Apache Hadoop
Apache Hadoop is an open-source framework for distributed processing of large data sets, ensuring reliability and scalability across clusters of computers.
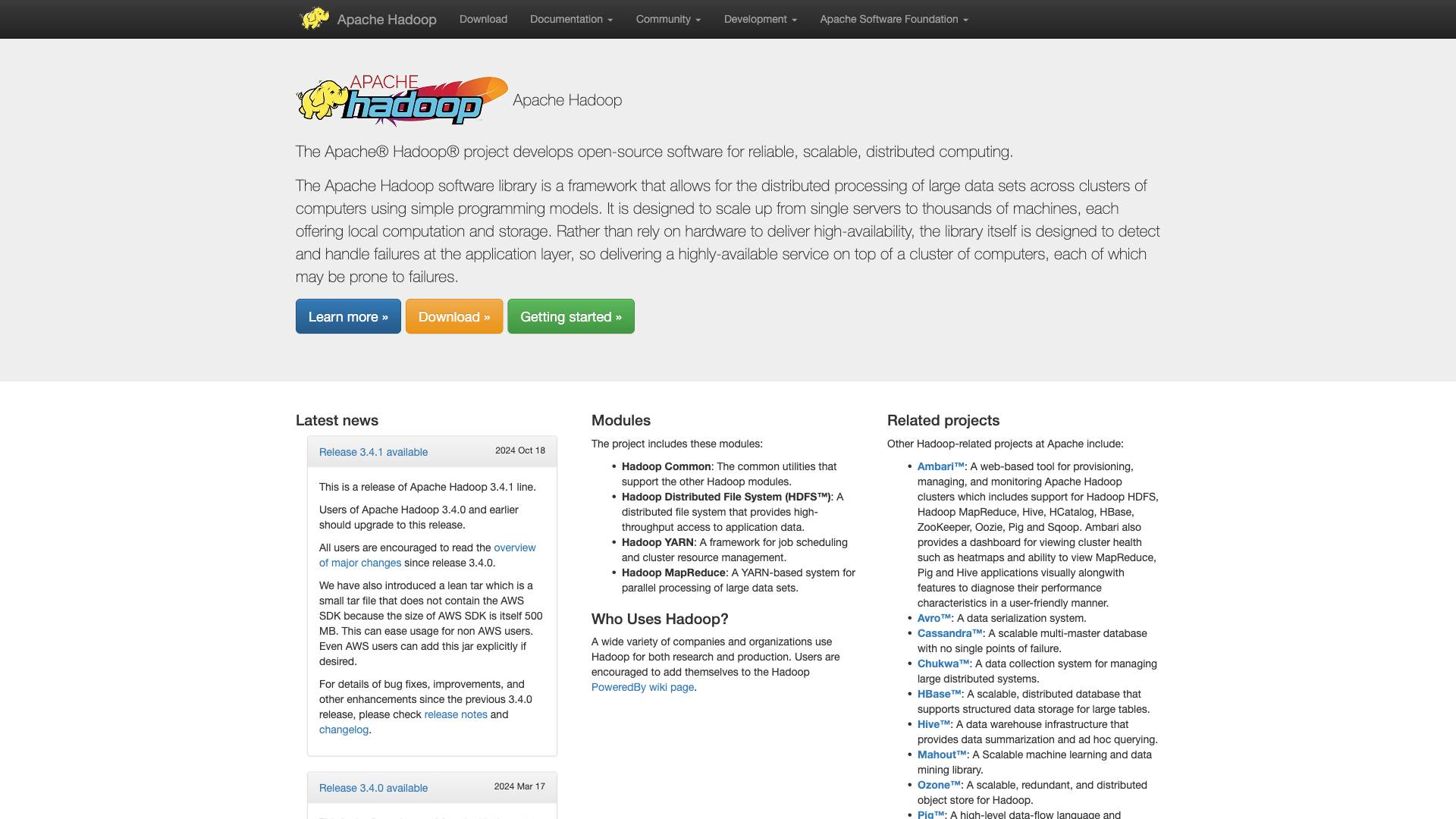
Tags
Useful for
- 1.What is Apache Hadoop?
