Amazon SageMaker Ground Truth
Amazon SageMaker Ground Truth enhances machine learning by providing human-in-the-loop capabilities for high-quality data generation and model evaluation.
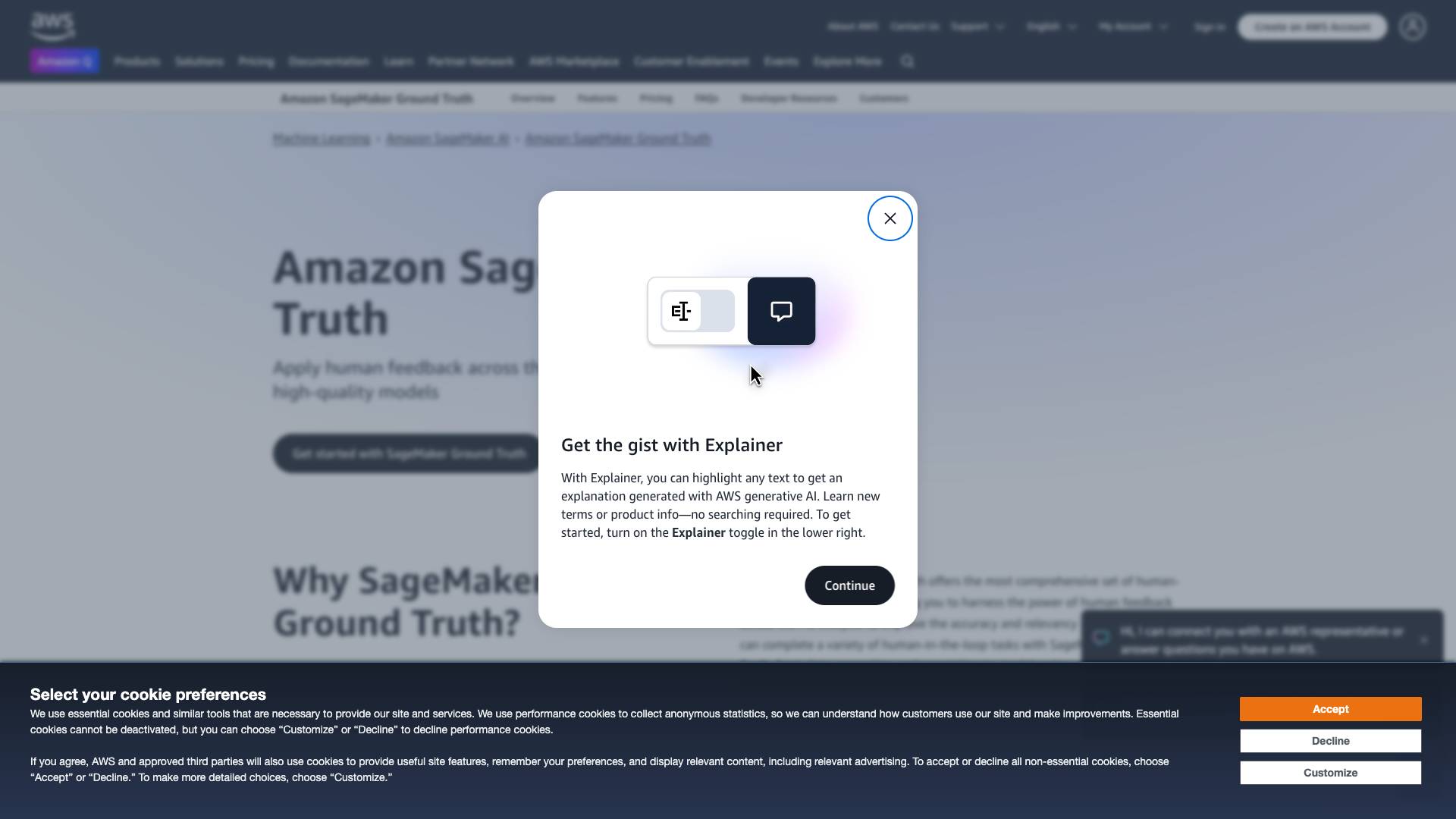
Tags
Useful for
- 1.What is Amazon SageMaker Ground Truth?
