posts, and creative writing. The ability to fine-tune models on specific datasets allows for tailored content generation that meets specific audience needs.
3. Sentiment Analysis
BigDL can be employed to build sentiment analysis models that classify user opinions in text data. This is particularly useful for businesses looking to gauge customer sentiment from reviews, social media, and other text sources.
4. Question Answering Systems
The library's support for fine-tuning techniques makes it suitable for developing question-answering systems that can retrieve accurate answers from large datasets, enhancing user interaction with information retrieval systems.
5. Language Translation
BigDL can be utilized to create language translation models, enabling real-time translation services that can bridge communication gaps across different languages.
6. Research and Development
Researchers in the field of NLP can use BigDL to experiment with various models and fine-tuning techniques, contributing to advancements in the understanding and capabilities of language models.
Pricing
BigDL is an open-source library, which means it is available for free. Users can download and use the library without any licensing fees. However, it is important to note that while the library itself is free, users may incur costs related to the hardware they choose to run BigDL on, particularly when utilizing Intel GPUs or cloud services for deployment.
Comparison with Other Tools
When comparing BigDL with other tools in the market, several unique selling points come to light:
1. Intel Optimization
Unlike many other libraries, BigDL is specifically optimized for Intel hardware, ensuring that users can leverage the full potential of Intel CPUs and GPUs. This focus on Intel architecture can lead to superior performance in terms of speed and efficiency.
2. Quantization Techniques
BigDL's support for various quantization formats (INT4, FP4, INT8, FP8) sets it apart from other libraries that may not offer such extensive options. This flexibility allows users to choose the best quantization method for their specific use case, balancing performance and accuracy.
3. Fine-Tuning Support
The library's comprehensive fine-tuning capabilities, including support for multiple techniques, provide users with the tools they need to adapt models for specific tasks effectively. This level of support is often lacking in other libraries, which may focus primarily on inference.
4. Integration with Existing Ecosystems
BigDL's compatibility with Hugging Face Transformers and other popular libraries makes it easy for users to transition from existing workflows. This integration reduces the learning curve and enables users to leverage their existing knowledge and resources.
5. User-Friendly Interfaces
The availability of a GUI through Text-Generation-WebUI and Docker support simplifies the deployment and usage of models, making BigDL accessible to a wider audience, including those with limited programming experience.
FAQ
1. What is the difference between BigDL and IPEX-LLM?
BigDL was initially the name of the library focused on large language models, but it has since transitioned to IPEX-LLM. Users are encouraged to migrate to IPEX-LLM for future developments and enhancements.
2. Can I use BigDL on non-Intel hardware?
While BigDL is optimized for Intel architectures, it may still work on non-Intel hardware. However, performance may not be as optimized, and users may not experience the same level of efficiency as they would on Intel devices.
3. Is BigDL suitable for production use?
Yes, BigDL is designed to be robust and efficient, making it suitable for production environments. Its optimization capabilities and fine-tuning features allow for the development of high-performance applications.
4. What programming languages does BigDL support?
BigDL primarily supports Python, particularly with integration into the PyTorch ecosystem. Users can leverage Python's extensive libraries and frameworks for machine learning and data science.
5. How can I get started with BigDL?
To get started with BigDL, users can follow the installation guides provided in the documentation. The library offers tutorials and examples to help new users understand its features and capabilities effectively.
6. Is there a community or support for BigDL?
Yes, as an open-source project, BigDL has a community of users and contributors. Users can seek support through forums, GitHub repositories, and community discussions related to the library.
In conclusion, BigDL is a powerful tool for developers and researchers looking to harness the capabilities of large language models on Intel hardware. With its optimized performance, extensive feature set, and user-friendly interfaces, BigDL stands out as a leading solution in the realm of natural language processing and machine learning.
Ready to try it out?
Go to BigDL