Apple Machine Learning
Apple Machine Learning advances AI research through innovative studies, enhancing products while contributing to the broader scientific community.
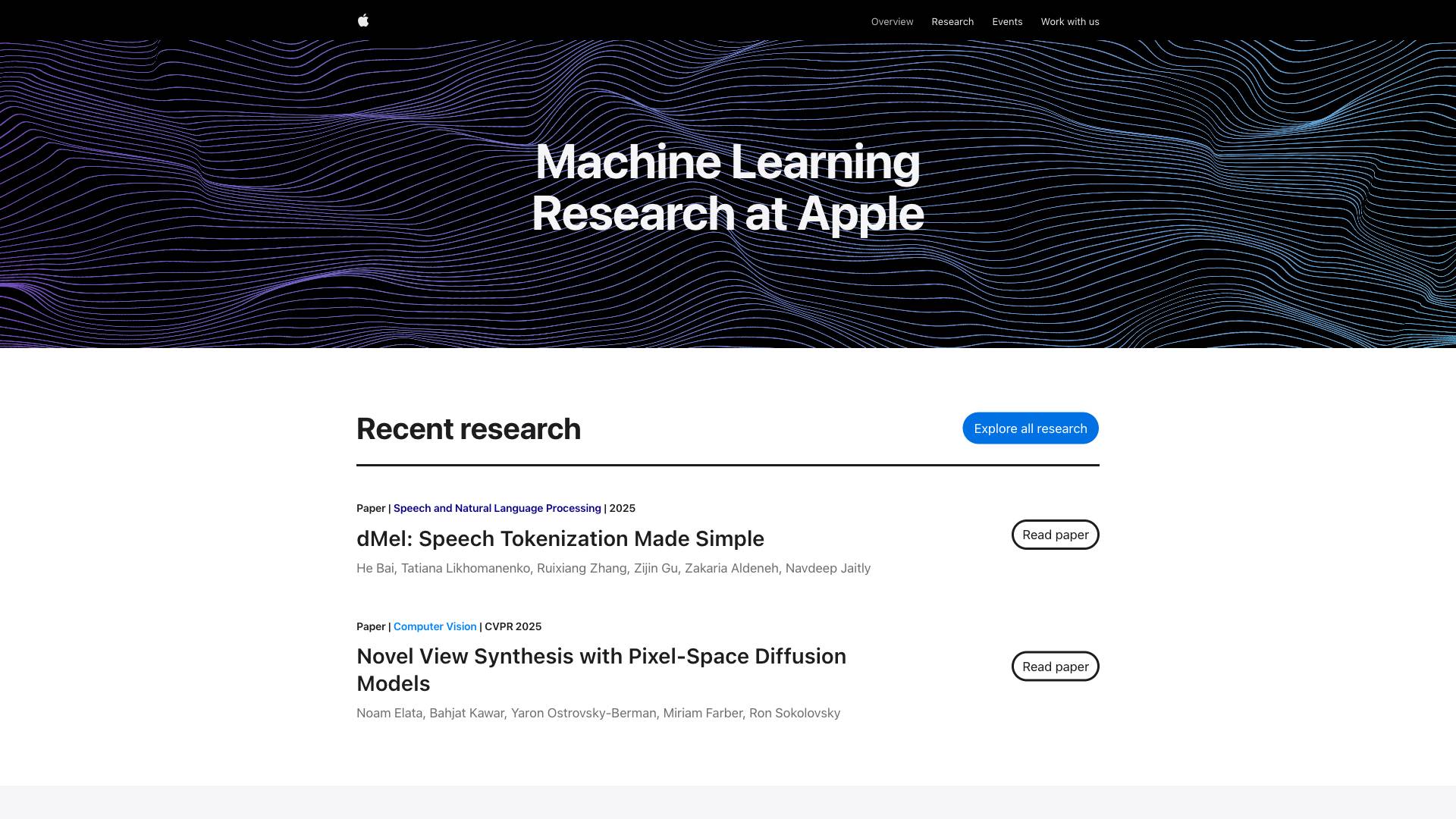
Tags
Useful for
- 1.What is Apple Machine Learning?
