AutoML Natural Language Vs Water Cooler Trivia Participants
Vertex AI's AutoML simplifies machine learning by automating model training for text classification, entity extraction, and sentiment analysis.
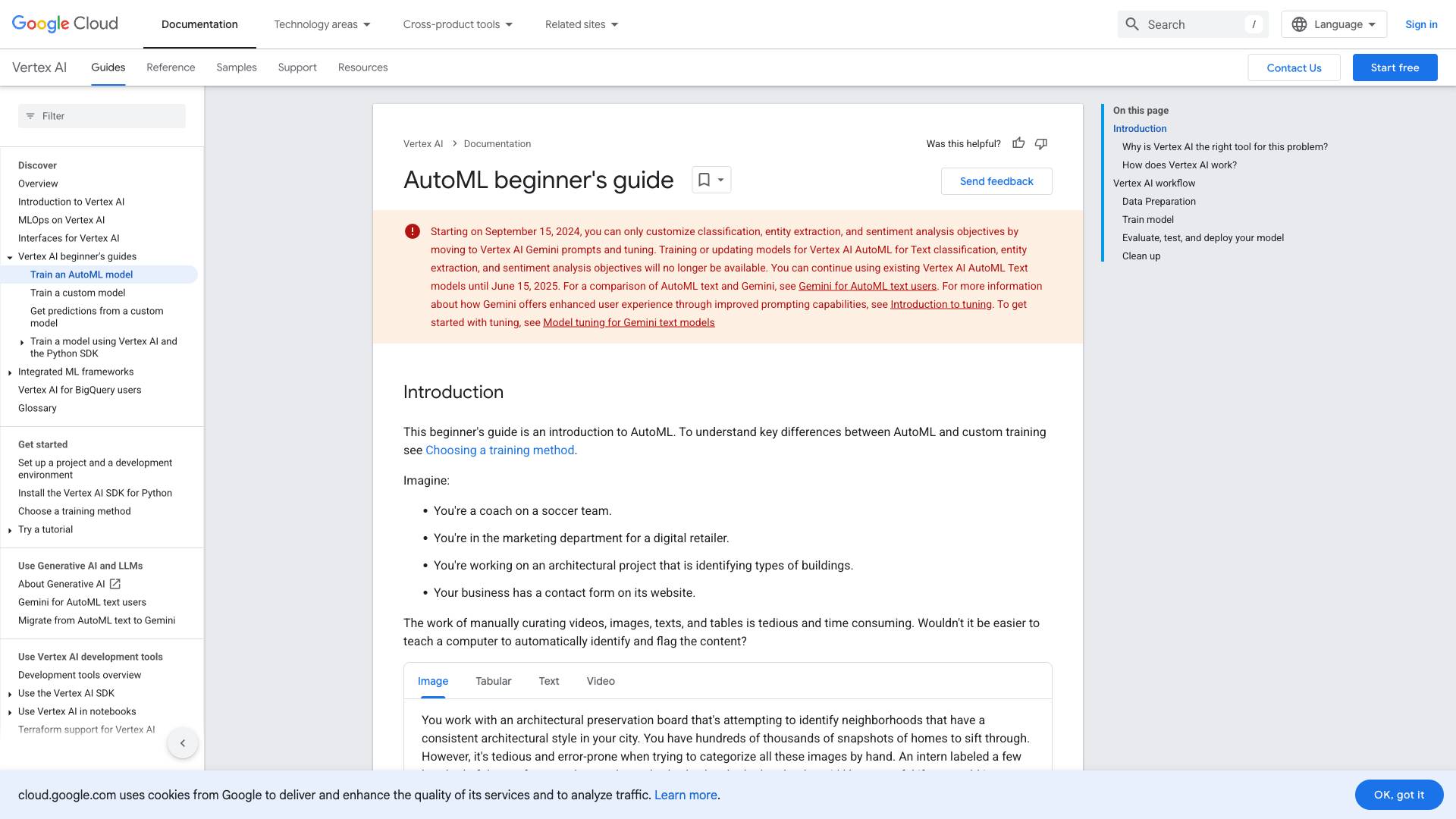
Tags
Useful for
- 1.What is AutoML Natural Language Vs Water Cooler Trivia Participants?