- 2.Features
- 2.1.1. Scalability
- 2.2.2. Fault Tolerance
- 2.3.3. Cost-Effectiveness
- 2.4.4. Flexibility
- 2.5.5. High Throughput
- 2.6.6. Ecosystem Integration
- 2.7.7. Community Support
- 3.Use Cases
- 3.1.1. Data Storage and Management
- 3.2.2. Data Analytics
- 3.3.3. Machine Learning
- 3.4.4. Log Processing
- 3.5.5. Data Warehousing
- 3.6.6. Fraud Detection
- 4.Pricing
- 4.1.1. Infrastructure Costs
- 4.2.2. Support and Services
- 4.3.3. Cloud Deployment
- 5.Comparison with Other Tools
- 5.1.1. Hadoop vs. Apache Spark
- 5.2.2. Hadoop vs. Apache Flink
- 5.3.3. Hadoop vs. Traditional RDBMS
- 6.FAQ
- 6.1.1. What programming languages can I use with Hadoop?
- 6.2.2. Is Hadoop suitable for real-time data processing?
- 6.3.3. How does Hadoop ensure data security?
- 6.4.4. Can I run Hadoop on cloud platforms?
- 6.5.5. What is the role of YARN in Hadoop?
What is Apache Hadoop?
Apache Hadoop is an open-source software framework developed by the Apache Software Foundation that enables the distributed processing of large data sets across clusters of computers. It is designed to scale up from single servers to thousands of machines, each offering local computation and storage. The primary goal of Hadoop is to provide a reliable, scalable, and efficient platform for handling big data, allowing organizations to analyze vast amounts of information quickly and effectively.
Hadoop is built on a simple programming model that abstracts the complexity of distributed computing, making it accessible to developers and data scientists. The framework consists of several modules, including Hadoop Common, Hadoop Distributed File System (HDFS), Hadoop YARN (Yet Another Resource Negotiator), and Hadoop MapReduce, which work together to provide a comprehensive solution for big data processing.
Features
Apache Hadoop boasts a wide range of features that make it a powerful tool for managing and processing large datasets:
1. Scalability
Hadoop can easily scale from a single server to thousands of machines, allowing organizations to grow their data processing capabilities as their data volume increases. This horizontal scalability is one of Hadoop's key strengths.
2. Fault Tolerance
Hadoop is designed to handle hardware failures gracefully. The framework automatically replicates data across multiple nodes, ensuring that if one node fails, the data remains accessible from other nodes in the cluster. This built-in fault tolerance is crucial for maintaining high availability and reliability.
3. Cost-Effectiveness
Hadoop is an open-source framework, which means that organizations can use it without incurring licensing fees. Additionally, it can run on commodity hardware, allowing businesses to build large clusters without significant investment in expensive infrastructure.
4. Flexibility
Hadoop supports a variety of data formats, including structured, semi-structured, and unstructured data. This flexibility makes it suitable for a wide range of applications, from traditional databases to log files and multimedia content.
5. High Throughput
Hadoop is designed for high-throughput data processing, allowing it to handle large volumes of data efficiently. The MapReduce programming model enables parallel processing of data, significantly speeding up data analysis tasks.
6. Ecosystem Integration
Hadoop is part of a larger ecosystem of tools and projects that enhance its capabilities. These include Apache Hive for data warehousing, Apache HBase for NoSQL database functionality, Apache Pig for data flow scripting, and Apache Spark for in-memory data processing, among others.
7. Community Support
As an open-source project, Hadoop benefits from a large and active community of developers and users. This community contributes to the continuous improvement of the framework, providing support, documentation, and resources for users.
Use Cases
Apache Hadoop is versatile and can be applied in various industries and scenarios. Here are some common use cases:
1. Data Storage and Management
Hadoop's HDFS allows organizations to store vast amounts of data across a distributed architecture. This is particularly useful for businesses that generate large volumes of data daily, such as e-commerce sites, social media platforms, and IoT applications.
2. Data Analytics
Hadoop's MapReduce framework enables organizations to perform complex data analytics tasks, such as customer behavior analysis, trend forecasting, and sentiment analysis. This can help businesses make data-driven decisions and improve their products and services.
3. Machine Learning
Hadoop is often used as a backend for machine learning applications. By leveraging its ability to process large datasets, organizations can train machine learning models more effectively and efficiently. Tools like Apache Mahout and Apache Spark MLlib facilitate machine learning tasks within the Hadoop ecosystem.