- 2.Features
- 2.1.1. Research Publications
- 2.2.2. Accelerated LLM Inference
- 2.3.3. Participation in Conferences
- 2.3.1.4. Open Source Contributions
- 2.4.5. Career Opportunities
- 3.Use Cases
- 3.1.1. Speech Recognition
- 3.2.2. Natural Language Processing
- 3.3.3. Computer Vision
- 3.4.4. Autonomous Systems
- 3.5.5. Healthcare
- 4.Pricing
- 5.Comparison with Other Tools
- 5.1.1. Integration with Apple Ecosystem
- 5.2.2. Research-Driven Approach
- 5.3.3. Emphasis on User Privacy
- 6.4. Open Source Contribution
- 6.1.5. Conference Engagement
- 7.FAQ
- 7.1.What types of research does Apple Machine Learning focus on?
- 7.2.How does Apple Machine Learning contribute to the broader community?
- 7.3.Can I apply for a job in Apple Machine Learning?
- 7.4.What is the significance of accelerated LLM inference?
- 7.5.How does Apple ensure user privacy in machine learning?
- 7.6.Are there any costs associated with using Apple Machine Learning?
- 7.7.Where can I find Apple Machine Learning research papers?
What is Apple Machine Learning?
Apple Machine Learning is a research initiative by Apple that focuses on advancing the field of machine learning (ML) and artificial intelligence (AI). The initiative encompasses a broad range of research areas, including but not limited to speech processing, natural language processing, and computer vision. Through its innovative research, Apple aims to improve its products and services while contributing to the broader scientific community. By engaging with industry conferences and publishing findings, Apple Machine Learning strives to redefine the possibilities of machine learning technology.
Features
Apple Machine Learning offers a variety of features that make it a robust platform for researchers and developers alike. Some of the key features include:
1. Research Publications
Apple Machine Learning publishes papers on cutting-edge research findings. Recent papers include:
- dMel: Speech Tokenization Made Simple - Focused on speech and natural language processing, this paper discusses a simplified approach to speech tokenization.
- Novel View Synthesis with Pixel-Space Diffusion Models - This work published at the CVPR conference addresses advancements in computer vision.
2. Accelerated LLM Inference
Apple has made strides in accelerating large language model (LLM) inference, particularly on NVIDIA GPUs. This feature is crucial for enhancing the efficiency of auto-regressive token generation, which is often slow and computationally intensive. By improving inference speed, Apple aims to reduce latency for users, making applications more responsive.
3. Participation in Conferences
Apple actively participates in major machine learning conferences, such as:
- Neural Information Processing Systems (NeurIPS) - This annual conference focuses on interdisciplinary research in machine learning. Apple presents new research findings and sponsors the event.
- Empirical Methods in Natural Language Processing (EMNLP) - Another significant conference where Apple shares its advancements in natural language processing and AI.
4. Open Source Contributions
Apple Machine Learning not only focuses on proprietary advancements but also shares its research with the broader community. This includes publishing papers, releasing open-source resources, and engaging with industry stakeholders to foster collaboration and innovation.
5. Career Opportunities
The initiative encourages individuals interested in machine learning to join its research team. Apple Machine Learning is committed to breaking new ground in ML research, providing a dynamic environment for collaboration and professional growth.
Use Cases
The advancements made by Apple Machine Learning can be applied across various industries and applications. Some notable use cases include:
1. Speech Recognition
The research on speech tokenization can significantly enhance voice-activated applications, such as Siri. Improved speech recognition capabilities can lead to better user experiences and more accurate interactions with devices.
