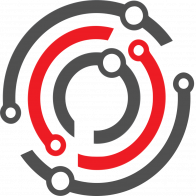
Apache Samza
Apache Samza is a stream processing framework designed for building real-time data processing applications with ease and scalability.
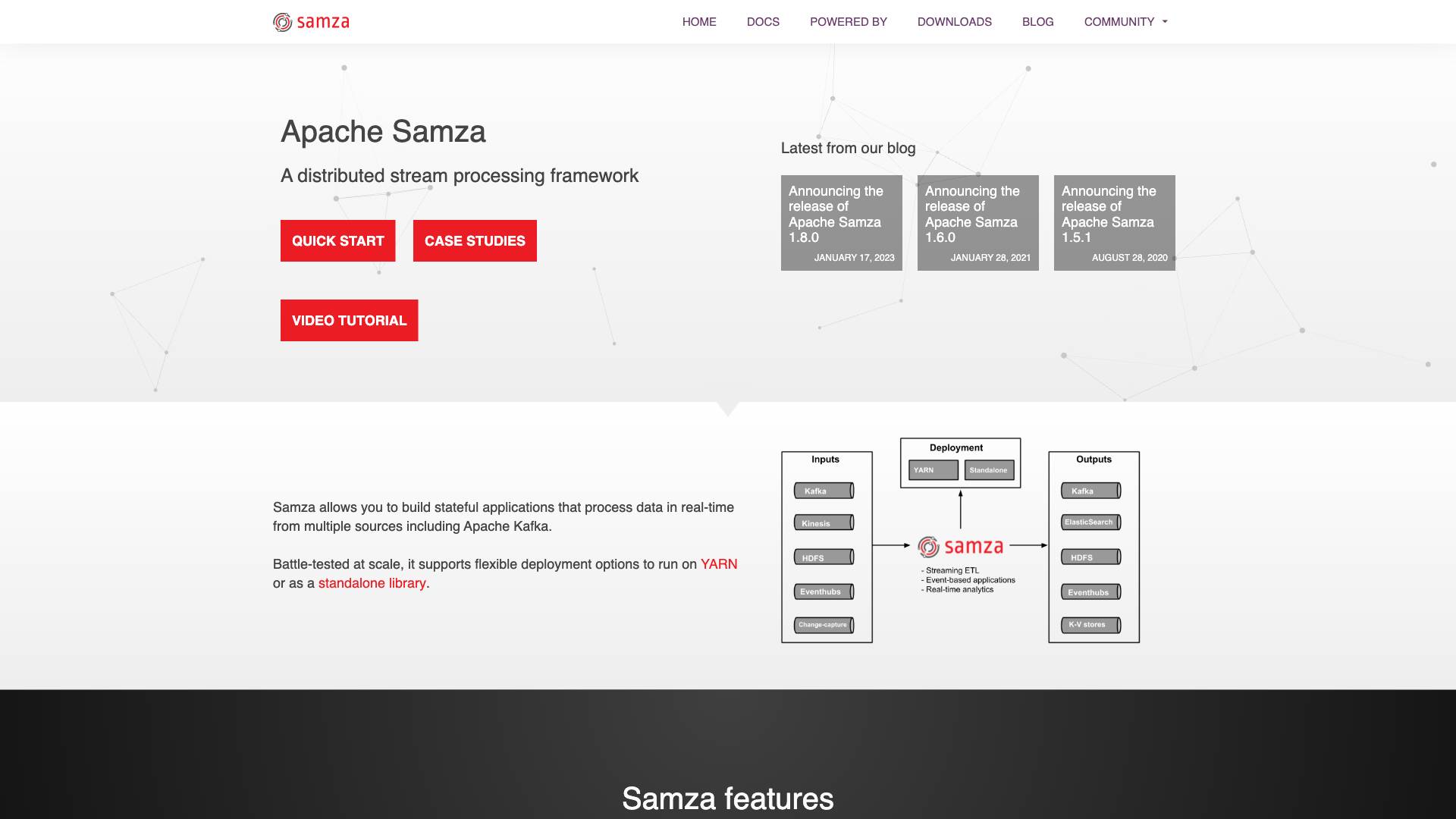
Tags
Useful for
- 1.What is Apache Samza?