4. Log Processing
Hadoop is ideal for processing and analyzing log files generated by web servers, applications, and network devices. Organizations can use Hadoop to extract valuable insights from these logs, such as user behavior patterns, system performance metrics, and security anomalies.
5. Data Warehousing
Hadoop can serve as a data warehouse solution, allowing organizations to consolidate data from various sources for reporting and analysis. Apache Hive provides a SQL-like interface for querying data stored in Hadoop, making it accessible to users familiar with traditional database systems.
6. Fraud Detection
Financial institutions and e-commerce companies can use Hadoop to analyze transaction data in real-time to identify fraudulent activities. By processing large volumes of data quickly, organizations can detect anomalies and respond to potential threats more effectively.
Pricing
Apache Hadoop is an open-source framework, which means there are no licensing fees associated with its use. Organizations can download and deploy Hadoop at no cost. However, there are several considerations regarding pricing:
1. Infrastructure Costs
While Hadoop itself is free, organizations must invest in the hardware required to run Hadoop clusters. This can include commodity servers, storage systems, and networking equipment. The total cost will depend on the scale of the deployment and the specific hardware chosen.
2. Support and Services
Organizations may choose to engage third-party vendors for support, training, and consulting services related to Hadoop. These services can incur additional costs, depending on the vendor and the level of support required.
3. Cloud Deployment
Many cloud providers offer Hadoop as a managed service, allowing organizations to deploy Hadoop clusters without having to manage the underlying infrastructure. While this can simplify deployment and scaling, it typically comes with associated costs based on usage.
Comparison with Other Tools
When evaluating Hadoop, it's essential to consider how it compares to other big data processing tools. Here are some key comparisons:
1. Hadoop vs. Apache Spark
- Processing Model: Hadoop uses the MapReduce model for processing, while Spark provides an in-memory processing model that can be significantly faster for certain workloads.
- Ease of Use: Spark offers a more user-friendly API and supports multiple languages (Python, R, Scala, Java), making it easier for developers to work with.
- Performance: For iterative algorithms and real-time processing, Spark generally outperforms Hadoop MapReduce due to its in-memory capabilities.
2. Hadoop vs. Apache Flink
- Stream Processing: Flink is designed for real-time stream processing, while Hadoop is primarily batch-oriented. Flink's architecture allows for low-latency processing of streaming data.
- Complex Event Processing: Flink provides built-in support for complex event processing, making it suitable for applications that require real-time analytics.
3. Hadoop vs. Traditional RDBMS
- Data Volume: Traditional relational databases are not designed to handle the same volume of data as Hadoop. Hadoop excels at processing large datasets that would overwhelm an RDBMS.
- Schema Flexibility: Hadoop can handle unstructured and semi-structured data without requiring a predefined schema, while traditional databases require strict schema definitions.
FAQ
1. What programming languages can I use with Hadoop?
Hadoop supports multiple programming languages, including Java, Python, R, and Scala. This flexibility allows developers to choose the language they are most comfortable with or that best fits their project requirements.
2. Is Hadoop suitable for real-time data processing?
While Hadoop is primarily designed for batch processing, it can be integrated with other tools like Apache Spark or Apache Flink to enable real-time data processing capabilities.
3. How does Hadoop ensure data security?
Hadoop provides several security features, including authentication, authorization, and encryption. Organizations can implement Kerberos authentication, use Apache Ranger for fine-grained access control, and enable data encryption at rest and in transit.
4. Can I run Hadoop on cloud platforms?
Yes, Hadoop can be deployed on various cloud platforms, including AWS, Google Cloud, and Microsoft Azure. Many cloud providers offer managed Hadoop services that simplify deployment and management.
5. What is the role of YARN in Hadoop?
YARN (Yet Another Resource Negotiator) is the resource management layer of Hadoop. It manages and schedules resources across the cluster, allowing multiple applications to run concurrently and efficiently utilize the cluster's resources.
In conclusion, Apache Hadoop is a powerful and versatile framework for big data processing. Its scalability, fault tolerance, and flexibility make it a popular choice among organizations looking to harness the power of big data for analysis and decision-making. With a robust ecosystem of tools and a strong community support, Hadoop continues to be a leading solution for managing and processing large datasets.
Ready to try it out?
Go to Apache Hadoop