2. Natural Language Processing
The findings from NLP research can be utilized in applications like chatbots, customer service automation, and content generation. The ability to process and understand human language more effectively can transform how businesses engage with their customers.
3. Computer Vision
The novel view synthesis techniques can be applied in augmented reality (AR) and virtual reality (VR) applications. By improving how machines perceive and interpret visual data, Apple can enhance user experiences in gaming, education, and training simulations.
4. Autonomous Systems
Apple's advancements in ML can be leveraged in autonomous systems, such as self-driving cars. Enhanced computer vision and natural language processing capabilities can improve navigation, object detection, and human interaction in these systems.
5. Healthcare
Machine learning can be utilized in healthcare for predictive analytics, patient monitoring, and diagnostic tools. By applying Apple’s research, healthcare providers can improve patient outcomes and streamline operations.
Pricing
As of now, Apple Machine Learning does not offer a standalone product or service with a pricing model. The initiative is primarily focused on research and development, contributing to the broader Apple ecosystem and the scientific community. However, the advancements made in machine learning are often integrated into Apple’s products and services, which may have associated costs depending on the specific offerings.
Comparison with Other Tools
When comparing Apple Machine Learning with other machine learning platforms and tools, several unique selling points stand out:
1. Integration with Apple Ecosystem
Apple Machine Learning benefits from seamless integration with Apple’s hardware and software ecosystem. This means that advancements made in ML can be directly applied to devices such as iPhones, iPads, and Macs, providing users with enhanced experiences.
2. Research-Driven Approach
Unlike many commercial ML platforms, Apple Machine Learning emphasizes fundamental research. This focus on academic rigor allows for groundbreaking discoveries that can redefine the capabilities of machine learning.
3. Emphasis on User Privacy
Apple has a strong commitment to user privacy, which extends to its machine learning initiatives. The research often focuses on enhancing ML capabilities while ensuring that user data remains secure and private.
4. Open Source Contribution
Apple Machine Learning actively shares its research findings and open-source resources with the community. This collaborative approach fosters innovation and allows other researchers and developers to build upon Apple’s work.
5. Conference Engagement
Apple’s participation in leading conferences such as NeurIPS and EMNLP sets it apart from many other tools that may not engage with the research community at the same level. This involvement helps Apple stay at the forefront of machine learning advancements.
FAQ
What types of research does Apple Machine Learning focus on?
Apple Machine Learning focuses on various research areas, including speech processing, natural language processing, and computer vision.
How does Apple Machine Learning contribute to the broader community?
Apple shares its research findings through publications, open-source resources, and participation in industry conferences, allowing others to benefit from its advancements.
Can I apply for a job in Apple Machine Learning?
Yes, Apple Machine Learning regularly seeks individuals interested in machine learning research. Opportunities are available for those looking to contribute to groundbreaking work in this field.
What is the significance of accelerated LLM inference?
Accelerated LLM inference is crucial for improving the efficiency of applications that rely on auto-regressive token generation. Faster inference reduces latency and enhances user experience.
How does Apple ensure user privacy in machine learning?
Apple prioritizes user privacy in its machine learning initiatives by implementing robust security measures and ensuring that user data is protected throughout the research and application processes.
Are there any costs associated with using Apple Machine Learning?
Currently, there is no standalone product or service associated with Apple Machine Learning that has a pricing model. The research is primarily aimed at improving Apple’s products and services.
Where can I find Apple Machine Learning research papers?
Recent research papers published by Apple Machine Learning can be found on their website, which showcases their latest findings and contributions to the field.
In conclusion, Apple Machine Learning represents a significant investment in the future of machine learning and artificial intelligence. Through its research initiatives, participation in industry events, and commitment to user privacy, Apple is poised to redefine what is possible in the realm of machine learning technology. Whether in speech recognition, natural language processing, or computer vision, the advancements made by Apple Machine Learning have the potential to transform industries and improve user experiences across the board.
Ready to try it out?
Go to Apple Machine Learning