- 2.Features
- 2.1.1. Human-in-the-Loop Capabilities
- 2.2.2. Data Generation and Annotation
- 2.3.3. Self-Service and AWS-Managed Options
- 2.4.4. Automated Data Labeling
- 2.5.5. Quality Control
- 2.6.6. Customizable Workflows
- 2.7.7. Integration with Other AWS Services
- 2.8.8. Scalability
- 2.9.9. Multi-Task Support
- 3.Use Cases
- 3.1.1. Natural Language Processing (NLP)
- 3.2.2. Computer Vision
- 3.3.3. Autonomous Vehicles
- 3.4.4. Healthcare
- 3.5.5. Fraud Detection
- 3.6.6. Retail and E-commerce
- 3.7.7. Industrial Automation
- 4.Pricing
- 4.1.1. Data Labeling Costs
- 4.2.2. Human Labeling Costs
- 4.3.3. Free Tier
- 4.4.4. Cost Management
- 5.Comparison with Other Tools
- 5.1.1. Comprehensive Human-in-the-Loop Capabilities
- 5.2.2. AWS Ecosystem Integration
- 5.3.3. Scalability and Flexibility
- 5.4.4. Automated Labeling
- 5.5.5. Customization Options
- 6.FAQ
- 6.1.What types of data can be labeled using Amazon SageMaker Ground Truth?
- 6.2.How does automated data labeling work in Ground Truth?
- 6.3.Can I use my own workforce for labeling tasks?
- 6.4.What quality control mechanisms are available in Ground Truth?
- 6.5.Is there a free trial available for new users?
- 6.6.How do I get started with Amazon SageMaker Ground Truth?
What is Amazon SageMaker Ground Truth?
Amazon SageMaker Ground Truth is a powerful tool provided by AWS (Amazon Web Services) designed to facilitate the creation and evaluation of high-quality machine learning (ML) models through human feedback. It offers a comprehensive set of human-in-the-loop capabilities that enhance the accuracy and relevance of machine learning models by enabling users to generate, annotate, and evaluate datasets efficiently. Ground Truth streamlines the data labeling process, allowing data scientists and ML practitioners to focus on model development rather than the complexities of managing labeling workflows or building custom applications.
Features
Amazon SageMaker Ground Truth is equipped with a variety of features that make it an essential tool for organizations looking to leverage ML effectively. Below are some of the key features:
1. Human-in-the-Loop Capabilities
Ground Truth allows users to incorporate human feedback throughout the ML lifecycle. This capability is critical for improving model accuracy and ensuring that the output aligns with real-world scenarios.
2. Data Generation and Annotation
The tool supports a wide range of human-in-the-loop tasks, including data generation and annotation. Users can create high-quality training datasets without the need to develop custom labeling applications or manage a labeling workforce.
3. Self-Service and AWS-Managed Options
Ground Truth offers both self-service and AWS-managed labeling options. This flexibility allows organizations to choose the level of control they prefer over their labeling workflows.
4. Automated Data Labeling
Ground Truth can automatically label data using active learning techniques. This feature significantly reduces the time and effort required for data preparation, enabling users to focus on model training and evaluation.
5. Quality Control
The platform includes built-in quality control mechanisms to ensure that the labeled data meets high standards. Users can implement various validation techniques to verify the accuracy of labeled datasets.
6. Customizable Workflows
Users can customize their labeling workflows to suit specific project requirements. This flexibility helps organizations adapt the tool to their unique needs and processes.
7. Integration with Other AWS Services
SageMaker Ground Truth integrates seamlessly with other AWS services, providing a comprehensive ecosystem for machine learning development. This integration allows users to leverage the full power of AWS's cloud infrastructure.
8. Scalability
The platform is designed to scale with the needs of organizations. Whether working with small datasets or large-scale projects, Ground Truth can handle varying data volumes without compromising performance.
9. Multi-Task Support
Ground Truth supports various annotation types, including image, text, and video, enabling users to work on diverse machine learning tasks across different domains.
Use Cases
Amazon SageMaker Ground Truth is versatile and can be utilized across various industries and applications. Here are some common use cases:
1. Natural Language Processing (NLP)
Organizations can use Ground Truth to label text data for NLP tasks such as sentiment analysis, entity recognition, and language translation. The human-in-the-loop capabilities ensure that the labeled data reflects nuanced language patterns.
2. Computer Vision
Ground Truth is ideal for computer vision applications, including image classification, object detection, and image segmentation. Users can annotate images to train models that identify and classify visual elements accurately.