- 2.Features
- 2.1.1. Stream Processing Model
- 2.2.2. Integration with Apache Kafka
- 2.3.3. Fault Tolerance
- 2.4.4. Stateful Processing
- 2.5.5. Scalability
- 2.6.6. Flexible Deployment Options
- 2.7.7. Rich Ecosystem
- 2.8.8. Ease of Use
- 2.9.9. Monitoring and Management
- 3.Use Cases
- 3.1.1. Real-time Analytics
- 3.2.2. Event-driven Applications
- 3.3.3. IoT Data Processing
- 3.4.4. Log Processing
- 3.5.5. Data Enrichment
- 3.6.6. Machine Learning Pipelines
- 4.Pricing
- 5.Comparison with Other Tools
- 5.1.1. Apache Flink
- 5.2.2. Apache Spark Streaming
- 5.3.3. Kafka Streams
- 5.4.4. Google Cloud Dataflow
- 6.FAQ
- 6.1.1. What programming languages does Apache Samza support?
- 6.2.2. Is Apache Samza suitable for batch processing?
- 6.3.3. How does Apache Samza handle state management?
- 6.4.4. Can I use Apache Samza with other messaging systems?
- 6.5.5. What are the system requirements for running Apache Samza?
What is Apache Samza?
Apache Samza is an open-source stream processing framework designed for processing large-scale data streams in real-time. It is built on top of Apache Kafka and Apache Hadoop, enabling developers to create applications that can process data in a fault-tolerant and scalable manner. Samza's architecture allows for easy integration with existing data systems and provides a robust platform for building complex data processing workflows.
Samza is particularly well-suited for use cases that require low-latency processing of data streams, making it an ideal choice for applications in various domains, including finance, e-commerce, and IoT. By leveraging Kafka for messaging and Hadoop for storage, Samza can efficiently handle vast amounts of data while ensuring reliable processing and state management.
Features
Apache Samza comes with a wide range of features that make it a powerful tool for stream processing:
1. Stream Processing Model
- Samza uses a simple programming model based on the concept of streams and processors. Developers can define processing logic as a series of transformations applied to incoming data streams.
2. Integration with Apache Kafka
- Samza seamlessly integrates with Apache Kafka, allowing it to consume and produce messages efficiently. Kafka acts as the underlying messaging system, providing durability and scalability.
3. Fault Tolerance
- Samza is designed to be fault-tolerant, ensuring that data processing continues even in the event of failures. It achieves this by maintaining state and checkpoints, allowing for recovery and reprocessing of data.
4. Stateful Processing
- Samza supports stateful processing, enabling developers to maintain and manage application state across different processing tasks. This feature is crucial for applications that require aggregations, joins, or other complex operations.
5. Scalability
- Samza's architecture allows for horizontal scaling, meaning that developers can add more processing units to handle increased workloads. This scalability is essential for applications that need to process large volumes of data in real-time.
6. Flexible Deployment Options
- Samza can be deployed in various environments, including standalone, YARN, or Kubernetes. This flexibility allows organizations to choose the deployment model that best fits their infrastructure.
7. Rich Ecosystem
- As part of the Apache Software Foundation, Samza benefits from a vibrant community and a rich ecosystem of tools and libraries. This ecosystem includes connectors to various data sources and sinks, making it easier to integrate with other systems.
8. Ease of Use
- Samza provides a user-friendly API and a straightforward programming model, making it accessible for developers with varying levels of experience in stream processing.
9. Monitoring and Management
- Samza offers built-in monitoring and management capabilities, allowing users to track the performance of their applications and manage resources effectively.
Use Cases
Apache Samza is versatile and can be applied in numerous scenarios. Here are some common use cases:
1. Real-time Analytics
- Organizations can use Samza to process and analyze data streams in real-time, enabling them to gain insights and make data-driven decisions quickly. For example, e-commerce platforms can analyze customer behavior in real-time to optimize recommendations and promotions.