- 2.Features
- 2.1.1. Automated Model Training
- 2.2.2. Data Preparation Tools
- 2.3.3. Model Evaluation and Testing
- 2.4.4. Deployment Capabilities
- 2.5.5. User-Centric Design
- 3.Use Cases
- 3.1.1. Customer Feedback Analysis
- 3.2.2. Content Moderation
- 3.3.3. Trivia and Engagement Analysis
- 3.4.4. Market Research
- 3.5.5. Chatbot Development
- 4.Pricing
- 4.1.1. Training Costs
- 4.2.2. Prediction Costs
- 4.3.3. Storage Costs
- 4.4.4. Additional Services
- 5.Comparison with Other Tools
- 5.1.1. Ease of Use
- 5.2.2. Integration with Google Cloud
- 5.3.3. Scalability
- 5.4.4. Fairness and Responsible AI
- 6.FAQ
- 6.1.1. What kind of data can I use with AutoML Natural Language?
- 6.2.2. Do I need to have programming skills to use AutoML Natural Language?
- 6.3.3. How does AutoML handle data bias?
- 6.4.4. Can I customize the models created with AutoML Natural Language?
- 6.5.5. What is the expected performance of models created with AutoML Natural Language?
- 6.6.6. Is there support available for users of AutoML Natural Language?
What is AutoML Natural Language Vs Water Cooler Trivia Participants?
AutoML Natural Language is a part of Google Cloud's Vertex AI platform, designed to simplify the process of building machine learning models for natural language processing (NLP) tasks. This tool enables users to create models that can classify text, extract entities, and analyze sentiments without requiring extensive knowledge of machine learning or data science. It automates the intricate processes involved in machine learning, allowing users to focus on their specific use cases rather than the technical details of model training and deployment.
In contrast, "Water Cooler Trivia Participants" refers to a specific use case or scenario where participants engage in trivia games, often in a social or corporate setting. This context can involve utilizing AutoML Natural Language to analyze participant responses, categorize trivia questions, or evaluate engagement levels based on textual interactions. By leveraging AutoML, organizations can gain insights into participant behavior and preferences, enhancing the overall trivia experience.
Features
AutoML Natural Language offers a range of powerful features that make it suitable for various natural language processing tasks:
1. Automated Model Training
- Ease of Use: Users can train machine learning models without deep expertise in data science, thanks to the automated processes that handle data preparation, model selection, and hyperparameter tuning.
- Customizable Objectives: Users can customize models for specific tasks such as text classification, entity extraction, and sentiment analysis.
2. Data Preparation Tools
- Data Import Options: Supports importing data from various formats, including CSV and JSON Lines, enabling users to easily integrate their datasets.
- Annotation Support: Allows users to annotate data directly within the platform, simplifying the process of preparing labeled datasets for training.
3. Model Evaluation and Testing
- Performance Metrics: After training, users can evaluate model performance using metrics such as precision, recall, and F1 score, providing insights into the model's effectiveness.
- Validation and Testing Sets: Automatically splits datasets into training, validation, and testing sets, ensuring robust model evaluation.
4. Deployment Capabilities
- Seamless Integration: Once models are trained and evaluated, they can be deployed easily for real-time predictions, making it simple to integrate NLP capabilities into applications.
- Scalability: Designed to handle large datasets and high traffic, ensuring that models perform efficiently in production environments.
5. User-Centric Design
- Guided Workflows: Step-by-step workflows guide users through the process of model creation, from data preparation to deployment.
- Fairness and Inclusivity: Built with responsible AI practices in mind, promoting fairness in model predictions and reducing bias in data handling.
Use Cases
AutoML Natural Language can be applied across various industries and scenarios, enabling organizations to leverage NLP capabilities effectively. Here are some notable use cases:
1. Customer Feedback Analysis
- Sentiment Analysis: Automatically analyze customer feedback from surveys, reviews, and social media to gauge overall sentiment and identify areas for improvement.
- Topic Classification: Categorize customer comments into relevant topics, allowing businesses to prioritize responses and enhance customer service.
2. Content Moderation
- Automated Filtering: Use text classification models to flag inappropriate content in user-generated submissions or comments, ensuring community guidelines are upheld.