3. Autonomous Vehicles
In the automotive industry, Ground Truth can be used to label data collected from sensors and cameras in autonomous vehicles. This data is critical for training models that enable vehicles to navigate safely and effectively.
4. Healthcare
Healthcare organizations can leverage Ground Truth to annotate medical images, electronic health records, and clinical notes. This annotated data can be used to develop models that assist in diagnostics and treatment planning.
5. Fraud Detection
Financial institutions can utilize Ground Truth to label transaction data for fraud detection models. Human feedback helps improve the accuracy of these models, reducing false positives and enhancing security.
6. Retail and E-commerce
Retailers can use Ground Truth to analyze customer reviews and feedback. By labeling sentiment and intent, businesses can gain insights into customer preferences and improve their offerings.
7. Industrial Automation
Ground Truth can assist in automating industrial processes by labeling data related to machinery and operational workflows. This data can then be used to optimize performance and reduce downtime.
Pricing
Amazon SageMaker Ground Truth offers a pricing model that is based on the volume of data processed and the type of labeling performed. Key components of the pricing structure include:
1. Data Labeling Costs
Users are charged based on the number of data items labeled. The cost may vary depending on the complexity of the labeling task (e.g., image annotation may have a different price point compared to text classification).
2. Human Labeling Costs
If users opt for AWS-managed labeling, there will be additional costs associated with the human workforce that performs the labeling tasks. Pricing may vary based on the skill level required for the task.
3. Free Tier
New users can take advantage of the AWS Free Tier, which provides free, hands-on experience with AWS services for 12 months. This allows users to explore SageMaker Ground Truth and its features without incurring costs initially.
4. Cost Management
Organizations can manage costs by selecting self-service options for labeling or automating labeling processes to reduce the need for human intervention. This flexibility helps optimize expenses based on project requirements.
Comparison with Other Tools
When comparing Amazon SageMaker Ground Truth to other data labeling and annotation tools, several unique selling points stand out:
1. Comprehensive Human-in-the-Loop Capabilities
While many tools offer data labeling, few provide the extensive human-in-the-loop capabilities that Ground Truth does. This feature is essential for organizations that require high-quality labeled data for complex ML tasks.
2. AWS Ecosystem Integration
Ground Truth's seamless integration with other AWS services sets it apart from competitors. Organizations already using AWS can leverage their existing infrastructure and tools, reducing friction in the workflow.
3. Scalability and Flexibility
Ground Truth is designed to handle projects of varying sizes and complexities. Its ability to scale with organizational needs makes it suitable for both small startups and large enterprises.
4. Automated Labeling
The automated labeling feature powered by active learning is a significant advantage. This capability reduces the time and effort required for data preparation, allowing users to focus on model development.
5. Customization Options
Ground Truth allows users to customize their labeling workflows, providing the flexibility needed to adapt to specific project requirements. This level of customization is often lacking in other tools.
FAQ
What types of data can be labeled using Amazon SageMaker Ground Truth?
Amazon SageMaker Ground Truth supports a variety of data types, including images, text, and video. This versatility allows organizations to work on diverse machine learning tasks across different domains.
How does automated data labeling work in Ground Truth?
Automated data labeling in Ground Truth utilizes active learning techniques. The system selects the most informative data points for labeling, allowing human labelers to focus on the data that will most improve model performance.
Can I use my own workforce for labeling tasks?
Yes, Ground Truth allows users to manage their own labeling workforce through self-service options. Organizations can choose to use their own labelers or leverage AWS-managed labeling services.
What quality control mechanisms are available in Ground Truth?
Ground Truth includes built-in quality control features that enable users to validate labeled data. This may include reviewing a sample of labeled data, implementing consensus labeling among multiple labelers, and applying validation rules.
Is there a free trial available for new users?
Yes, new users can benefit from the AWS Free Tier, which offers free, hands-on experience with AWS services, including SageMaker Ground Truth, for 12 months.
How do I get started with Amazon SageMaker Ground Truth?
To get started with Ground Truth, users can follow tutorials available on the AWS platform that guide them through setting up labeling workflows and utilizing the tool's features effectively.
In conclusion, Amazon SageMaker Ground Truth is a robust and flexible tool that enhances the machine learning lifecycle by integrating human feedback into the data labeling process. With its comprehensive features, diverse use cases, and seamless integration with the AWS ecosystem, it stands out as a leading solution for organizations looking to develop high-quality ML models efficiently.
Ready to try it out?
Go to Amazon SageMaker Ground Truth