2. Event-driven Applications
- Samza is well-suited for building event-driven applications that respond to incoming data in real-time. This can include applications in finance that react to market changes or fraud detection systems that analyze transactions as they occur.
3. IoT Data Processing
- With the rise of IoT devices, Samza can be used to process data generated by sensors and devices in real-time. This is critical for applications in smart cities, industrial automation, and health monitoring.
4. Log Processing
- Organizations can leverage Samza to process and analyze log data from various sources, enabling them to monitor system performance, detect anomalies, and improve security.
5. Data Enrichment
- Samza can be used to enrich data streams by combining them with additional data sources. For instance, a company might enrich customer interaction data with demographic information to improve targeting.
6. Machine Learning Pipelines
- Samza can be integrated into machine learning workflows, allowing for real-time data processing and feature extraction. This can enhance the performance of machine learning models by providing up-to-date data.
Pricing
As an open-source project, Apache Samza is free to use under the Apache License. However, organizations may incur costs related to infrastructure, support, and maintenance. The overall cost will depend on the deployment environment, the scale of data processing, and any additional tools or services used in conjunction with Samza.
Organizations may also choose to invest in commercial support or consulting services to help with implementation, optimization, and troubleshooting. This can be beneficial for teams that require expert guidance or have limited experience with stream processing.
Comparison with Other Tools
When evaluating Apache Samza, it's helpful to compare it with other stream processing frameworks. Here are some key comparisons:
1. Apache Flink
- Strengths: Flink is known for its advanced features, including event time processing and complex event processing capabilities. It also offers high throughput and low latency.
- Weaknesses: Flink can have a steeper learning curve and may require more resources for setup and maintenance compared to Samza.
2. Apache Spark Streaming
- Strengths: Spark Streaming is part of the larger Apache Spark ecosystem, making it a good choice for organizations already using Spark for batch processing. It supports micro-batch processing and has a rich set of libraries for machine learning and graph processing.
- Weaknesses: Spark Streaming's micro-batch processing model can introduce latency, making it less suitable for ultra-low-latency applications compared to Samza.
3. Kafka Streams
- Strengths: Kafka Streams is tightly integrated with Apache Kafka, allowing for easy stream processing without the need for a separate processing framework. It is lightweight and easy to use.
- Weaknesses: Kafka Streams may not offer the same level of scalability and fault tolerance as Samza, especially for complex stateful processing tasks.
4. Google Cloud Dataflow
- Strengths: Dataflow is a fully managed service that simplifies stream and batch processing. It automatically scales resources and integrates well with other Google Cloud services.
- Weaknesses: Dataflow can be more expensive, and organizations may face vendor lock-in when using a cloud-based solution.
In summary, the choice between Apache Samza and other stream processing tools will depend on specific project requirements, team expertise, and existing infrastructure.
FAQ
1. What programming languages does Apache Samza support?
- Apache Samza primarily supports Java and Scala for developing stream processing applications. This flexibility allows developers to choose the language they are most comfortable with.
2. Is Apache Samza suitable for batch processing?
- While Samza is primarily designed for stream processing, it can be integrated with batch processing frameworks like Apache Hadoop for hybrid use cases. However, it is not optimized for traditional batch processing tasks.
3. How does Apache Samza handle state management?
- Samza manages state using local storage and checkpointing mechanisms. Developers can define stateful processors that maintain application state across processing tasks, and Samza ensures that state is consistent and recoverable.
4. Can I use Apache Samza with other messaging systems?
- While Samza is optimized for use with Apache Kafka, it can be extended to work with other messaging systems through custom connectors. However, this may require additional development effort.
5. What are the system requirements for running Apache Samza?
- The system requirements for running Samza depend on the deployment model (standalone, YARN, or Kubernetes) and the scale of data processing. Generally, it requires a Java runtime environment and access to a compatible version of Apache Kafka.
In conclusion, Apache Samza is a powerful and flexible stream processing framework that caters to a wide range of real-time data processing needs. Its integration with Kafka, fault tolerance, and stateful processing capabilities make it an excellent choice for organizations looking to build scalable and reliable data-driven applications.
Ready to try it out?
Go to Apache Samza