- Entity Recognition: Identify and extract sensitive information (e.g., personal data) from user interactions, enhancing privacy and compliance.
3. Trivia and Engagement Analysis
- Participant Insights: Analyze responses from trivia participants to understand engagement levels, preferences, and areas of interest.
- Question Classification: Automatically categorize trivia questions based on difficulty or topic, streamlining the trivia creation process.
4. Market Research
- Trend Analysis: Monitor online discussions and sentiment around products or services to identify emerging trends and customer preferences.
- Competitor Analysis: Analyze competitor mentions and sentiment to inform strategic decisions and marketing efforts.
5. Chatbot Development
- Intent Recognition: Train models to understand user intents in chatbot interactions, enabling more accurate and relevant responses.
- Contextual Understanding: Enhance chatbots' ability to maintain context in conversations, improving user experience and satisfaction.
Pricing
AutoML Natural Language operates on a pay-as-you-go pricing model, allowing users to pay only for the resources they consume. Pricing typically includes:
1. Training Costs
- Charged based on the compute resources used during model training, including the type of machine and duration of training.
2. Prediction Costs
- Fees are incurred for each prediction made by the deployed model, with costs varying based on the volume of predictions and the type of model used.
3. Storage Costs
- Users may incur charges for storing datasets, models, and other resources within Google Cloud, depending on the storage tier selected.
4. Additional Services
- Costs for additional services, such as data storage or advanced analytics features, may apply based on user requirements.
For organizations with specific needs or larger-scale projects, Google Cloud may offer customized pricing plans to accommodate varying budgets and resource requirements.
Comparison with Other Tools
When evaluating AutoML Natural Language against other tools in the market, several unique selling points and differences emerge:
1. Ease of Use
- AutoML Natural Language: Designed for users with minimal technical expertise, featuring a user-friendly interface and guided workflows.
- Other Tools: Many traditional NLP tools require substantial coding knowledge and data science expertise, making them less accessible to non-technical users.
2. Integration with Google Cloud
- AutoML Natural Language: Seamlessly integrates with other Google Cloud services, such as BigQuery and Cloud Storage, enabling users to leverage a comprehensive ecosystem for data analytics and machine learning.
- Other Tools: Competing tools may lack such seamless integration, requiring additional steps to connect with other services or platforms.
3. Scalability
- AutoML Natural Language: Built to handle large datasets and high traffic, ensuring reliable performance in production environments.
- Other Tools: Some alternative tools may struggle with scalability, especially when dealing with extensive datasets or high-demand applications.
4. Fairness and Responsible AI
- AutoML Natural Language: Emphasizes responsible AI practices, focusing on fairness and inclusivity in model training and predictions.
- Other Tools: Not all tools prioritize responsible AI, which can lead to biased models and ethical concerns.
FAQ
1. What kind of data can I use with AutoML Natural Language?
- You can use various types of textual data, including customer feedback, social media comments, and any other text-based information relevant to your use case.
2. Do I need to have programming skills to use AutoML Natural Language?
- No, AutoML Natural Language is designed for users with little to no programming experience. The platform provides guided workflows and automated processes to simplify model creation.
3. How does AutoML handle data bias?
- AutoML Natural Language incorporates responsible AI practices, focusing on fairness and inclusivity. Users are encouraged to follow best practices for data collection and annotation to mitigate bias.
4. Can I customize the models created with AutoML Natural Language?
- Yes, users can customize models for specific tasks, such as text classification and sentiment analysis, allowing for tailored solutions based on unique requirements.
5. What is the expected performance of models created with AutoML Natural Language?
- The performance of models can vary based on data quality, quantity, and the specific use case. Users can evaluate model performance using metrics such as precision and recall to gauge effectiveness.
6. Is there support available for users of AutoML Natural Language?
- Yes, Google Cloud provides documentation, tutorials, and support resources to assist users in navigating the platform and optimizing their machine learning projects.
In conclusion, AutoML Natural Language stands out as a powerful tool for organizations looking to leverage natural language processing capabilities without the complexities often associated with machine learning. Its user-friendly design, robust features, and integration with Google Cloud make it an ideal choice for a wide range of applications, from customer feedback analysis to trivia engagement insights.
Ready to try it out?
Go to AutoML Natural Language Vs Water Cooler Trivia Participants